IBM Builds AI Framework Around Power9
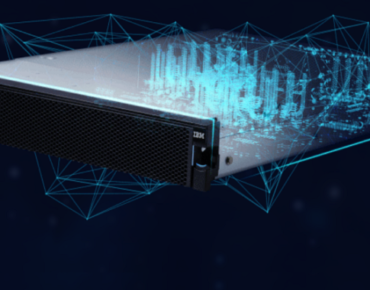
Moving artificial intelligence initiatives from the proof-of-concept stage to production and enterprise adoption remains tough sledding as projects often hit data management and infrastructure performance roadblocks. Those performance issues include a lack of production-grade software for advanced scale, analytics and big data components of enterprise AI initiatives.
Cloud and other IT infrastructure vendors are attempting to fill the AI development gap with software tools, reference architectures and alternatives to traditional on-chip processing. All are designed to accelerate the transition from experimentation and model training to production and enterprise-wide adoption of AI applications.
Some, like IBM, are looking to combine new these AI development frameworks with customized hardware such as servers based on its Power9 processors.
IBM (NYSE: IBM) is touting a new on-premises AI infrastructure reference architecture, a set of tools based on cognitive algorithms and automations intended to hasten the transition of open source AI frameworks to production. The platform runs on GPU-accelerated Power System servers customized for AI development along scalable storage that “not only cost-effectively handles the volume of data needed for AI, but also delivers the performance needed to keep data-hungry GPUs busy all of the time,” the company explained in a recent blog post.
IBM said last fall that Power9 would be the first commercial platform providing on-chip support for Nvidia’s next-generation NVLink, OpenCAPI 3.0 and PCI-Express 4.0. “These technologies provide a giant hose to transfer data,” the company said. The chip architecture also seeks to move away from single-chip processing, allowing AI developers to “mix and match” GPU and FPGA accelerators for AI development.
IBM’s PowerAI Enterprise architecture also is being positioned as a way to fill the current “knowledge gap” as AI and deep learning make inroads in the enterprise, taxing the resources of data science operations. The new tools aim to “increase the productivity of data scientists throughout the AI workflow,” IBM said.
Among the early deployments based on IBM’s AI development platform are deep learning models used to comply with banking regulations. Another bank used the platform to develop a sort of AI academy for its data scientists aimed at improving their ability to identify fraud and minimize risk. The result was a distributed deep learning service that allowed data scientists to share infrastructure resources, IBM said.
Along with supplying tools intended to streamline the AI model development workflow, the company said improved models can be scaled as requirements grow and the resulting data science infrastructure can be shared across development teams.
The reference architecture includes a “developer portal” for deep learning projects running on IBM Power systems along with a PowerAI cluster deployment kit.
The AI service is promoted as supporting data ingest and preparation, model training and optimization, and from testing to inference. With an eye on filling current AI software gaps, the architecture also includes IBM distributions of open source deep learning frameworks such as TensorFlow and IBM’s version of the Caffe deep learning framework.
The AI reference architecture represents both an attempt to fill the current software development gap and another step by IBM to push its trove of propriety AI and Watson cognitive technologies along with its Power server platform deeper into enterprises. Its Power9 server is tailored to AI and data-intensive workloads, noted Steven Fiorillo in a IBM market analysis posted on the web site SeekingAlpha.com.
“Every organization will recognize the need for an AI interface which can understand their data,” Fiorillo wrote.
--Managing Editor Doug Black contributed to this report.
Related
George Leopold has written about science and technology for more than 30 years, focusing on electronics and aerospace technology. He previously served as executive editor of Electronic Engineering Times. Leopold is the author of "Calculated Risk: The Supersonic Life and Times of Gus Grissom" (Purdue University Press, 2016).