AI Cloud Competition Heats Up: Google’s Cloud TPUs, Amazon’s AI Chip
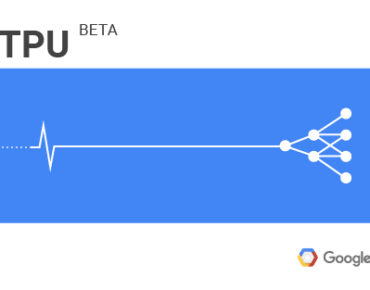
Competition in the white hot AI (and public cloud) market pits Google against Amazon this week, with Google offering AI hardware on its cloud platform intended to make it easier, faster and cheaper to train and run machine learning/deep learning systems, while Amazon is reportedly developing its own AI chip portfolio. It’s the latest in a series of processor-related moves by the two companies, along with Microsoft Azure, IBM Cloud and other public cloud services providers, have made in recent months to position themselves as AI becomes increasingly integrated into our business and home lives.
Google is making Cloud TPU (Tensor Processing Units) accelerators available starting today on the Google Cloud Platform (GCP), an offering the company said will help get machine learning (ML) models trained and running more quickly.
Cloud TPUs is Google-designed hardware designed to speed and scale up ML workloads programmed with TensorFlow. Built with four custom ASICs, each Cloud TPU has up to 180 teraflops of floating-point performance and 64 GB of memory on a single board.
“Instead of waiting for a job to schedule on a shared compute cluster, you can have interactive, exclusive access to a network-attached Cloud TPU via a Google Compute Engine VM that you control and can customize,” said John Barrus, product manager for Cloud TPUs, Google Cloud, and Zak Stone, product manager for TensorFlow and Cloud TPUs, Google Brain Team, in a jointly written blog post. “Rather than waiting days or weeks to train a business-critical ML model, you can train several variants of the same model overnight on a fleet of Cloud TPUs and deploy the most accurate trained model in production the next day.”
Meanwhile, Reuters reports that Amazon two months ago paid $90 million for home security camera maker Blink and its energy efficient chip technology, according to unnamed sources.
“The deal’s rationale and price tag, previously unreported, underscore how Amazon aims to do more than sell another popular camera, as analysts had thought,” Reuters reported. “The online retailer is exploring chips exclusive to Blink that could lower production costs and lengthen the battery life of other gadgets, starting with Amazon’s Cloud Cam and potentially extending to its family of Echo speakers, one of the people said.”
According to the report, Amazon seeks to strengthen its ties to consumers via in-house devices. And while Amazon’s Cloud Cam and Echo need a plug-in power source, Blink claims its cameras can last two years on two AA lithium batteries.
Amazon declined to comment on the acquisition’s terms or strategy.
In addition, a published report from The Information states that Amazon is developing its own AI chip designed to work on the Echo and other hardware powered by Amazon’s Alexa virtual assistant. The chip reportedly will help its voice-enabled products handle tasks more efficiently by enabling processing to take place locally at the edge, by the device, rather than in AWS.
Sister publication HPCwire reported last October that the surging demand for HPC and AI compute power has been shrinking the time gap between the introduction of high-end GPUs, primarily developed by Nvidia and adoption by cloud vendors. “With the Nvidia V100 launch ink still drying and other big cloud vendors still working on Pascal generation rollouts, Amazon Web Services has become the first cloud giant to offer the Tesla Volta GPUs, beating out competitors Google and Microsoft,” HPCwire reported. “Google had been the first of the big three to offer P100 GPUs, but now we learn that Amazon is skipping Pascal entirely and going directly to Volta with the launch of V100-backed P3 instances that include up to eight GPUs connected by NVLink.”
As for Google’s Cloud TPUs, the company said it is simplifying ML training by providing high-level TensorFlow APIs, along with open-sourced reference Cloud TPU model implementations. Using a single Cloud TPU, the authors said ResNet-50 (and other popular models for image classification) “to the expected accuracy on the ImageNet benchmark challenge in less than a day” for less than $200.
Barrus and Stone also said customers will be able to use Cloud TPUs either alone or connected via “an ultra-fast, dedicated network to form multi-petaflop ML supercomputers that we call ‘TPU pods.’”Customers who start now with Cloud TPUs, they said, will benefit from time-to-accuracy improvements wne TPU pods are introduced later this year. “As we announced at NIPS 2017, both ResNet-50 and Transformer training times drop from the better part of a day to under 30 minutes on a full TPU pod, no code changes required.”
"We made a decision to focus our deep learning research on the cloud for many reasons,” said Alfred Spector, CTO at investment management firm Two Sigma, “but mostly to gain access to the latest machine learning infrastructure. Google Cloud TPUs are an example of innovative, rapidly evolving technology to support deep learning, and we found that moving TensorFlow workloads to TPUs has boosted our productivity by greatly reducing both the complexity of programming new models and the time required to train them. Using Cloud TPUs instead of clusters of other accelerators has allowed us to focus on building our models without being distracted by the need to manage the complexity of cluster communication patterns."
On-demand transportation company Lyft also said it’s impressed with the speed of Google Cloud TPUs. “What could normally take days can now take hours,” said Anantha Kancherla, head of software, self-driving Level 5, Lyft. “Deep learning is fast becoming the backbone of the software running self-driving cars. The results get better with more data, and there are major breakthroughs coming in algorithms every week. In this world, Cloud TPUs help us move quickly by incorporating the latest navigation-related data from our fleet of vehicles and the latest algorithmic advances from the research community.”
Barras and Stone highlighted in Cloud TPUs the usual advantages offered by public cloud computing. “Instead of committing the capital, time and expertise required to design, install and maintain an on-site ML computing cluster with specialized power, cooling, networking and storage requirements,” they said, “you can benefit from large-scale, tightly-integrated ML infrastructure that has been heavily optimized at Google over many years.”
Google said Cloud TPUs are available in limited quantities today and usage is billed by the second at the rate of $6.50 USD / Cloud TPU / hour.