Google AlphaGo Zero Trains Self to Beat the ‘God of Go’
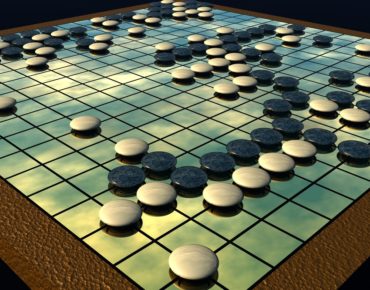
Source: Shutterstock
Imagine if all the atoms in the universe could be added up to a single number. Big number, right? Maybe the biggest number conceivable. But wait, there’s a bigger number out there. We're told that Go, the world’s oldest board game, has more possible board positions than there are atoms in the universe. Urban myth? All right, let’s say Go has half as many positions as there are atoms. Make it a tenth. The point is: Go complexity is beyond measure.
DeepMind, Google’s AI research organization, announced today in a blog that AlphaGo Zero, the latest evolution of AlphaGo (the first computer program to defeat a Go world champion) trained itself within three days to play Go at a superhuman level (i.e., better than any human) – and to beat the old version of AlphaGo – without leveraging human expertise, data or training.
The absence of human training may have "liberated" AlphaGo Zero to find new ways to play Go that humans don't know, putting the new system beyond the talents of the human-trained AlphaGo (which only last May was called by world no. 1 player Ke Jie "the god of Go").
Richard Windsor, analyst at Edison Investment Research, London, notes that today’s announcement is an important step forward on one of the three big AI challenges which, he said, are:
- AI systems that can be trained with less data
- AI that takes lessons learned from one task and applies it across multiple tasks
- AI that builds its own models
“DeepMind has been able to build a new Go (AlphaGo Zero) algorithm that relies solely on self-play to improve and within 36 hours was able to defeat AlphaGo Lee (the one that beat [professional Go player] Lee Sedol) 100 games to 0…,” Windsor said. “DeepMind’s achievement represents a huge step forward in addressing the first challenge as AlphaGo Zero used no data at all…”
According to DeepMind, previous versions of AlphaGo were trained on the basis of thousands of human games. But AlphaGo Zero “skips this step and learns to play simply by playing games against itself, starting from completely random play.” In doing so, it quickly surpassed human level of play and went undefeated against AlphaGo.
The new self-training algorithm, according to the DeepMind blog, is significant for AI systems taking on problems for which “human knowledge may be too expensive, too unreliable or simply unavailable. As a result, a long-standing ambition of AI research is to bypass this step, creating algorithms that achieve superhuman performance in the most challenging domains with no human input.”
DeepMind said AlphaGo Zero uses a novel form of reinforcement learning in which the system starts off with a neural network that knows nothing about Go. “It then plays games against itself, by combining this neural network with a powerful search algorithm. As it plays, the neural network is tuned and updated to predict moves, as well as the eventual winner of the games.”
The updated neural network is then recombined with the search algorithm to create a new, stronger version of AlphaGo Zero, and the process begins again, improving incrementally with each game.
“This technique is more powerful than previous versions of AlphaGo because it is no longer constrained by the limits of human knowledge. Instead, it is able to learn tabula rasa from the strongest player in the world: AlphaGo itself.”
Put another way by Windsor: “It is almost as if the use of human data limited the potential of the machine’s ability to maximize its potential.”
While the new system makes strides against the self-training Big AI Challenge, Windsor expressed doubts that it addresses the third challenge (automated model building) because it used a model already used by the previous version of AlphaGo.
“…the system of board assessment and move prediction (but not the experience) used in AlphaGo Lee was also built into AlphaGo Zero,” said Windsor. “Hence, we think that this system was instead using a framework that had already been developed to play and applying reinforcement learning to improve, rather than building its own models.”
But this isn’t to minimize the achievement of AlphaGo Zero, nor to quell those (such as Elon Musk) who worry that human intelligence will eventually be dwarfed by AI, with potential dystopic implications.
“What will really have the likes of Elon Musk quaking in their boots is the fact that AlphaGo Zero was able to obtain a level of expertise of Go that has never been achieved by a human mind,” Windsor said.
Having said that, include Windsor among those who don’t believe machines will enslave the human race. He also said that DeepMind may have trouble applying its achievement elsewhere.
“Many of the other digital ecosystems have been trying to use computer generated images to train image and video recognition algorithms but there has been no real success to date and we suspect that taking what DeepMind has achieved and applying it to real world AI problems like image and video recognition will be very difficult,” he said, explaining that “the Go problem is based on highly structured data in a clearly defined environment whereas images, video, text, speech and so on are completely unstructured.”
But DeepMind sounded a more optimistic note on the broader applicability of AlphaGo Zero teaching itself new and incredibly complicated tricks.
“These moments of creativity give us confidence that AI will be a multiplier for human ingenuity, helping us with our mission to solve some of the most important challenges humanity is facing…. If similar techniques can be applied to other structured problems, such as protein folding, reducing energy consumption or searching for revolutionary new materials, the resulting breakthroughs have the potential to positively impact society.”