With ‘Dojo’ in the Future, Tesla Reveals Its Massive Precursor Supercomputer
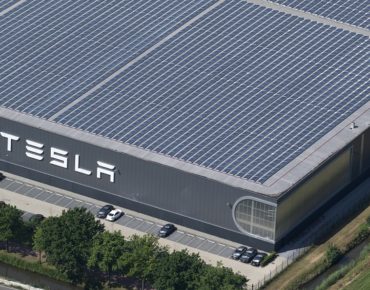
Tesla first made a cryptic reference to it's project Dojo, a “super-powerful training computer” for video data processing, in 2019. Later, in the summer of 2020, Tesla CEO Elon Musk tweeted: “Tesla is developing a [neural network] training computer called Dojo to process truly vast amounts of video data. It’s a beast! … A truly useful exaflop at de facto FP32.”
Well, now it is the summer of 2021, so it must be time for your annual Dojo update. But instead of revealing the latest ins and outs of Dojo, Tesla has instead opted to reveal a precursor cluster that the company estimates may be the fifth most-powerful supercomputer in the world.
The casual reveal happened during a talk by Andrej Karpathy, the senior director of AI at Tesla, at the 4th International Joint Conference on Computer Vision and Pattern Recognition (CCVPR 2021). “I wanted to briefly give a plug to this insane supercomputer that we are building and using now,” Karpathy said. As he explained, the cluster (if it has a name, Karpathy didn’t share it with the audience) sports 720 nodes, each powered by eight of Nvidia’s A100 GPUs (the 80GB model), for a whopping 5,760 A100s throughout the system. This accelerator firepower is complemented by ten petabytes of “hot tier” NVMe storage, which has a transfer rate of 1.6 terabytes per second. Karpathy said that this “incredibly fast storage” constitutes “one of the world’s fastest filesystems.”
“So this is a massive supercomputer,” Karpathy said. “I actually believe that in terms of flops this is roughly the number five supercomputer in the world, so it’s actually a fairly significant computer here.”
Some back-of-the-envelope flops math seems to bear out Karpathy’s remarkable claim. According to Nvidia’s marketing materials, each A100 is capable of 9.7 peak teraflops, but in benchmarking for systems like the Selene supercomputer, eight-A100 nodes each deliver around 113.3 Linpack teraflops (~14.2 Linpack teraflops per GPU, inclusive of accompanying processors). 720 eight-A100 nodes later, you get around 81.6 Linpack petaflops — enough to place the Tesla cluster well above the aforementioned Selene system, operated by Nvidia, which delivers 63.5 Linpack petaflops and placed fifth on the most recent Top500 list. (The Top500 often does not include corporate systems like Tesla’s due to trade secrecy, and the list is due to be refreshed at ISC21 this coming week.)
This cluster – and, eventually, Dojo – are being deployed in service of Tesla’s feverish push for the next generation of vehicle automation: full self-driving (FSD) vehicles. In the talk, Karpathy discussed why the electric vehicle juggernaut is moving toward FSD and how its clusters – including the new one – serve that ambition.
One of Karpathy’s first slides was particularly telling: a poorly-Photoshopped brain in the driver’s seat of a zooming car, captioned with statistics characterizing humans as meat computers with a “250 ms reaction latency” in a “tight control loop with one-ton objects at 80 miles per hour.” For Tesla, FSD is about replacing that sluggish computer (which Karpathy noted could write poetry, but often had trouble staying within the lines on the road) with a faster, safer one.
But training computers to understand roads – even with cameras and lidar on-board – is difficult, involving innumerable contingencies and bizarre scenarios that impede the vehicle’s ability to process its surroundings in a traditional manner. In one example, Karpathy showed a truck kicking up dust and debris that obscured the cameras, effectively blinding the vehicle for several seconds.
In order to train systems that can cope with these obstacles, Tesla first collects mountains of data. “For us, computer vision is the bread and butter of what we do and what enables the autopilot,” Karpathy said. “And for that to work really well, you need a massive dataset – we get that from the fleet.” And, indeed, the dataset is massive: one million ten-second videos from each of the eight cameras on the sampled Teslas, each running at 36 frames per second and capturing “highly diverse scenarios.” These videos contain six billion object labels (including accurate depth and velocity data) and total 1.5 petabytes.
“You … need to train massive neural nets and experiment a lot,” Karpathy said. “Training this neural network – like I mentioned, this is a 1.5 petabyte dataset – requires a huge amount of compute.” Accordingly, he said, Tesla “invested a lot” into this capability. In particular, Karpathy explained, the newly unveiled cluster is optimized for rapid video transfer and processing, thanks to that aforementioned “incredibly fast storage” and “a very efficient fabric” that enables distributed training across the nodes.
Dojo, for its part, is still being teased. “We’re currently working on Project Dojo, which will take this to the next level,” Karpathy said. “But I’m not ready to reveal any more details about that at this point.” Little is known about the mysterious forthcoming system beyond a handful of tweets by Musk referencing the exaflop target, claiming that “Dojo uses our own chips [and] a computer architecture optimized for neural net training, not a GPU cluster” and sharing that Dojo will be available as a web service for model training “once we work out the bugs.”
“Could be wrong,” Musk tweeted, “but I think it will be best in the world.”
For now, though, Tesla is content to let the world know that it’s betting big on HPC – and that the bets are only getting bigger. Karpathy said that the HPC team is “growing a lot,” and encouraged audience members who were excited by HPC applications in self-driving cars to reach out to the company.