How to Overcome the AI Trust Gap: A Strategy for Business Leaders
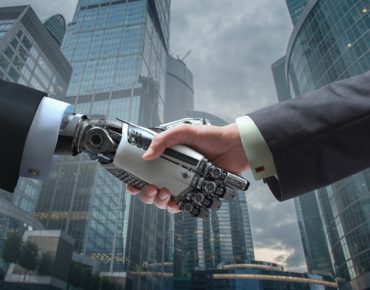
AI is like the world, you can’t get around it – you’ve got to take it on. Numerous studies point to higher profits and productivity at companies that adopt machine and deep learning, and most companies have AI projects underway. Business leaders frozen in AI FUD are on borrowed time: eventually, they’ll be at a major competitive disadvantage. But enterprise AI at-scale is mainly confined to the top 5 percent of hyperscalers and resource-rich companies in the major business verticals. For the bottom 95 percent, breaking AI out of the pilot project level is a critical business imperative that is happening too slowly.
What’s holding back the 95 percent? Everything about AI is hard for business managers to get their hands around: how it works, how it makes decisions, whether the data it’s using is valid, whether its decisions can be trusted and free of bias. Companies want to move beyond pinpricks of AI, but AI is like a castle surrounded by a moat of complexity barring all but project-level raiding parties.
Too often of no help making AI less opaque are data scientists, the AI elite who talk with alarming fluency about such things as bias variance decomposition, expected generalization errors of algorithms, achieving higher model accuracy without overfitting, gradient boosting and random forests tree ensemble learning algorithms, longitudinal and latitudinal model value rounding, geolocation data scoring and how model hyperparameters affect the decomposition of expected prediction errors for regression problems.**
What was the second thing?
It’s the impenetrability of the AI black box and the disconnect between business managers and AI technical specialists that blocks broader AI adoption, according to a report recently released by KPMG. But there are ways of getting AI unstuck. KPMG is telling clients: Stop ducking AI and take charge of it.
The consulting company has developed an AI oversight model that it says is symptomatic of a new chapter for AI, “signal(ing) the end of self-regulation” and broadening it beyond the domain of data scientists in the lab. At the heart of KPMG’s approach: business leaders should embrace AI, weave it into the mainstream of the organization’s business and management culture, don’t treat data scientists as members of a separate sect – assimilate them.
In “Controlling AI: The Imperative for Transparency and Explainability,” the consulting firm said:
“Any organization that builds or adopts advanced, continuous-learning technologies is tapping into a power for insight and decision-making that far exceeds the capabilities of the human mind. This is a massive opportunity. But algorithms can be destructive when they produce inaccurate or biased results, an inherent concern amplified by the black box facing any leader who wants to be confident about their use. That is why, in the midst of enormous excitement around AI, there is hesitancy in handing over decisions to machines without being confident in how decisions are made and whether they’re fair and accurate. This is a trust gap.”
Bridging the gap and realizing the potential of AI, according to KPMG, will happen “only when algorithms become explainable (and, hence, understandable) in simple language, to anyone. The trust gap exists because there is no transparency of AI; instead, there is an inherent fear of the unknown surrounding this technology. Gaining trust also involves understanding the lineage of the AI models and protecting them (and data that forms them) from different types of adversarial attacks and unauthorized use. Critical business decisions made by AI affect the brand—and consumer trust in the brand — and they can have an enormous impact on the well-being or safety of consumers and citizens. No one wants to say, ‘because the machine said so.’ No one wants to get AI wrong.”
Getting it right means overcoming a classic, early-stage struggle with any emerging technology that has the power to impact business and society – the struggle by non-specialists to understand, control and manage it. Part of this will be addressed by AI democratization, the development of more user-friendly AI tools that can be used by a broader market. But it also requires a new strategy on the part of business managers.
We talked with report co-author Martin Sokalski, KPMG principal, advisory, Emerging Technology Risk Services, who said, “We wrote (it) mainly because we’ve noticed that the market was struggling a little bit with adopting AI at scale, so we came up with this hypothesis that if we can help a client think about driving greater trust, transparency, explainability and bias detection into their AI programs, maybe that will help them scale AI more within their organizations.”
