Breaking Down COVID-19 Models – Limitations and the Promise of Machine Learning
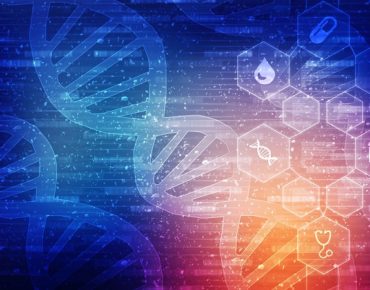
Every major news outlet offers updates on infections, deaths, testing, and other metrics related to COVID-19. They also link to various models, such as those on HealthData.org, from The Institute for Health Metrics and Evaluation (IHME), an independent global health research center at the University of Washington. Politicians, corporate executives, and other leaders rely on these models (and many others) to make important decisions about reopening local economies, restarting businesses, and adjusting social distancing guidelines. Many of these models possess a shortcoming—they are not built with machine learning and AI.
Predictions and Coincidence
Given the sheer numbers of scientists and data experts working on predictions about the COVID-19 pandemic, the odds favor someone being right. Like the housing crisis and other calamitous events in the U.S., someone took credit for predicting that exact event. However, it’s important to note the number of predictors. It creates a “multiple hypothesis testing” situation where the higher number of trials increases the chance of a result via coincidence.
This is playing out now with COVID-19, and we will see in the coming months many experts claiming they had special knowledge after their predictions proved true. There is a lot of time, effort, and money invested in projections, and the non-scientists involved are not as eager as the scientists to see validation and proof. AI and machine learning technologies need to step into this space to improve the odds that the “right” predictions were very educated projections based on data instead of coincidence.
Modeling Meets its Limits
The models predicting infection rates, total mortality, and intensive care capacity are simpler constructs. They are adjusted when the conditions on the ground materially change, such as when states reopen; otherwise, they remain static. The problem with such an approach lies partly in the complexity of COVID-19’s different variables. These variables mean the results of typical COVID-19 projections do not have linear relationships with the inputs used to create them. AI comes into play here, due to its ability to ignore assumptions about the ways the predictors building the models might assist or ultimately influence the prediction.
Improving Models with Machine Learning
Machine Learning, which is one way of building AI systems, can better leverage more data sets and their interrelated connections. For example, socioeconomic status, gender, age, and health status can all inform these platforms to determine how the virus relates to current and future mortality and infections. It’s enabling a granular approach to review the impacts of the virus for smaller groups who might be in age group “A” and geographic area “Z” while also having a preexisting condition “X” that puts people in a higher COVID-19 risk group. Pandemic planners can use AI in a similar way as financial services and retail firms leverage personalized predictions to suggest things for people to buy as well as risk and credit predictions.
Community leaders need this detail to make more informed decisions about opening regional economies and implementing plans to better protect high-risk groups. On the testing front, AI is vital for producing quality data that are specific for a city or state and takes into account more than just basic demographics, but also more complex individual-based features.
Variations in testing rules across the states require adjusting models to account for different data types and structures. Machine learning is well suited to manage these variations. The complexity of modeling testing procedures means true randomization is essential for determining the most accurate estimates of infection rates for a given area.
The Automation Advantage
The pandemic hit with crushing speed, and the scientific community has tried to quickly react. Enabling faster movement with modeling, vaccine development, and drug trials is possible with automated AI and machine learning platforms. Automation removes manual processes from the scientist’s day, giving them time to focus on the core of their work, instead of mundane tasks.
According to a study titled “Perceptions of scientific research literature and strategies for reading papers depend on academic career stage,” scientists spend a considerable amount of time reading. It states, “Engaging with the scientific literature is a key skill for researchers and students on scientific degree programmes; it has been estimated that scientists spend 23% of total work time reading.” Various AI-driven platforms such as COVIDScholar use web scrapers to pull all new virus-related papers, and then machine learning is used to tag subject categories. The results are enhanced research capabilities that can then inform various models for vaccine development and other vital areas. AI is also pulling insights from research papers that are hidden from human eyes, such as the potential for existing medications as possible treatments for COVID-19 conditions.
Machine learning and AI can improve COVID-19 modeling as well as vaccine and medication development. The challenges facing scientists, doctors, and policy makers provide an opportunity for AI to accelerate various tasks and eliminate time-consuming practices. For example, researchers at the University of Chicago and Argonne National Laboratory collaborated to use AI to collect and analyze radiology images in order to better diagnose and differentiate the current infection stages for COVID-19 patients. The initiative provides physicians with a much faster way to assess patient conditions and then propose the right treatments for better outcomes. It’s a simple example of AI’s power to collect readily available information and turn it into usable insights.
Throughout the pandemic, AI is poised to provide scientists with improved models and predictions, which can then guide policymakers and healthcare professionals to make informed decisions. Better data quality through AI also creates strategies for managing a “second wave” or a future pandemic in the coming decades.
About the Author
Pedro Alves is the founder and CEO of Ople.AI, a software startup that provides an Automated Machine Learning platform to empower business users with predictive analytics.
While pursuing his Ph.D. in Computational Biology from Yale University, Alves started his career as a data scientist and gained experience in predicting, analyzing, and visualizing data in the fields of social graphs, genomics, gene networks, cancer metastasis, insurance fraud, soccer strategies, joint injuries, human attraction, spam detection and topic modeling among others. Realizing that he was learning by observing how algorithms learn from processing different models, Alves discovered that data scientists could benefit from AI that mimics this behavior of learning to learn to learn. Therefore, he founded Ople to advance the field of data science and make AI easy, cheap, and ubiquitous.
Alves enjoys tackling new problems and actively participates in the AI community through projects, lectures, panels, mentorship, and advisory boards. He is extremely passionate about all aspects of AI and dreams of seeing it deliver on its promises; driven by Ople.