Qeexo Takes ‘TinyML’ to AWS Cloud
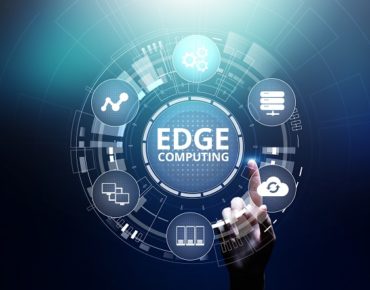
via Shutterstock
Qeexo, the Carnegie Mellon University spinoff, is expanding public cloud access to its automated machine learning platform as it pushes its no-code “TinyML” approach to the network edge.
The company this week announced general availability of its AutoML platform as a web application hosted on Amazon Web Services (NASDAQ: AMZN). Expanded cloud access is designed to boost TinyML model development and deployment “from novices to expert data scientists,” said Qeexo CEO Sang Won Lee.
Along with public cloud access, the no-code platform now includes new machine learning algorithms, control features and sensors designed to simplify training of machine learning models using device data. The combination is touted as accelerating deployment of models in edge devices for Internet of Things scenarios.
Qeexo’s AutoML framework is based on technology the company originally developed for a platform called FingerSense deployed on Android smartphones. Among Qeexo’s earliest customers was Chinese telecom giant Huawei, which implemented the startup’s ML-based finger-gesture application in its mobile phones.
That labor-intensive early application prompted the company to develop its AutoML platform to generate models based on sensor and other data types, Lee told sister web site Datanami in February.
Access to the automated machine learning capabilities on AWS is aimed at extending the company’s framework to edge devices and IoT sensors via its TinyML approach. A free package available for a limited time gives users access to data sets used to automate building of “lightweight” ML models that can be deployed and tested on embedded hardware.
The framework is currently being used on IoT devices such as STMicroelectronics’ (NYSE: STM) SensorTile module.
The latest release also adds support for recurrent neural networks that arrive at decisions based on previous learning. Also supported is a classifier capability tailored to anomaly detection in industrial applications. That’s on top of existing algorithm support for artificial and convolutional neural networks, decision trees and other models.
The AutoML platform can then be used for sensor data collection and data visualizations applied to automated model building and “one-click” deployment.
Among the potential TinyML applications are development of machine learning algorithms for smart IoT devices, the company said Monday (June 8).
Qeexo announced its AWS partnership earlier this year, moving model training from laptops to the cloud. It also announced support for chip maker Renesas’ (OTCMKTS: RNECY) family of Cortex-M microcontrollers aimed at low-power IoT applications.
Despite limited MCU memory and processing power, Lee noted that tools like TinyML could enable viable machine learning applications. “You don’t want to neglect all the sensor data that’s connected to the microcontroller,” he told Datanami.
Related
George Leopold has written about science and technology for more than 30 years, focusing on electronics and aerospace technology. He previously served as executive editor of Electronic Engineering Times. Leopold is the author of "Calculated Risk: The Supersonic Life and Times of Gus Grissom" (Purdue University Press, 2016).