Overcoming the trust gap is a top goal of business leaders, 45 percent of whom surveyed by Forrester Research last year said that trusting AI systems was challenging or very challenging. Sokalski said most leaders don’t have a clear idea of how to approach AI governance, and 70 percent of those surveyed in a KPMG study said they don’t know how to govern algorithms.
“Companies are struggling to decide who is accountable for AI programs and results,” stated the KPMG report. “During our interviews, we heard that most companies are still trying to determine who has authority over AI deployment. Some companies have established a central authority in an AI council or Center of Excellence; others have assigned responsibility to different leaders, like the Chief Technology Officer or Chief Information Officer.”
Sokalski said KPMG recommends a strategy that incorporates tech-based methods that address the inherent risks and ethical issues in AI directed at four “trust anchors”: integrity, explainability, fairness, and resilience.
Further, Sokalski said it’s important to adopt a lifecycle approach, “to monitor for those things on an ongoing basis. The inherent nature of machine learning is that it continues to learn, so you don’t put a model algorithm out into the wild and … it will remain static for years to come. With AI, it continues to learn and evolve as you introduce new data sets to it…, we’ve seen cases where that has gone wrong, and you might have had an unbiased model at design but then over time bias was introduced. So it’s not just the build, not just the deployment, it’s the continual evolution of the model that changes, so continual monitoring is needed to see if your four pillars of trust maintain their existence.”
In the end, Sokalski said, it’s about integrating the work of data scientists within an AI oversight model in a way that’s suffused with open, ongoing communications between AI technicians and business managers with the goal of enabling non-specialists to understand, and be able to explain, how the machine learning system works.
“It’s not just the data scientists cooking up these incredible solutions in a vacuum,” he said, “it is the data scientists that historically sat in the lab, developing mathematical models, are now sitting in business meetings to gain a better understanding of what the business outcomes are hoping to achieve, as well as how do we accomplish that in a way that meets our business objectives, as opposed to some AI-specific problem.”
The four anchors of trust underpinning KPMG oversight model include:
Algorithm integrity: “What leaders need to know is this: the provenance and lineage of training data, controls over model training, build, model evaluation metrics and maintenance from start to finish, and the verification that no changes compromise the original goal or intent of the algorithm.”
Explainability: “Understanding the reasons a model made a prediction — and being able to interpret the reasons— is essential in trusting the system, especially if one has to take an action based on those probabilistic results.” The report cites explainability approaches, including LIME (local interpretable model-agnostic explanations), the Defense Advanced Research Projects Agency (DARPA) Explainable AI (XAI) program.
Resilience: This relates to the robustness and resilience of deployed models or algorithms, which “are typically exposed as APIs or embedded within applications, and they need to be portable and operate across diverse and complex ecosystems. Resilient AI should cover all the aspects of secure adoption and holistically address risks through securely designed architecture... The goal is to help ensure all the components are adequately protected and monitored."
Fairness: ethics and accountability: For algorithms to be fair, they need to be built free from bias and maintain fairness throughout their lifecycles. “In some instances…, personal information is relevant to the model, as in healthcare when gender or race can be a critical part of studies or treatment. Careful oversight and governance is needed to make sure proxy data doesn’t train a model. A postal code, for example, can be a proxy for ethnicity or income and inadvertently produce biased results and downstream risks — just one being regulatory violations. Techniques must be applied to understand bias that inherently exist in the data, and mitigate them using approaches such as rebalancing, reweighting, or adversarial debiasing.”
As for governance, KPMG said organizations must ask the question: “Who among the humans is accountable for the results of AI? Accountability is a crucial governance issue that must be established across all AI initiatives, down to each individual model.”
KPMG said too few organizations “have solid accountability practices in place, a leadership gap that can weaken trust internally and among external stakeholders. A big reason for this missing link: Most organizations lack tools and expertise to gain a full understanding and introduce transparency into their algorithms."
The key, Sokalski said, is to “make sure the we don’t let the technology get ahead of human capabilities… It’s integrating the framework, the tooling, the methods, the processes from strategy through execution through evolution that will help everyone gain more confidence in AI so that the decisions it makes, they can stand behind them confidently.”
** See recent Nvidia blogs “Bias Variance Decompositions using XGBoost” and “When Less is More: A brief story about feature engineering with XGBoost.”