AI Trust Remains an Issue in the Life Sciences
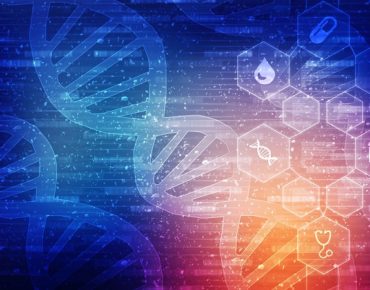
The rise of AI – machine and deep learning – in life sciences has stirred the same excitement and skepticism as in other fields of scientific research.
AI is mostly used in two areas of life sciences. The first is embedded in instruments such as cryo-electron microscopes, where AI tools assists in feature recognition. Those tools are mostly hidden from users.
The other application includes high profile projects such as the National Cancer Institute-Department of Energy effort known as CANDLE (NCI-DoE’s CANcer Distributed Learning Environment), which has access to supercomputing capacity and plenty of AI expertise.
AI is, of course, being used against COVID-19 at many large labs. There’s not much in between.
AI nevertheless remains in its infancy elsewhere in life sciences. The appetite for its use is high but so are the early obstacles—not least the hype which has already ticked off clinicians. Another stumbling block is uneven data quality, non-optimal compute infrastructure and limited AI expertise.
On the plus side, practical pilot programs are emerging.
Sister website HPCwire spoke with Fernanda Foertter, senior scientific consultant at BioTeam, the research computing consultant. An AI specialist, Foertter joined Bioteam from Nvidia where she worked on AI for healthcare. Foertter also did a stint at Oak Ridge National Laboratory as a data scientist working on scalable algorithms for data analysis and deep learning.
What got the AI ball rolling at research agencies was natural language processing (NLP), especially within the Energy Department’s supercomputer initiatives, Foertter noted. “We have the CANDLE project whose three pilots had the main, basic AI applications [like] NLP, using AI for accelerating molecular dynamics and drug discovery.”
“NLP is actually working really well, [and] the molecular dynamics is working really well,” Foertter added. “The drug discovery issue was they didn't have the right data to begin with. So, they're still generating data, in vivo data, for that.”
The application of HPC to AI also has been used extensively in COVID-19 research. It seems reasonable to expect those efforts will not only bear fruit against the pandemic, but also generate new approaches for using HPC cum AI in life sciences research.
Still, making AI work in clinical settings remains challenging.
When Foertter joined Nvidia in 2018), medical imaging applications were emerging. Those expectations have since been tempered. “We went from talking about, “Can we discover pneumonia? Can we discover tumors?’ to talking about being able to grade tumors, which is much more refined. The one application I think everybody wishes would happen really, really quickly, but hasn't really materialized, is digital pathology,” said Foertter.
The workflow remains challenging. “Somebody has to go through a picture that has a very, very high pixel number,” she continued. “They choose a few points and they have experience, and the miss rate is… anywhere between 30 percent to 40 percent. That means you can send somebody to pathology and they're going to miss that you have cancer….”
Large image formats also have slowed digital pathology. “To do any sort of convolution neural network on a really large image to train it would just break it,” Foertter explained. “Just the memory size is really hard.”
AI hype also has turned off clinicians, particularly claims the technology would make them obsolete. “There's a lot of animosity [among] physicians. The whole AI thing was kind of sold as if it could replace a lot of folks,” an impression that AI vendors moved quickly to correct.
Hence, trust and the ability to see and analyze data used for training and inference remain issues within the medical the medical community.
“AI is still seen as a black box,” Foertter stressed.
Read John Russell’s full report detailing AI’s impact on the life sciences here.