Cerebras Second-Gen 7nm Wafer Scale Engine Doubles AI Performance Over First-Gen Chip
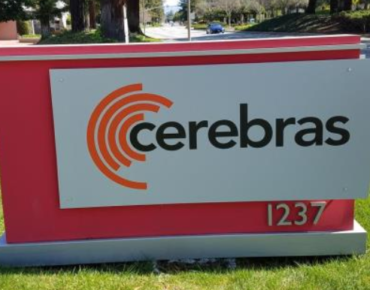
Cerebras Systems has just given a massive compute boost to its latest second-generation Wafer Scale Engine (WSE-2) AI chips, which pack twice the performance of the company's original WSE-1 chips that debuted almost two years ago at the Hot Chips conference.
The original WSE-1 included 1.2 trillion transistors and 400,000 cores on an 8″x8″ silicon footprint. The new WSE-2 more than doubles that chip density, with 2.6 trillion transistors and 850,000 cores.
“We’re going bigger, faster and better in a more power efficient footprint,” Cerebras Founder and CTO Andrew Feldman told HPCwire ahead of yesterday’s launch.
The new chip, made by TSMC on its 7nm node, delivers 40 GB of on-chip SRAM memory, 20 petabytes of memory bandwidth and 220 petabits of aggregate fabric bandwidth. Gen over gen, the WSE-2 provides about 2.3X on all major performance metrics, said Feldman.
For AI customers, the new chips will enable new workloads and possibilities while ramping up compute power for users. Earlier Cerebras WSE-1 chips are already being used by a wide range of customers, including Argonne National Laboratory, Lawrence Livermore National Laboratory, Pittsburgh Supercomputing Center (for its Neocortex AI supercomputer), the EPCC supercomputing center at the University of Edinburgh, pharmaceutical leader GlaxoSmithKline and Tokyo Electron Devices.
Compared to the the largest GPU, which has ~54 billion transistors, the WSE-2 is 2.55 trillion transistors larger. Further, Cerebras claims its new platform has 123 times more cores, 1,000 times more on-chip memory, more than 12,000 times the memory bandwidth and more than 45,000 times the fabric bandwidth as the leading GPU.
Both Cerebras’ first and second generation chips are created by removing the largest possible square from a 300 mm wafer to create 46,000 square millimeter chips roughly the size of a dinner plate. An array of repeated identical tiles (84 of them) is built into the wafer, enabling redundancy.
To drive its new engine, Cerebras designed and built its next-generation system, the CS-2, which it bills as the industry’s fastest AI supercomputer. Like the original CS-1, CS-2 employs internal water cooling in a 15 unit rack enclosure with 12 lanes of 100 Gigabit Ethernet.
The new system has a max power draw of 23 kW, up from 20 kW max for the original chassis. “We tried to stay in the original power envelope, and made some changes in the system to take full advantage of the power envelope,” said Feldman.
Feldman said that the last two years have taught him how valuable it is for a system to be physically easy to deploy. The “CS” systems weigh approximately 500 lbs, about the same as 15 RU servers, but deploy in just 15 minutes, he said, adding that cabling projects on a typical cluster can take weeks.
Looking further back to when Cerebras was still designing its first-generation product and charting its go-to-market strategy, Feldman said that he originally underestimated the size of the market due to how quickly the space is moving.
“In my career, I’ve always misestimated on the too big side. I’ve always assumed the market was going to be bigger than it is,” he shared. “In 2015, I estimated the market will be smaller than it is, and the demand for AI and the the rate of innovation.
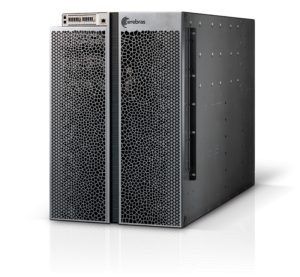
“We’re selling a lot of systems to do BERT. BERT didn’t exist the first half of 2018, right? That’s a quick moving market if what hadn’t yet existed until Q3 2018 is [now] the bulk of business. And things that are brand new, like graph neural networks, are piquing people’s interest and top of everybody’s mind. The market is moving unbelievably quickly.”
Making the leap to wafer-scale
As Feldman describes it, codes that have been optimized for CS-1’s 400,000 cores will scale to leverage CS-2’s 850,000 cores without any modification. Further, he attests that GPU codes are easy to port to the Cerebras platform. “We can take as input any TensorFlow or PyTorch model designed for a GPU. You define your model and write it in TensorFlow, that’s your model function. You define your parameters, that’s your input function. All you have to do is take your TensorFlow code, and type one thing: “est = CerebrasEstimator.
Primarily dedicated to AI computing, the Cerebras engine is also being applied to HPC workloads. At last year’s Supercomputing Conference, researchers from Cerebras and NETL revealed how the CS-1 was being used perform fast stencil-code computation (a CFD code), demonstrating a speed up of 200X over the largest existing supercomputers.
“That’s how you take a model that was written for a GPU and run it on our machine,” he said. “Each layer of your neural network is converted into a region of compute. Then we configure a circuit through it, begin streaming your data, and we send out the answers.”
The CTO further claimed it is easier to go from a model written for one GPU to a Cerebras CS-1, than it is to go from a model written for one GPU to 20 GPUs.
How big is too big?
With a chip this size, the question arises as to whether users can fill up all that silicon real estate? Are today’s models able to take advantage of an 850,000 core machine? Feldman said there’s no question the demand is there for the larger Cerebras machine, and he sees smaller size platforms (including GPUs) as being primarily for entry-level use cases.
“If you’re happy on one GPU, then we’re not the machine for you,” he said. “But we think that is a small part of the market. As soon as you do work on one GPU, you want more, you want to write bigger models. When you use AI to further the mission of your organization – those are our customers, not the hobbyist.”
Users who need a smaller slice of the Cerebras engine may still be in luck, however. Cerebras plans to announce a cloud offering down the road, according to Feldman.
Shipping Timelines
CS-2 systems will begin shipping in the third quarter of this year, according to Cerebras, and current customers GlaxoSmithKline and Argonne National Lab are expected to be among the the first to take delivery of the upgraded machines.
“At GSK, we are pioneering the use of AI in drug discovery and design,” said Kim Branson, executive vice president of AI, GlaxoSmithKline. “We have been early adopters of the Cerebras technology and have found extraordinary speedups over our legacy infrastructure. We are excited to receive delivery of our CS-2.”
“As an early customer of Cerebras solutions, we have experienced performance gains that have greatly accelerated our scientific and medical AI research,” said Rick Stevens, Argonne National Laboratory associate laboratory director for computing, environment and life sciences, in a statement. “The CS-1 allowed us to reduce the experiment turnaround time on our cancer prediction models by 300X over initial estimates, ultimately enabling us to explore questions that previously would have taken years, in mere months. We look forward to seeing what the CS-2 will be able to do with more than double that performance.”
This article first appeared on sister website HPCwire.
Related
With over a decade’s experience covering the HPC space, Tiffany Trader is one of the preeminent voices reporting on advanced scale computing today.