A New Approach to AIoT Data Infrastructure Using NVMe-oF Shared Flash Storage
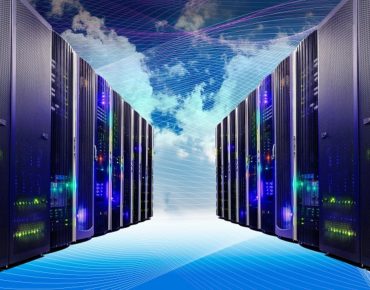
The Internet of Things (IoT) is projected to comprise nearly 30 billion connected devices by 2023. These IoT devices create and transfer massive amounts of data over the network. But how do data owners derive intelligence from the data being transferred? That’s where AIoT comes in, the combination of artificial intelligence (AI) and IoT.
AIoT adds intelligent processing to the large and varied data sets collected by IoT devices. It enables businesses to analyze data and deliver insights. AIoT is alive today in connected use cases such as healthcare for remote patient monitoring and preventative medicine; in robotics within manufacturing; in autonomous vehicles; in network surveillance; and in research such as the C3.ai Digital Transformation Institute striving to mitigate pandemics and prevent future infectious outbreaks. AIoT is a powerful tool for any use case where sifting through mountains of data quickly is required to execute deep learning algorithms. It is self-learning, self-monitoring and self-healing. AIoT enables autonomous decision making with high predictive accuracy that far surpasses human beings.
The challenge for AIoT is that systems involve complex data pipelines with multiple phases. It’s not just the volume, variety, velocity and veracity of data from IoT that businesses must handle, but also the need to maintain model quality, data access latency, throughput and data caching capabilities when implementing AI solutions. If compute systems are optimized to process data fast, then getting data into those systems can be a bottleneck unless the right combination of compute, storage and memory is built.
Storage is the critical foundation and needs to address all phases of the AIoT data pipeline, from ingestion to data preparation to model training and inference, with careful consideration to TCO, performance and power requirements. It also needs to meet the changing needs of AI workloads. Enterprises are turning to NVMe flash storage for the high throughput and low latency AI requires, but let’s take it one step further with NVMe over Fabrics (NVMe-oF)
A New Approach – Shared Storage
A new approach is to use composable disaggregated infrastructure (CDI) with NVMe flash storage, GPU pools, and a high-capacity storage system to enable the delivery of rapid response times and the scaling requirements of AI in a dynamic, software-defined application environment.
CDI takes physically disaggregated resources (compute, networking, storage and GPUs) and pools them together as needed for a specific application. This enables flexible, independent scaling of resources to meet the changing needs of AI workloads.
Composable storage will significantly increase the agility and flexibility in how enterprises provision and optimize their data infrastructure to meet dynamic application requirements.
CDI allows for sharing and scaling storage, network and compute resources across many hosts. Unlike converged systems, it avoids the latency of subsequent data transfers in and out of local SSDs of GPU servers as the data grows over capacity in the servers. It can provide immediate access to the trained models and data on shared flash storage and enables rapid response times.
When selecting a storage solution, open composable infrastructure with NVMe flash can seamlessly allocate the shared storage pool across teams to improve efficiency, cost and the KPI metrics CIOs care about.
How to Implement NVMe-oF Shared Storage Across the AIoT Data Pipeline
When implementing an AI initiative, it’s important to design a storage infrastructure that can support the unprecedented volume of AIoT data. One way to optimize TCO and efficiency is to look at each phase of an AI workload to determine which type of storage is best suited at each juncture. NVMe-oF shared storage is suitable for virtually all stages of the AIoT workflow:
- Ingest – The ingest phase needs to have the speed and scale to sustain the volume and velocity of the incoming data from IoT systems. For ingestion to a temporary landing zone, you can use NVMe storage platforms or high-capacity storage systems (HDD-based, object or cloud storage), whereas for ingestion to a centralized, globally accessible capacity tier, one can use high-capacity storage systems. For ingestion to a high-performance tier, whether deployed on-premises or on the cloud, NVMe flash is needed for real-time analytics.
- Data Preparation – The primary focus in the data preparation stage should be on data quality. NVMe storage platforms are also a good choice both in terms of cost and performance. Or, you can go with a hybrid option of flash and HDDs, which offer the benefit of performance and higher capacity.
- Model Training - The model training phase is sensitive to model quality, data access latency, throughput and data caching capabilities of the implemented AI solution. This requires a low-latency, throughput-oriented, scalable, high-performance storage tier, and NVMe-oF storage platforms are well-suited to address these needs.
- Inference – The inference phase, similarly, requires low data-access latency, throughput response times, and data-caching capabilities. The model training and inference phases are heavily interdependent as they access one shared storage in a disaggregated architecture. For example, if the inference score is poor, the model needs to be retrained, and until the model training is over, inference can’t be generated. Therefore, it makes sense to use a shared storage pool of the same type for both.
- Backup/Restore – In the backup phase, high-capacity HDD, object storage or cloud storage is best suited for storing and on-demand access of old models and data. Old models often need to be restored from backup for inference or retraining to meet the needs of new incoming IoT data, so leveraging a nearline or online backup solutions is best.
Composable Disaggregated Infrastructure with NVMe-oF for AIoT
NVMe-oF is unifying how storage is shared, composed and managed at scale to meet the demands of increasingly varied applications and workloads. A composable disaggregated infrastructure using NVMe flash storage allows IT to allocate storage on the fly to support AIoT implementations at each phase of AI.
We’ll continue to see increasing adoption of composable disaggregated storage solutions that efficiently scale over Ethernet fabrics and deliver the full performance potential of NVMe devices to diverse data center applications. Composable storage will significantly increase the agility and flexibility in how enterprises provision and optimize their data infrastructure to meet the dynamic application requirements of AIoT and its data center KPIs.
Sanhita Sarkar is a Global Director, Software Development, at Western Digital, where she focuses on systems architecture, AI data pipelines, and development of features and solutions spanning edge, data center, data hub, and cloud. She has experience in key vertical markets such as the Industrial Internet of Things (IIoT), Defense and Intelligence, Financial Services, Genomics, and Healthcare. Sanhita previously held leadership positions at Teradata, SGI, Oracle, and a few startups. She was responsible for overseeing design, development, and delivery of optimized software and solutions involving large memory, scale-up, and scale-out systems. Sanhita has multiple patents, published several papers, and presented at various conferences and meetups. She received her Ph.D. in Electrical Engineering and Computer Science from the University of Minnesota, Minneapolis.