MIT Says Its Forecasting Model Outperforms Wall Street Benchmark
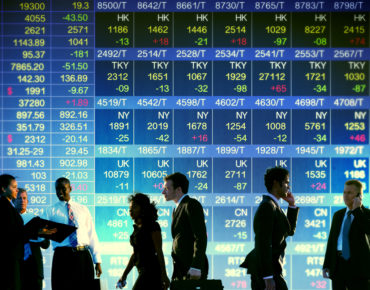
Accurately predicting a business's performance is a prized skill among investors, competitors and other market analysts. Now, MIT is highlighting a new model that challenges the typical blend of punditry, quarterly balance sheet numbers and data-crunching by bringing in new sources of data and by automating the process. The result: it outperforms Wall Street analysts, according to MIT.
The model makes use of “alternative data” – such as credit card purchase data, smartphone location data, satellite imagery and so on – generated more frequently than more direct financial data, such as stock prices and announced earnings. The researchers set out to effectively combine alternative data with traditional financial data, a feat that had proved difficult until now.
Michael Fleder, an MIT postdoctoral researcher and first author of "Forecasting with Alternative Data," said, “Alternative data are these weird, proxy signals to help track the underlying financials of a company. We asked, ‘Can you combine these noisy signals with quarterly numbers to estimate the true financials of a company at high frequencies?’ Turns out the answer is yes.”
The researchers leveraged consumer credit card transactions and quarterly financial reports for 34 retailers across three years, working to use the alternative data as a proxy for gradual changes in sales numbers. “We have a ‘small data’ problem,” Fleder said. “You only get a tiny slice of what people are spending and you have to extrapolate and infer what’s really going on from that fraction of data.”
“That requires a bit of untangling the numbers,” he continued. “If we observe 1 percent of a company’s weekly sales through credit card transactions, how do we know it’s 1 percent? And if the credit card data is noisy, how do you know how noisy it is? We don’t have access to the ground truth for daily or weekly sales totals. But the quarterly aggregates help us reason about those totals.”
Using a variation on the standard inference algorithm, the technique calculates the probability of unknown sales given the known credit card data. To do that, it first breaks sales into chunks of days, matching the credit card data to the unknown daily sales with an allowed day-to-day variance. In addition to the estimated daily sales, the technique also produces metrics for the noise level and estimated prediction error range. On the 34 companies tested, the MIT researchers' model beat an aggregate Wall Street analyst benchmark in 57.2 percent of quarterly predictions tested in the experiment.
Looking to the future, the researchers hope to incorporate location information, along with other forms of alternative data that may prove useful as proxies for sales data. They also highlighted potential uses for people outside of the finance sector – for instance, political scientists studying social behaviors. “It’ll be useful for anyone who wants to figure out what people are doing,” Fleder said. “This isn’t all we can do. This is just a natural starting point.”
About the research
The research discussed in this article was published as "Forecasting with Alternative Data" in the December 2019 issue of Proceedings of the ACM on Measurement and Analysis of Computing Systems. It was written by Michael Fleder and Devavrat Shah and can be accessed here.