Shortage of Chips Hurts AI, But Problems Go Deeper
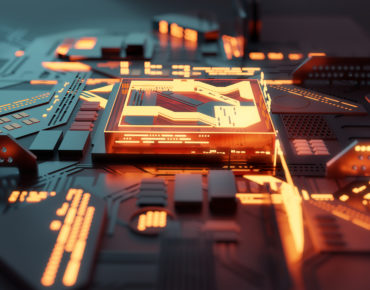
The impacts of the chip shortage can be spotted everywhere: from higher prices on home appliances to empty new car lots to higher prices for consumer devices. Processor-hungry AI applications are feeling the pinch, but according to SambaNova’s CEO, hardware alone isn’t a determining factor in AI’s success.
The roots of the current bottleneck in the semiconductor supply chain have lurking for years, but it took the COVID-19 pandemic to bring the problems to the surface. In a prescient story from February 2020, Semiconductor Engineering warned that demand was close to outpacing supply for chip fab equipment for 200mm wafers, which are typically used for older, slower chips (with newer, faster chips being made from 300mm wafers and gear).
A surge in demand for Internet of Things (IoT) devices, which don’t require the fastest chips and can be made from 200mm wafers, is commonly cited as an underlying cause for the chip imbalance. Then came COVID-19, which temporarily lowered demand and worker supply before unleashing an explosion in need for chips of all sizes. Lockdowns and social distancing requirements in chip fabs in China and Southeast Asia have not helped.
Chip production appears to be falling further behind. In the third quarter of 2021, the lead time between when a chip order was placed and when the chip was delivered reached 22 weeks, nearly double the lead time at the end of 2020, when it was about 13 weeks, according to a Wall Street Journal article last week that cited Christopher Rolland, an analyst with the quantitative analyst firm Susquehanna.
That is the highest lead time Rolland has seen since he started monitoring the industry in 2013. The lead time for other specific types of chips, including the microcontrollers used in cars, is even greater: 32 weeks, or just under eight months. This is the underlying factor driving the 21 percent increase in used car prices over the past year, a figure that comes from CNBC.
The global chip shortage is also starting to impact AI workloads. Many AI models, such as computer vision and NLP, rely on powerful GPUs for model training. While chip fabs are struggling to produce older, slower chips, there is also a shortage of high-end chips like GPUs.
“A lot of GPU users are complaining that it’s hard for them to get the GPU time” on public clouds, Gigamon AI analyst Anand Joshi told Datanami earlier this year. “They put a job in a queue and it takes a while for it to ramp.”
Jensen Huang, the CEO of GPU giant Nvidia, which relies on Asian chip fabs to produce its GPUs, also warned that the company was facing limitations in its supply chain. “I would expect that we will see a supply-constrained environment for the vast majority of next year is my guess at the moment,” Huang said during a call with analysts on August 15, after the company recorded its financial results. “But a lot of that has to do with the fact that our demand is just too great.”
To be sure, COVID-19 has been a unique occurrence, in that it is actually accelerating companies’ digital transformation strategies and driving demand for AI applications faster than before the pandemic. This is occurring amid a renaissance in novel chip designs, which are targeting emerging AI workloads as well as analytics workloads.
One of those chipmakers is SambaNova Systems, a four-year-old AI startup that’s already valued at over $5 billion. The company has contracted with TSMC to manufacturer its Reconfigurable Dataflow Unit (RDU) with a 7nm process. The RDUs are sold in pre-configured racks, or available as an API via the software-as-a-service delivery method.
But fast hardware is only part of the AI equation for SambaNova, according to Rodrigo Liang, the company’s CEO and co-founder. There are lots of other factors that go into developing a successful AI solution, he says.
“The chip shortage has accelerated the industry’s focus on compute efficiency. End users are gravitating towards AI-specific solutions rather than commodity components to attain the benefit of this efficiency,” Liang tells Datanami via email.
“AI is a software problem that cannot be solved with chips alone,” he continues. “To successfully take AI projects into production, end-users must be able to integrate AI into their workflows and applications. Accumulating individual components such as chips and self-integrating the solution is a time-consuming and expensive endeavor that requires significant skills and expertise that most organizations lack.
Beyond the chip shortage, an equally important issue is the AI talent shortage, Liang says. “Currently, the majority of AI talent sits within the largest tech companies,” he says. “To enable more universal AI equity, industry must deliver their products with high ease-of-use to limit the end-user expertise required to implement and scale AI.”
To be sure, we live in interesting times. The combination of an explosion of IoT devices, work-from-home mandates and worker shortages in the chip fabs themselves have combined to drive demand for chips beyond the available supply.
However, as the COVID-19 pandemic has demonstrated time and time again, a crisis can also be an opportunity. If processing power is constrained, innovative companies will find a workaround for that constraint. Already, companies have committed to spending hundreds of billions of dollars to build new chip fabs. The constraint eventually will be eased, which will unleash even more innovation in the years to come.
This story was originally featured on sister website Datanami.
Related
Alex Woodie has written about IT as a technology journalist for more than a decade. He brings extensive experience from the IBM midrange marketplace, including topics such as servers, ERP applications, programming, databases, security, high availability, storage, business intelligence, cloud, and mobile enablement. He resides in the San Diego area.