Building and Implementing Responsible AI: A Practical Guide
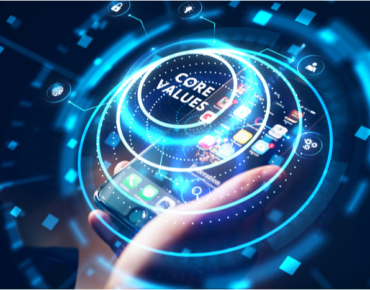
Artificial intelligence can help companies solve complex business challenges and identify new opportunities — but businesses need to be able to trust the technology. Currently, only 25 percent of businesses have processes fully enabled by AI and only 20 percent of companies have an ethical AI framework. This can create serious business consequences.
In today’s business environment, however, AI is vital. Retailers, for example, are facing a hectic holiday season and AI can create better online experiences at speed. AI increases productivity, product quality and consumption. By automating tedious tasks, AI helps companies alleviate the burdens facing IT teams that are holding down the fort during these unprecedented times.
Despite all the benefits that come with innovation, teams need a responsible AI framework in place and a toolkit before they start using AI. AI as a technology is neutral — it is not inherently ethical or unethical. Instead, AI is a system that conforms to the norms and standards of society. It is critical to evaluate what controls, requirements or standards are or should be in place to achieve this.
What are some steps that can be taken to make this happen?
- Catalog AI’s Impact on Systems
An important part of creating a responsible AI framework is to catalog its use inside your company. AI, especially in the form of recommendation engines, chatbots, customer segmentation models, pricing engines and anomaly detection are becoming pervasive in the enterprise. Keeping track of these AI models and the systems or applications where they are embedded is critical to ensure that your organization is not exposed to operational, reputational and financial risks.
You also need to know how your models will be used and what potential harms – physical, emotional or financial – that they could cause. Understanding these harms and risks will help you to embed AI ethics before you build or deploy a model.
Ideally, you will understand what systems the AI will impact before you start development or deployment. However, if you already have AI in place, you need to catalog this knowledge gap.
To instill trust in AI systems, people need to be able to look under the hood in the underlying models, analyze how the AI was built, explore the data used to train it, expose the reasoning behind each decision and provide coherent explanations to all stakeholders in a timely manner. There is a tradeoff between accuracy, explainability, fairness and security for each AI system. Being able to justify these tradeoffs internally to the different stakeholders, to your customers and to regulators is critical to gaining trust.
Cataloging what you have makes it possible to tune AI systems to mitigate bias, which is supported through a governance process. It is important that your AI – like every employee – adheres to your organization’s corporate code of ethics.
- Standardize Your AI Development Lifecycle
It is important to have a standardized process of managing your data, building an AI model and embedding it into an application system. Once the AI model is deployed in a production system you also need a standard process to monitor its performance and continue to refine and retrain it as required.
This standardized process typically involves scoping the AI model to build it based on the business requirements and the data available. This leads to the design of the model and how it will be used within a larger application system. This design phase is followed by data exploration and model build. Once the model has been trained, tested and found to meet the acceptance criteria, it is ready for deployment. One needs to monitor the performance of the model on an ongoing basis and ensure that the model is retrained, refined or retired as necessary.
As part of this AI development lifecycle, you must maintain datasheets for the data sets as well as model cards. The datasheets will capture important data items and outline the motivation for gathering the data, the collection process, recommended uses and more. It is also a good practice to have model cards. These cards include details on the AI model, the chosen algorithm, the intended use of the model, ethical considerations and more. Social impact and risk assessment must also undergo reviews. These tools help to shape more informed decisions on whether the algorithm should be adopted.
To evaluate the deployment of the model, you need to start with agreed upon success and acceptance criteria. These should look at the performance of the model as well as interpretability, explainability, fairness, safety, control, security, privacy, robustness and reproducibility of the model. If those success and acceptance criteria are not met in the deployment, the AI should not be deployed in its current state. Some businesses will still move forward, relaxing the threshold. However, this is only for exceptional cases. Data scientists should not make this decision – it should be up to the business sponsor or product owner.
Because AI systems learn to make conclusions based on training data, evaluating the impact of an application over the course of its development can help identify areas for improvement that can prevent risks.
- Create a Governance Process
Creating a governance process helps ensure that teams are addressing specific issues related to bias and fairness in AI systems before they are deployed. It empowers teams to think critically and be able to answer questions about the decision-making of AI applications.
If done correctly, a successful governance process will provide guidance and reassurance. It will equip teams to assess whether systems in place align with their business strategy and to encourage accountability and compliance.
For any team to uncover the full promise of AI, they must analyze the systems the AI is using, evaluate the impact, and create a governance process. In today’s increasingly transparent, fast-moving, and competitive marketplaces, implementing ethical and responsible AI is not just nice to have, but is a prerequisite for success.
About the Author
Anand Rao is the global and U.S. artificial intelligence and U.S. data and analytics leader for PwC’s U.S. advisory practice. He has more than 24 years of industry and consulting experience, helping senior executives structure, solve and manage critical issues facing their organizations. He has worked extensively on business, technology, and analytics issues across a wide range of industry sectors including financial services, healthcare, telecommunications, aerospace and defense across the U.S., Europe, Asia and Australia. Before his consulting careers, Rao was the chief research scientist at the Australian Artificial Intelligence Institute, a boutique research and software house.