OpenAI’s GPT-3 Language Generator Is Impressive, but Don’t Hold Your Breath for Skynet
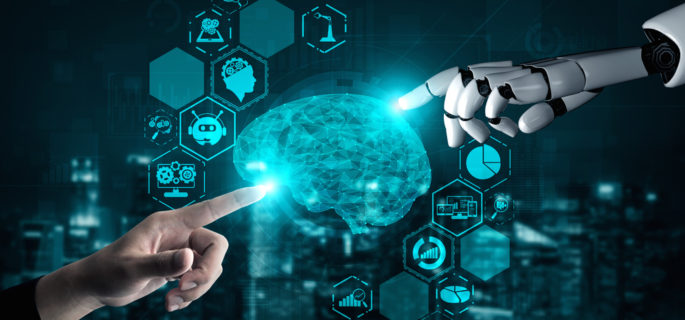
Just a couple of months after releasing a paper describing GPT-3, AI development and deployment company OpenAI has begun testing its novel, AI-powered language generator with a select group of users through a private beta – and many have been understandably wowed. Fed only with a handful of words, the tool can generate articles, short stories and even poetry that – in many cases – convincingly conveys facts, answers and writing styles. Still, GPT-3 often trips up in surprisingly rudimentary ways, pulling the curtain back on how convincing imitation of human speech patterns doesn’t necessarily require genuine cognition.
GPT-3 is an autoregressive language model with a staggering 175 billion parameters, which OpenAI claims is ten times more than any previous non-sparse language model. This allows GPT-3 to achieve remarkable results in many translation, question-answer and text generation tasks with no fine-tuning using only a small amount of training data. Asked to produce a poem in the style of Wallace Stevens titled “Shadows on the Way,” for instance, GPT-3 wrote (in part):
The sun was all we had. Now, in the shade
All is changed. The mind must dwell on those
White fields, that to its eyes were always old;
Those ancient gleams, convoluting
The way they lay among the huge roots,
The great dark tomes of reverie,
The plumed labyrinth of the sea.
The early users are, of course, pushing the limits of GPT-3. Twitter user Mario Klingemann had GPT-3 imitate Jerome K. Jerome writing an essay about “The importance of being on twitter,” opening “It is a curious fact that the last remaining social life in which the people of London are still interested is Twitter.” In its research, OpenAI found that the samples of news articles generated by GPT-3 proved difficult for humans to distinguish from human-written articles.
GPT-3 can even understand the syntax of other kinds of language, like music composition and coding. Another early-access user, Sharif Shameem, reported building a layout generator with GPT-3 that could build JSX code for functional website layouts using just a description of the desired layout.
However, GPT-3 still has significant blind spots. Kevin Lacker, another early-access user, put GPT-3 through the hoops of a basic Turing test. It was doing well, until it started answering questions like “How many eyes does my foot have?” and “How do you sporgle a morgle?” with answers like “Your foot has two eyes” and “You sporgle a morgle by using a sporgle.”
Perhaps more troubling, GPT-3 is prone to another flaw of automated language repetition: bigotry. Unfortunately, simple prompts to GPT-3 on subjects like “black” or “Jews” sometimes generated offensive sentences – a problem that required fine-tuning to resolve in GPT-2.
The near-magical results of GPT-3 seem almost incompatible with these slightly rudimentary failures, prompting polarization in the public response to the tool’s early launch. Veteran coder John Carmack said that GPT-3’s coding abilities “generated a slight shiver”; but Twitter user Simon Sarris quipped, “we aren’t pulling the mask off the machine to reveal a genius wizard, we’re pulling the mask off each other to reveal the bar is low.”
GPT-3 is slated for a commercial release later this year.