JPMorgan Chase AI Head: Here Are the Next Steps for Enterprises to Accelerate Their AI Deployments
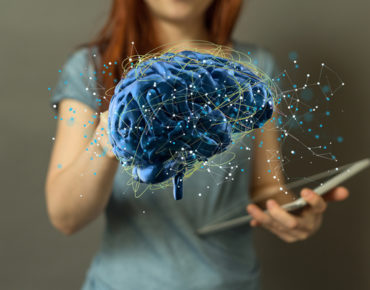
AI usage inside enterprises often starts out slowly, from conceptual stages to pilots to testing and eventual deployment. But as those ground-breaking projects mature and more AI uses are discovered and encouraged, companies must constantly re-evaluate their strategies and look to accelerate their AI deployments to keep pace and aim for bigger goals.
That’s the advice of Daniel Wu, the head of commercial banking AI and machine learning at JPMorgan Chase, who gave a presentation at the virtual AI Hardware Summit on Sept. 15 about “Mapping the AI Acceleration Landscape.”
The rapid rise and expansion of AI across industries in recent years has been fueled by vast improvements in compute power, open and flexible software, powerful algorithms and related advances, but enterprises should not stand pat on early AI successes, said Wu.
Instead, as more experiences are gained, it is a good time to accelerate those efforts and democratize further AI innovations to help companies use these still-developing tools to drive their business goals and strategies, he said.
To further drive AI capabilities, enterprises need to start with the basics they already know, said Wu, including data, hardware, IT staffing, governance and operations.
“It is my intention to attempt to address this very daunting question about how we should go about building AI capability, regardless of the size of an organization and the types of resources you have for your organization,” said Wu. “Data, obviously, first comes to our attention,” with the biggest pain point for data scientists and machine learning practitioners being dirty data.
“About 60 percent of developers think that dirty data is a major problem for them, with around 30 percent of data scientists saying that the [adequate] availability of usable data is a major roadblock for them,” said Wu. “So, what can we learn about this?”
Such challenges with data are not new and have been around for a long time, he said. “You see data silos everywhere, cross-functional domains, with each team developing their own solution and creating their own data assets without thinking about how that data asset can be used across the organization.”
For many IT systems that were developed many years ago, proper data models were not included in their creation, he said. At the time, functional and performance requirements were all that mattered, with little regard about the future use of the data for other needs.
But AI has changed that old approach, said Wu.
“Even today, a lot of companies are going through digital transformations, and they are bringing their on-premises data centers into the cloud,” he said. “During this transition, there is this hybrid, awkward state where you have some part of the data sitting in the cloud and some part of the data sitting on-premises in your own private data center. Most of the time, that creates unnecessary duplication.”
To tackle this and better prepare that data for AI use today, one strategy is investing in data cleansing, which is a one-time, upfront cost to clean up the data and consolidate it. “You try to get to that single source of truth, so when data comes to the data scientists, they do not have to battle between which data should they believe in,” said Wu.
To ensure this works better in the future, enterprises should practice data-centric design, where data should be top of mind from the outset as part of every process and technology, he said. “It should not be a second-class citizen. We should automate data processes. A lot of organizations still have many manual steps to execute certain steps, or scripts, to do their ETL (extract, transform, load). And part of that automation is to make sure that you incorporate the data governance and cataloguing in your process to make that an integral process.”
Data also must be made more accessible to further drive AI use, said Wu.
“Part of that can be enabled by building some self-service tools for organizations, for data workers, so they can get to the data more easily,” he said. “Reusability is it should also be highlighted here. And we need to break down the silos.”
These steps will also help enterprises save a lot of time in their model development processes, he added. “Think in terms of a feature store, which is a very popular trend now floating around, these reusable features that you can use to build multiple solutions.”
Changes for accelerating AI are also needed when it comes to compute, said Wu.
“The challenges here are still around availability, cost and efficiency of compute, but we also need to pay attention to the carbon footprint,” he said. “People are not guessing how much of a carbon footprint there is as we train these large models. But there is hope in leveraging them.”
Another trend being seen is that some users are moving from very general computer architectures to more domain specific architectures, both in cloud deployments as well as in edge deployments, he said.
Wu also has new ideas when it comes to creating more language models.
“There is no need for every organization to co-develop another large language model,” said Wu. “We should move towards leveraging what has been developed and only require some refinement and tuning of the model to make it serve a different business use case.”
Instead, enterprises can look to leverage a layered AI model architecture that is more generic for most uses, while allowing the creation of more specific models to meet specialized business cases, he said.
Overall, however, it will take more than data, compute and modeling to drive faster AI acceleration, said Wu.
It also requires skilled, trained and imaginative IT workers who can bring their innovations to AI to help drive their company’s missions, he said.
“We all know about the shortage of AI talent around the globe,” he said, but there is also an imbalanced distribution of talent that is exacerbating the problem. About 50 percent of the nation’s AI talent is in Silicon Valley, with about 20 percent of those workers employed by the largest technology companies. That does not leave enough experts available for other companies that want to drive their AI technologies, he said.
“This is a real challenge that we have to address across the community,” said Wu. To combat the problem, enterprises must find ways to reduce the burdens on their AI team by ensuring they focus on developing models, and not dealing with other IT overhead in their organization, he added.
“Thirty-eight percent of organizations spend more than 50 percent of their data scientists’ time in doing operations, specifically deploying their models,” he said. “And only 11 percent of organization can put a model into production within a week. Some 64 percent take a month or longer to do that production integration, with a model fully trained, validated and tested. Going to the finish line would take over a month most for most organizations.”
These delays and distracting activities happen because AI operation support is not there, said Wu. “We need to move to build out the machine learning operation capabilities and move from model-centric to data-centric ideas. Think about how much lift you can get easily from getting better data to train your models, rather than focusing on inventing yet another more powerful model architecture itself.”
An important step to control these issues is to recognize the importance of change management, while also maintaining a clear lineage from your data to your model so that you can have or increase the ability to reproduce your model, he added.
Ultimately, even after an organization’s models are developed and deployed, there are still worries that will keep IT leaders awake at night, said Wu. “Now they are thinking, ‘oh, my God, what is the risk of deploying the solution and making it available to the customers? This becomes the afterthought, and this usually becomes the biggest blocker at the end and prevents the solution going out to general availability.”
The challenge for enterprises is to try not to only think about time to market, said Wu. “You also need to think about doing things right, so you do not end up going back to the drawing board and have to redevelop your entire solution. That will be much more costly. And meet regulatory requirements – there are a lot of ethics around AI development that organizations must address early to mitigate these risks. Implement a process to guide your model development lifecycle and incorporate and streamline your compliance practice to this cycle.”
In another presentation at the AI Hardware Summit, Aart De Geus, the chairman and co-CEO of electronic design automation (EDA) and semiconductor IP design firm, Synopsys, spoke about how Moore’s law continues to be stretched to its limits in recent years and might be better replaced by the concept of “SysMoore,” a blending of long-held Moore’s law insights with new technology innovations that leverage systemic complexity.