Graphcore Announces Wafer-on-Wafer IPU, ‘Good’ Computer
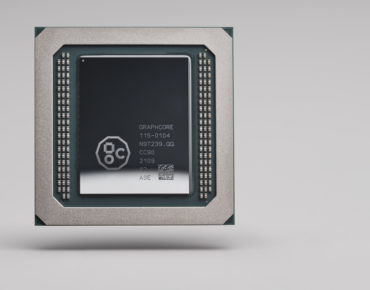
Graphcore introduced its AI-focused, PCIe-based Intelligent Processing Units (IPUs) six years ago. Since then, the company has done anything but slow down, announcing a second generation of IPUs in 2020 and, over the years, larger and larger IPU-based “IPU-POD” systems — most recently the IPU-POD128 and the IPU-POD256, both announced just a few months ago. Now, Graphcore is (quite literally) taking things to the next level, introducing its two-layer, wafer-on-wafer, third-generation IPU. Called “Bow,” the processor — which is shipping now — offers substantial improvements in performance and power efficiency over its predecessor. The company also announced plans for a massive system based on a forthcoming generation of its IPUs, which it is calling the Good computer.
Bow, WoW
The Bow IPU — so named after a London district, the new Graphcore naming convention — was manufactured using a new variant of TSMC’s 7nm process that enables wafer-on-wafer (WoW) packaging. “Wafer-on-wafer is a different technology to the chip-on-wafer vertical stacking that you might have seen, for example, with AMD’s Milan-X [which stacks] L3 cache on top of the processor,” explained Simon Knowles, co-founder, CTO and executive vice president for engineering at Graphcore. “Wafer-on-wafer is a more sophisticated technology. What it delivers is a much higher interconnect density between dies that are stacked on top of each other. As its name implies, it involves bonding wafers together before they are sawn. So two wafers are connected together — or in the future, more than two wafers — and then they are singulated into separate silicon chips.”
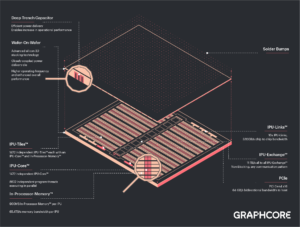
Click to view: a detailed diagram of Graphcore's WoW structure for the Bow IPU. Image courtesy of Graphcore.
For Bow, Graphcore and TSMC attached a second wafer to the processor wafer, with the second wafer carrying a large number of deep-trench capacitor cells that allowed smoother power delivery to the device — which, in turn, enabled the processor to run faster and at a higher voltage. “This is just the first step for us,” Knowles said. “We have been working with TSMC to master this technology. We use it, initially, to build a better power supply for our processor — but it will go much further than that in the near future.”
Graphcore lauded TSMC, which, they said, had been working with them for 18 months on the Bow IPU. Graphcore is the first company to deliver wafer-on-wafer technology in a production product.
Thanks to the improved power delivery, Bow boasts up to a 40 percent improvement over its predecessor across major AI workloads, ranging from a 29 percent improvement at the low end of things (for an object detection workload) to a 39 percent improvement on the higher end for various NLP and image classification workloads. The Bow IPU is also up to 16 percent more power efficient.
Bow is, otherwise, relatively similar to the previous-gen IPU. “It has the same nearly 1GB of static RAM on the chip as the previous device, but now 40 percent faster access — so 65 terabytes-per-second access to nearly a gigabyte of on-chip memory,” Knowles said. “It has the same 1,472 independent processor cores, each capable of running six independent programs. … And finally, it has the same 10 IPU links to connect chips together, delivering, in total, 320 gigabytes per second of inter-chip bandwidth.”
The Bow IPU offers 350 peak teraflops of mixed-precision AI compute, or 87.5 peak single-precision teraflops. Graphcore noted that this compares favorably on paper to the listed peak for an Nvidia A100 (19.5 peak teraflops FP32), but real-world performance comparisons will, of course, be interesting to see.
