AI Implementation Challenges: A Primer
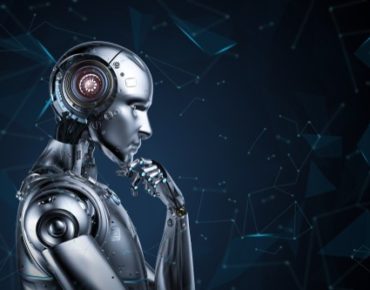
AI can help organizations save time and money, make automated processes smarter and more efficient, it can go through mountains of data faster than the human mind and provide real-time insights for better decision-making and it can respond on behalf of human administrators, performing tasks such as fixing bugs and blocking bot attacks.
AI is the product of complex technological processes. It will provide different results in different fields and perform differently based on the underlying infrastructure. This complexity often prevents organizations from locating and implementing the right AI solutions for their needs.
Let’s look at some key implementation challenges in large organizations and review potential solutions.
Lack of Familiarity with AI Technology
AI’s a technology that requires careful handling using science-based skills. Companies that rush to adopt or create an ad-hoc AI solution without proper planning and administration are likely to be disappointed. The goal is to give machines the ability to autonomously perform tasks, such as planning, learning and problem-solving. There are various degrees of autonomy, but to be considered AI, the machine needs to be able to perform tasks without being instructed by a program.
The most common use case of AI is automation, which enables machines to perform tasks with little to no human intervention. There are 5 levels of automation, which define the amount of human intervention involved, if at all. Automation can be enabled through a program that tells the machine how to perform tasks, or through AI, which enables script-less behavior. With AI, automation becomes an intelligent process that follows independent thinking rather than a program.
To enable autonomous thinking, data scientists design machine learning (ML) models that identify, understand and analyze patterns. Just like humans, ML processes become more proficient given time and experience. The more data the ML goes through, the more expertise it acquires. Building a DL process requires the precision of a brain surgeon and often involves expensive hardware. Data scientists use modeling environments and deep learning platforms to build, test and run their AI, ML and DL algorithms.
Before embarking on an AI journey, organizations should get familiar with the technology. Most everyone wants AI, and the increasing demand for AI talent, AI consultants and AI resources has created a steep price for these services.
Lack of Familiarity With AI Vendors
According to Crunchbase, the AI marketplace is at an all-time record, with a global capacity of nearly 10,000 AI-dedicated organizations. With so much data, choosing an AI vendor could be a task for AI rather than a human. To help organizations make sense of this, in 2018 the AI research company Cognilytica introduced a simple classification system of the AI vendor ecosystem. Cognilytica separates the AI vendor marketplace into four major layers, placed according to hierarchy levels.
Each layer/level represents a different AI technology, as follows:
- Industry-Specific Applications of AI—this is the highest level and the end result of the AI operation. Vendors of this level apply a variety of AI technologies and provide their customers with a finished product. For example, intelligent cars, autonomous farming and financial fraud detection.
- Horizontal Applications of AI—these are AI-powered solutions that solve specific problems, such as intelligent drones, chatbots and intelligent data analysis, but the vendor solutions don’t have to be tied to an industry.
- AI Enabling Technology—this level is comprised of solutions that support the infrastructure of AI products, they help organizations build and maintain AI products, such as adaptive robotic hardware, computer vision suites and cloud ML platforms.
- AI and ML Infrastructure—this level is made up of the building blocks of AI and ML infrastructures, offering components and services – such as edge devices, data labeling and data science platforms – that aren’t necessarily dedicated to AI but are required for the creation and maintenance of the AI technology.
Before implementing an AI solution, take stock of the needs of your organization. Once you have the specs and research, you’ll be able to choose the vendors that meet the exact needs of your organization. To avoid unnecessary overhead, choose solutions that can be integrated with your existing infrastructure. Every piece of technology that enters your ecosystem should be able and ready for optimal communication with the network and underlying systems.
Gilad David Maayan is a technology writer who heads Agile SEO, a technology marketing agency.