How to Avoid the Downside of a Cloud-First Strategy
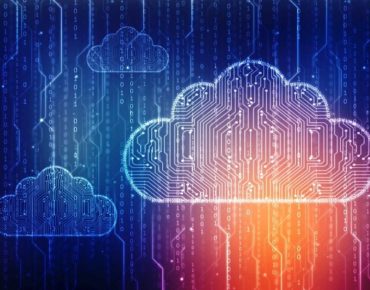
Cloud computing has evolved to the point where most enterprises have, to one degree or another, deployed cloud workloads. Our 2018 Cloud Survey found 70 percent of over 900 IT professionals use cloud services. But as enterprises see the benefits – namely business continuity, IT efficiency, elasticity and the pay-as-you-go billing model – business challenges remain.
According to the survey, only 29 percent of IT professionals said their organizations have a centralized strategy for moving applications to the cloud, while 42 percent reported migrations were typically conducted on an ad hoc basis. This piecemeal approach, which may benefit companies beginning to delve into cloud technology, often means organizations struggle to contain cloud costs, especially those using it beyond initial adoption or experimentation. In fact, 53 percent said cloud costs were higher than expected and 62 percent called out data and application migration costs as a particular challenge.
Is the high cost of cloud storage related to this ad hoc approach to application management?
In 2017, while some of our customers operating in the cloud had specific initiatives around ecommerce or healthcare, last year saw a big shift as large financial services, banking and insurance companies either created new workloads in the cloud or created internal policies mandating new workloads to be cloud deployment-ready.
Some organizations refer to this as “cloud-native” or “cloud-first,” that is, requiring new application design to take advantage of cloud elasticity. The growing volume and variety of data being migrated to in the cloud is pushing IT to reevaluate cloud management as they face lightspeed changes in areas like data science and governance.
It’s clear cloud adoption is increasing, but many organizations lack a formal strategy, and these “lift and shift” cloud migrations may be hurting them. Keep in mind that most applications weren’t architected to take advantage of cloud elasticity, they were created to run on a particular hardware, storage or capacity. As such, companies may be running at the maximum capacity of what they require, which becomes expensive for both storage and computing purposes. Leveraging cloud elasticity requires rearchitecting, and without a cloud-first strategy, enterprises run the risk of running up costs of migrating workloads.
So what’s to be done?
Technology stacks and cloud deployment will continue to rapidly evolve, requiring organizations to create cloud-first applications. It will also be necessary to build skill sets to handle diverse platforms and deployment models. As part of an overarching strategy, selecting cloud-agnostic tools, or working with partners offering future-proofing tools for vendor-based implementations, ensure applications run with no changes, whether on-premise, in hybrid cloud or in the public cloud. For these instances, tool selection becomes even more important to find partners and software, such as data integration and data quality tools, that are interoperable among all cloud vendors.
Another consideration is to adopt a multi-cloud approach. Many companies are running pilots and production with all three major cloud vendors to optimize processes. This way, they’re finding world-class tools to address specific needs, helping them remain as cloud agnostic as possible to avoid vendor lock-in. Interoperability of tools and technology stack is critical. Optimizing cloud infrastructure for cost and efficiency means looking at the requirements for storage, network data load in and out of cloud, processing requirements and selecting the cloud vendor and cloud instances accordingly.
Enterprise adoption of hybrid cloud is increasing. Organizations considering this model should look for partners with multiple deployment models, both on-premise or in the cloud – especially for data integration, data quality and capacity management requirements.
Many organizations start their new projects by analyzing the increasing volume of data generated in the cloud. When running production deployments, the enterprise often needs to integrate cloud-originated data sets with data assets in on-premise data stores. Utilizing machine learning and data science tools offered by cloud vendors can help implement proof-of-concepts faster and reduce time-to-market for new solutions. All three major public cloud vendors offer a rich set of tools for ML and AI, which simplifies data science without requiring separate IT infrastructure for these emerging technologies.
As cloud adoption continues and the market becomes more established, certain aspects will continue to evolve. To maximize benefits from cloud, IT organizations must develop a cloud strategy while continuing to strengthen security and privacy in the cloud – a previous roadblock for many organizations – for public, private or hybrid cloud.
Tendu Yogurtcu, PhD., is chief technology officer at Syncsort.