WekaIO Launches DataOps AI Pipeline for Data-Starved GPUs
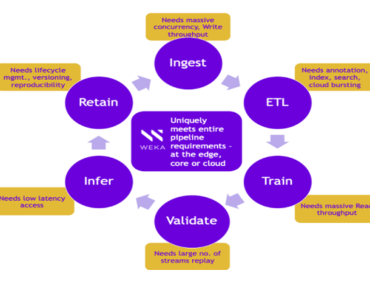
source: WekaIO
WekaIO, the high-performance file storage specialist, today launched Weka AI, a DataOps “storage solution framework” designed to cut friction in edge-core-cloud data AI pipelines.
The company said the offering enables a single namespace for data pipeline visibility while addressing the variety of storage IO requirements at each stage of AI data pipelines: for ingest and training large bandwidth is required while mixed read/write is needed for ETL (extract, transform, load) and low latency is the priority for inference.
Weka AI fits into the “DataOps” category, an offshoot of DevOps that Amita Potnis, research director, Enterprise Infrastructure Practice at industry analyst firm IDC, called “a new class of intelligent data operations platforms … that can reduce friction, improve efficiencies with automation and provide flexibility and openness with policy and metadata-driven processes that can accommodate the diversity and distribution of data in modern environments.”
Comprised of the Weka File System, customizable reference architectures and software development kits based on partnerships with Nvidia and Mellanox, Weka AI delivers more than 73 GB/sec of bandwidth to a single GPU client, according to WekaIO, adding that it delivers “operational agility with versioning, explainability and reproducibility and provides governance and compliance with in-line encryption and data protection.”
“AI data pipelines are inherently different from traditional file-based IO applications,” the company said. “So the ideal solution must meet all these varied requirements and deliver timely insights at scale. Traditional solutions lack these capabilities and often fall short in meeting performance and shareability across personas and data mobility requirements… The solutions must provide data management that delivers operational agility, governance, and actionable intelligence by breaking silos.”
Looking across the data pipeline landscape, Shailesh Manjrekar, WekaIO’s head of AI and strategic alliances, said in a blog, “in order to cater to complex DNNs (dynamic neural networks) and the convergence of HPC, HPDA, and AI, architectures such as GPUDirect storage are becoming paramount to feeding the GPU memory directly, providing the highest bandwidth and lowest latencies. Sharing datasets for distributed training across 64 DGX-2s (5,000 cores x 16 Teslas x 64 DGX-2s) with SuperPODs has become the norm. Imagine the parallelism that is involved at the compute layer. Transports such as NVMeOF (over InfiniBand or RoCE Fabrics) are making data locality a non-issue, especially with support for 100 Gb/sec and 200 Gb/sec networking.”
“End-to-end application performance for AI requires feeding high-performance NVIDIA GPUs with a high-throughput data pipeline,” said Paresh Kharya, director of product management for accelerated computing, Nvidia. “Weka AI leverages GPUDirect storage to provide a direct path between storage and GPUs, eliminating I/O bottlenecks for data intensive AI applications.”