ModelOps: The Key to Managing AI Models at Scale
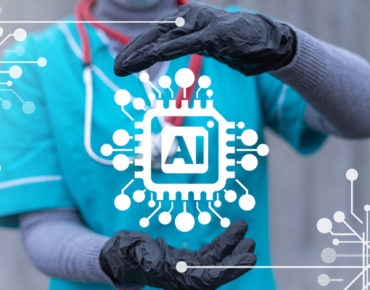
AI is being used by enterprises to solve major business problems, anticipate future activities and leverage data in ways that were impossible even a few short years ago. Yet, while creating predictive algorithms has become the standard practice of data scientists using innovative tools and technologies, companies still struggle with effectively deploying and maintaining those algorithms in what is often called the “last mile” of the AI journey.
ModelOps, which operationalizes AI models, has been gaining traction to effectively automate the rollout and maintenance of AI, carrying it across the finish line and ensuring that it continues to improve and increase in value.
The complexity of AI is clearly driving this new practice. As AI continues to proliferate, the number of algorithms deployed to address specific business problems also continues to grow, so organizations must deploy multiple algorithms to attack new problems. As an extreme case, consider Alexa – you would need an army of people to update the hundreds of algorithms that must be created to answer a host of new questions.
So, what is the answer? That is where automating the AI lifecycle comes in as the only way to handle the growing armies of algorithms.
Defining ModelOps
ModelOps is focused primarily on the governance and life cycle management of a wide range of AI models, according to research firm, Gartner. It automates the development, validation, scoring, deployment, governance and maintenance of AI solutions. ModelOps helps companies shorten production cycles and deliver results to end-users quickly at scale, while also continuously improving the results.
ModelOps shares many similarities with DevOps, a set of practices which integrate software development and IT operations to help shorten software development lifecycles and enable continuous updates. Both practices aim to remove the siloes between software engineers or data scientists and IT to make it easier to get projects running and keep them working smoothly.
In ModelOps, collaborating between data science teams and IT ensures that the data used to train AI models also takes into consideration the operational data that will be used in production, as well as the modeling and retraining that will be required down the road. Since IT professionals are not always trained to understand analytical models, it can be difficult to deploy them without the support of data scientists.
Armed with ModelOps, here are four steps that enterprises can follow to standardize and automate the process of effectively initiating ModelOps and ensuring that it becomes a key methodology for AI development and deployment.
Remove the Siloes
It is essential to instill a sense of collaboration enterprise-wide, but it is especially so between data scientists, software engineers, IT departments and business leaders. Everyone must have a clear idea of the end goal and use the same processes and rules to deliver analytics-driven outcomes that can be constantly improved upon. This collaboration requires a deep commitment from the C-suite, which must fully and clearly communicate across the organization about the value of the AI processes being created, its role in the success of the business and the expectations for a continuous improvement mindset.
Assess the Current State of Affairs
Before implementing ModelOps, it is important to understand where you currently are in terms of AI development effectiveness and automated data collection. You need to have a handle on how many models are in operation, how they are used and who developed them. You also need to know what data is used to train them, how accurate they are and how they can be leveraged for future algorithms. But you also need to know where the challenges are, what typically delays production and what causes the most friction. By knowing where you are at the beginning, these factors will help you determine where to start and how to begin to automate the AI process journey.
Establish the Rules of Operations and Automation
Building a ModelOps strategy can be difficult when organizations have different programming languages in operation, little collaboration between teams and different procedures in place. It can be even more difficult when teams use manual processes to train data, add data, score data, and assess effectiveness of the algorithm. By automating these laboriously manual processes, organizations not only reduce errors and produce highly accurate and relevant algorithms, but they also eliminate AI bias, by more easily reviewing input data to ensure that is it diverse, fair and explainable.
Monitor KPIs
Once your model is deployed, it is important to monitor it against agreed-upon Key Performance Indicators (KPIs). This ensures that it is continuing to meet its intended purpose, that it is constantly being trained with diverse and relevant data and that it is growing in accuracy. By automating model operations across the many algorithms that may be in operation, you gain a holistic view to better streamline model governance, explainability and workflow analytics.
As businesses, governments and others continue to rely upon hundreds of algorithms to address complex problems, the need for ModelOps to manage them all is growing in importance. ModelOps is enabling AI to be rolled out faster and more efficiently than ever before, while helping enterprises quickly adapt to changing market needs as it incrementally makes AI more intelligent and relevant. But to make it a true success inside an organization, it requires a collaborative mindset across data scientists, operations teams and the company at large, working together to maximize the value of AI.
With those goals reached, ModelOps can truly be a useful strategy to traversing that last mile on the road to improved and stealthier AI development.
About the Author
Carlos M. Meléndez is the COO and Co-Founder of Wovenware, a Puerto Rico-based design-driven company that delivers customized AI and other digital transformation solutions that create measurable value for customers across the U.S.