Startup Applies Deep Learning to Secure Unstructured Data
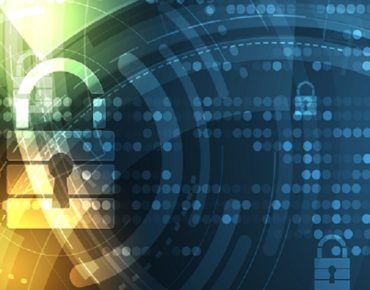
via Shutterstock
The millions of documents in the form of product roadmaps, contracts and other components of corporate strategies also represent unstructured—and it turns out—vulnerable data stored in-house or in the cloud.
A data security startup called Concentric emerged from stealth mode this week, claiming to be the first to use deep learning tools to help companies determine where unstructured data resides and how to protect it. While quantifying data security challenges, Concentric further claims its “semantic intelligence” platform generates insights that can be used to protect strategic data while also meeting data governance mandates.
The San Jose-based startup launched by industry veterans also on Wednesday (Jan. 29) announced a $7.5 million funding round led by Clear Ventures.
“Unstructured data is now the industry’s primary threat surface because it’s highly dispersed and comes in all forms, and it’s tough to protect business-critical content,” said Chris Rust, Clear Venture’s founder and managing partner.
Concentric’s automated approach applies deep learning to generate semantic understanding of unstructured data. According to the startup, its framework discovers, categorizes and classifies business documents. A separate data risk report released by the startup notes that enterprise datacenters often contain millions of unsecured documents that can be inappropriately shared across organizations.
Along with preventing data breaches via “oversharing,” the approach is said to shield users from fines associated with a growing list of data privacy regulations.
The security challenge posed unstructured corporate data ranging from payroll information to source code is growing. Concentric estimates an average company generates about 10 million documents, of which an estimated 1.2 million are deemed “business critical.”
More than 80 percent of enterprise data is unstructured, according the data security study, meaning it is embedded in documents and source code files spread across organizations. Those data become even more vulnerable as employees “overshare” data with inadequate security classifications.
“An extreme amount of data is left unsecured, unidentified, misclassified and at risk,” said Karthik Krishnan, Concentric’s CEO and co-founder. "Unstructured data is currently copious and dispersed, and it includes an alarming amount of business-critical information.”
The startup notes that current security frameworks used to protect databases or restrict access don’t cover unstructured data. Given the scope of the unstructured data security problem, its semantic platform seeks to automate a task that would overwhelm IT teams already coping with constant false alarms.
Concentric provided few technical details about its semantic intelligence platform, but claims to have scanned 26 million unstructured data files from customers in the financial and healthcare sectors. It’s deep learning approach generally focuses on excessive sharing of business documents. The framework applies a formula that weighs the material damage resulting from a security breach with inappropriate sharing of documents.
Oversharing of unstructured company data is seen as critical since it “dramatically increases the threat surface,” the data security study found.
The startup’s founders previously worked at networking and security firms, including Aruba Networks, Hewlett Packard Enterprise, Juniper Networks, PGP Corp. and its parent, Symantec.
Machine learning approaches designed to help automate data security are gaining traction in both the public and private sectors. For example, the Defense Advanced Research Projects Agency announced an effort last year to help plug security gaps in enterprise networks. Threat detection algorithms developed under the DARPA program could for instance be used to respond to threats “in the context of different data types and sources,” the agency said.
Related
George Leopold has written about science and technology for more than 30 years, focusing on electronics and aerospace technology. He previously served as executive editor of Electronic Engineering Times. Leopold is the author of "Calculated Risk: The Supersonic Life and Times of Gus Grissom" (Purdue University Press, 2016).