IPU Machines & Bow Pods
Similarly to previous generations of Graphcore’s IPU, Bow gets packed (4×) into Bow-2000 IPU Machines, which offer 1.4 peak petaflops of AI compute (350 peak teraflops FP32). The Bow-2000s are then packed into Bow Pods of varying sizes, ranging from the Bow Pod16 (4× Bow-2000, 1.4 peak petaflops FP32) to the unprecedented Bow Pod1024 (256× Bow-2000, 89.6 peak petaflops FP32), which is currently in early access. (Graphcore also offers Pod32, Pod64 and Pod256 sizes.)
“These products all exist today and we are shipping to customers today,” said Nigel Toon, co-founder and CEO of Graphcore. Further, he said, there would be no increase in cost. (“We may choose to reduce the cost of [previous] systems — we haven’t made that announcement yet.”)
Toon compared the Bow Pod-16 (which he said retails for “just shy of $150,000”) to an Nvidia DGX A100 that retails for “just under $300,000.” The Bow Pod, he said, took 14 hours to train an efficient image classification model versus 17 hours on the Nvidia system. “That’s five times faster to train on a system that costs half the price.”
All of this, Toon said, comes without painful adjustments for developers accustomed to Graphcore’s preceding products. “There are no code changes,” he assured. “So all of our existing models, all of our customers’ models that they built using our Poplar software environment, work seamlessly out of the box.”
And Graphcore has assembled an impressive list of customers for its third-generation products. The star of the show is Pacific Northwest National Laboratory (PNNL), which Graphcore says will be using these IPUs to help develop transformer-based and graph neural network models for computational chemistry and cybersecurity.
“At Pacific Northwest National Laboratory, we are pushing the boundaries of machine learning and graph neural networks to tackle scientific problems that have been intractable with existing technology,” said Sutanay Choudhury, co-director of PNNL's Computational and Theoretical Chemistry Institute. “For instance, we are pursuing applications in computational chemistry and cybersecurity applications. This year, Graphcore systems have allowed us to significantly reduce both training and inference times from days to hours for these applications. This speed up shows promise in helping us incorporate the tools of machine learning into our research mission in meaningful ways. We look forward to extending our collaboration with this newest generation technology.”
Other major customers include Sandia National Laboratories, Imperial College London, the University of Massachusetts Amherst, the University of Oxford, Stanford Medicine and more — many of which the company said it could not name due to confidentiality.
The Good computer
But Graphcore isn’t stopping there. “When we started Graphcore, we talked about building the ‘Intelligence Processing Unit,’ so the idea has always been in the back of our mind to build an ultra-intelligence machine that would surpass the capability of a human brain — and that is what we are now working on,” Knowles said.
He clarified that they don’t know exactly what will be necessary for that dramatic goal — but that they can make some guesses. The human brain, he said, had around 100 billion neurons, plus axons with hundreds of trillions of synaptic weights; by comparison, today’s largest neural network models have around a trillion parameters.
“So we clearly have another two or three orders of magnitude to go before we might build a machine with the information capacity that clearly exceeds the human brain and therefore potentially unlocks ultra-intelligent AI,” he said. “Graphcore is intending — in fact, is on the path — to build such a machine.”
“This machine will contain 8,192 IPUs of a generation beyond the Bow processor, but further leveraging 3D wafer-on-wafer stacking technology,” he said, adding that the machine will deliver “over 10 exaflops” of floating-point performance and 4PB of memory, accessible at more than 10PB per second. “This will allow AI models to be hosted which are many hundreds of trillions of parameters.” (Graphcore specifically cites a goal of 500 trillion parameters.)
Knowles said that the Good computer is named after Jack Good, a 1960s computer scientist who “talked about the concept of an ultra-intelligence computer.” They clarified that the Good computer — which they expect to cost $120 million — will be a product intended for sale to multiple customers, not a one-off system.