Can We Trust AI — and Is That Even the Right Question?
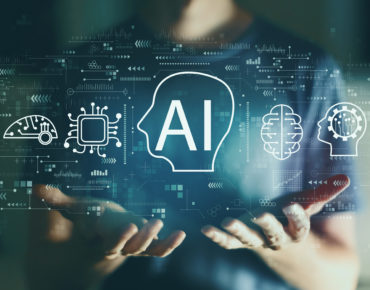
AI is becoming more ubiquitous, from everyday voice assistants and online shopping to healthcare and workplace management — but can we trust it? That question was the headline of a panel called “Can We Trust AI?” during the Discover Experiential AI event at Northeastern University’s Institute for Experiential AI (EAI) this week.
The panel was moderated by Ricardo Baeza-Yates, a professor at Northeastern’s Khoury College of Computer Sciences and director of research for EAI. It featured four guests:
- Silvio Amir, an assistant professor at Khory College and a core member of EAI
- Cansu Canca, founder and director of the AI Ethics Lab and head of AI ethics for EAI
- Rayid Ghani, a professor of machine learning and public policy at Carnegie Mellon University
- Lorena Jaume-Palasí, founder and executive director of the Ethical Tech Society
Where AI Is Unwelcome
“A lot of people in the [previous sessions in the] morning have been talking about stakeholders, and the company, and the risk, and the HR people, and the marketing people,” said Ghani toward the beginning of the panel. “And in my mind, the most important stakeholder is the person who gets affected by these systems. It’s the person on the street that’s not here.”
Much of the panel centered around how those stakeholders were treated, how they differed from one another (both culturally and as individuals) — and whether AI could make those important distinctions. “I think a lot of the time the way we think about it in relation to AI is, ‘what types of AI should we create?’ or ‘how should society perceive AI systems?’” Canca said. “But I think perhaps the more interesting thing to think about is: how does the AI eventually see us?”

From left to right, top to bottom: Silvio Amir; Ricardo Baeza-Yates and Rayid Ghani; Cansu Canca; and Lorena Jaume-Palasí.
But the conversation really kicked in when Baeza-Yates asked each participant to name a problem for which AI should not be used.
“I was in a program committee for a conference,” recalled Amir, “and I was assigned a paper which was proposing models to detect whether someone is gay or not. And this is one example of something that I cannot really see a positive or beneficial utility for this type of model, but I can see plenty of opportunities for abuse and nefarious outcomes.”
Ghani disagreed, saying that he often posed questions like this to students, asking them whether certain AI models would be unethical. “Nobody is saying what you’re gonna do with it, right?” he said. “So the act of predicting if somebody is gay has, in my mind, neither positive nor negative ethical consequences. It’s the intervention — it’s the next step, right?”
He offered a hypothetical use case where a support organization was looking to predict traits to preemptively help people to avoid harassment. “And if it doesn’t get used for anything else and it’s guaranteed that nobody else gets access to it, I can see the value,” he said. “I think any argument we have on these topics is about the intervention. The ethics are coming from the intervention and the action, and the ethics aren’t coming from the analysis.” (“You can just ask people: do you need help? Are you part of this population?” Amir countered.)
Canca offered another example. “I dealt with a project that was trying to make a much stronger and [more] integrated Fitbit-style type of thing — so, a system that analyzes you at all times and connected with everything else, all your social media, to understand you really well, with the purpose of coaching you to lead a good life. And I think the idea of an AI system just looking at the data available for you and leading you to lead a good life is… extremely problematic.”
The Importance of the Individual
Jaume-Palasí honed in on a key issue: trying to predict or assess individual characteristics from amalgamated data and group trends. “It’s simply not scientific,” she said, comparing AI applications like predicting sexuality to scientifically shunned or discarded practices like eugenics or phrenology.
“Let’s say, for instance, in recidivism, when you have to decide as a judge whether you give someone parole, which is a situation where it’s not dependent on any other prisoner or any other contingent,” she said. “I would never use it there! Because you are not evaluating, individually, the person with their own circumstances — but you are [instead] trying to understand in a generic way how this person is similar to others — or how this person is similar to people who suffered a specific decision in the past from a judge.”
Jaume-Palasí similarly cited applications in human resources and recruiting processes, saying that the use of predictive AI in these kinds of processes were beginning to be prohibited in some parts of Germany.
“I would not do anything with AI where humans are doing it perfectly today and we can’t make it any better,” Ghani said. “Judges make decisions about recidivism every single minute. Do we think they’re so good, and so not racist, and so not sexist that nothing can improve on them? Probably not! … If we can improve people’s lives and we can do it in a way that achieves our value systems, and that combines humans and AI in a way that’s nuanced … I think that’s where we need to think about it, rather than say, ‘machines should never make predictions about somebody going to jail.’”
Baeza-Yates chimed in. “If noise cancellation works 99% of the time, would you use it? Yes, I would,” he said, referencing an earlier presentation from Bose. “No harm if it doesn’t work. But if the elevator that you took today works 99% of the time… would you have taken it? I want to know!”
Then, he asked the question of the hour: can we trust AI?
“Whether we should or [should] not trust AI — I do not think that that’s the most important question,” Amir said. “I think that the question is how we can build AI that is trustworthy, right? And I say this because AI does not arise spontaneously out of thin air, it does not have a personal agenda — instead, AI is developed by individuals with goals and motivations, and we, as practitioners … get to decide which models to build, which functions to optimize for, which data to use, how to process, filter, and curate this data… and all of these choices will have a direct impact on how trustworthy our systems are.”
And right now, he suggested, they are not built to be as trustworthy as they could be. “The models that we use today … they do indeed learn how to replicate and even exacerbate biases that they find in the data,” he said.
“I don’t think trust is the right term to use in relation to AI,” agreed Canca. Instead, she suggested, it was useful to think in terms of whether AI could be safely relied upon — to evaluate “whether the AI is working in the way that we want it to work, and also does it reduce harm, does it duplicate the biases, and all of these things. … Talking about it in terms of trust, I think, convolutes where the responsibility lies.”
“To err is human,” concluded Baeza-Yates as the panel ended, “but not for machines.”