A ‘Deliberate Data Strategy’ for Today’s Attention-Deficit Economy
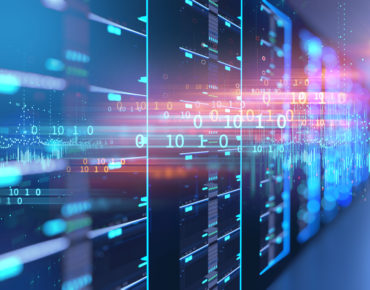
In our attention-deficit economy, organizations able to grab our attention end up winning. Companies that stand out are those that embrace an insight-driven ethos and build data-competent organizations. They do this by adopting a "deliberate data strategy" that follows these key principles:
- Love the problem, not the solution
- Inject intelligence into operations
What Is the Attention Economy?
In the early 1970s, Nobel Prize winning economist Herbert Alexander Simon argued we were starving in an information-rich world. Simon believed the wealth of one resource meant the dearth of whatever that resource consumed. Because information consumes our attention, the abundance of it creates an attention deficit. Fast forward 50 years, data continues to explode in abundance. We are inundated with advice, ideas, technological breakthroughs.
However, competitive advantage stems not from what is abundant but from what is scarce. Though data is everywhere, the real-time extraction and deployment of insight is limited.
The Crisis of Complexity
While data may be the most valuable asset, the overabundance of data sources has created a crisis of complexity. As the proliferation of information places more demands on the finite attention of consumers, enterprises need to efficiently collect, analyze and allocate data sources.
The crisis of complexity began when we started collecting data in separate silos, resulting in towers of disconnected data. Any IT manager will tell you, it’s not the tools that allow you to save data that are hard, it’s drawing the lines between them.
Data in separate buckets leads to the loss of information and causes misinformed decisions. And, perhaps worse, because we have a limited amount of attention, we tend to solve for the simple and obvious and don’t solve for harder problems, which are more important and beneficial to our business.
With machine learning, this problem only gets worse because now we are creating yet another silo for AI/ML – only this one is taller and bigger.
Data Logistics to the Rescue
Not only has the abundance of machine learning algorithms led to a deficit of data scientists, it has also created a deficit in the data logistics space. So how do you remedy that deficit?
Data logistics is the process by which all data, regardless of location or format, is provided to both data scientists and machine learning algorithms in a secure and efficient way. To resolve the deficits in data logistics, a deliberate data strategy is needed that follows three principles:
Love the Problem, Not the Solution
It’s hard not to focus first and foremost on tools. But don't get let your attention get consumed by the technology. There are simply too many tools out there, and bigger and better ones will soon replace them anyway.
Instead, become enamored with the problem. Take the time to dig in and understand what your organization needs. Once you’ve identified and understand your issues, you will be able to find a solution and choose your tools more wisely.
The main problem here is that point products lead to a crisis of complexity. Imagine for your AI or analytics project you have Solution Vendor 1, Tool Vendor 2, Solution Vendor 3 and Cloud Vendor 4. How do you launch, manage and support across that spectrum of point products? Let’s get a bit more granular: imagine a fraud detection use case. Vendor 1 is a data lake vendor, vendor 2 a BI vendor, vendor 3 a governance product. As for the cloud vendor, honestly, it doesn’t matter – cloud vendors are not interested in interoperability today. And guess what? They won’t be interested in it in 2019 either.
How do you manage all those point products to get to your fraud detection application? Let your business problem guide you on what needs to be done, and don’t get distracted by those shiny toys masquerading as point solutions.
Inject Intelligence into Operations
Enterprises need modern, mission-critical data flows and real-time applications as business happens. You need to mine real-time data for insight, and then leverage that insight back into operations before its advantage vanishes.
A deliberate data strategy also must combine real-time ops and historical data in one platform for queries, analytics, reporting, AI and more. Though using test data for AI/ML is great, it’s simply not enough anymore.
In order to win in our attention-deficit economy, you need to understand the concept of dataware. Dataware is an approach that works across broad use cases and optimizes the entire data lifecycle, from ingestion to processing. It supports applications that require real-time analytics and AI/ML. It also lets you handle data on-premises, across multiple clouds, at the edge and in container infrastructures.
Dataware is a new layer in the enterprise software stack. It solves the challenges of today’s data environment by letting you manage the entire data ecosystem, including multiple clouds and containerized infrastructure. You no longer need to manage multiple data technologies to support distributed applications and analytics across locations. Dataware is powerful for three reasons:
- The abstraction layer provides a consistent approach for enterprises to manage, secure, govern and protect data
- A set of standard APIs enable the consumption of data by a broad set of applications and tools
- The complexity of data is handled across locations, hardware infrastructures from on-premises to the cloud to the edge, and containers
Dataware optimizes the entire data lifecycle – from ingestion to processing – to enable applications that simultaneously require real-time analytics, machine learning, and AI. Dataware gives complete flexibility in leveraging the underlying infrastructure (on-premises, cloud, or containerized infra- structure) and deployment patterns (hybrid or multi-cloud).
Because dataware unshackles data from the layers of software, middleware and hardware, your enterprise has end-to-end control over data security, placement, access, tenancy and controls. This allows you to focus on leveraging data instead of being distracted by the management of multiple technologies required to support distributed applications and analytics across locations.
A Wealth of Deliberate Data
These strategies will help organizations deal with the deficits created by the abundance of data in our information-rich world. Creating a deliberate data strategy is the only way to win in our attention-deficit economy.
William Peterson is VP industry solutions for MapR.