Key Strategies Enterprises Can Implement Now for Success with AI
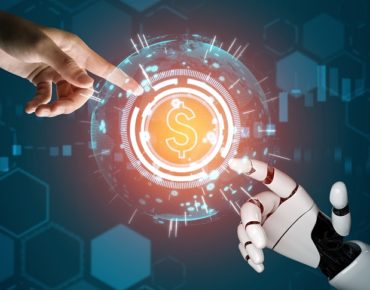
According to the latest “Voice of the Enterprise” survey conducted by 451 Research, 34% of machine learning (ML) projects fail and don’t get to production. And a previous Gartner report that found 47% of machine learning projects fail to go into production. What these statistics show is that there’s still a long way to go when it comes to ML projects.
451 Research’s survey also revealed that it takes an average of 12 weeks to bring a machine learning project from conception to production. While that may seem like a long time, in most cases, it’s well worth it. However, the long timeframe also creates frustration for businesses and even worse, what often happens is that many models simply sit in the lab.
So, how do you effectively get your AI model into production?
Establish a business use case
What does your business need a machine learning model for? What problem are you trying to solve? These are the foundational questions. Once they’re answered, proceed to the other important considerations. For instance, all models have an expiration date, and you don't know what that expiration date is. You just know that you're going to have to update it. So, when you're building the business case, keep in mind that you’ll have to know how much benefit you can get by improving the model, but also how much it is going to cost to do the integration and deployment at scale, and to monitor the model effectively.
In addition, keep seasonality in mind. Some companies peak during the holidays, some during summer, some on weekdays vs. weekends and so on. You need a system that can dynamically adapt to different use cases.
Speaking of use cases, you may have a model today for one business use case, but soon it will expand to another business unit. So, if best practices are initially ignored, it's going to become increasingly difficult to scale. In the situation where use cases have expanded rapidly, there needs to be governance on the development side as well, which can be new to data science teams. You will need strong version control, strong code, reviews and the establishment of a baseline.
Much of what machine learning models are doing is optimizing decisions. That’s why you must know what the baseline is. If you leave this to humans, for example, what is the error rate? What is the customer experience if humans make this decision? Then you monitor the model and track its performance. Having that strong baseline enables you to know what’s working and what to improve on.
Synchronize your data science, DevOps and engineering teams
When going into a real production environment, there needs to be a cross-collaboration across all teams. It's extremely important that you find a seamless way of integrating with the existing DevOps tools that these organizations are using, because they are the foundation of the model. Without the synchronization, key elements such as scalability and reliability will suffer, rendering your model useless.
Production is typically owned by the engineering organization or the DevOps organization, so they carry a lot of influence, and the team must make sure that the tools that need to be set up around this model are in alignment with their thought process and understanding.
The key to success is strong product teams. It takes a village to get an AI and machine learning model into production and integrated into a larger ecosystem. The NetOps team, engineering team, information security and stakeholders are all involved in the project. Having everyone aligned to the same objective is crucial.
Your existing DevOps software tools don’t apply to your AI model
A model is not the same as software; the behavior of the software doesn't change based on the user, for example. But with a model, the prediction will change depending upon the type of the request that comes in.
If the DevOps software tools don’t apply to your model, this creates too much friction between the data science teams and the engineering teams. Best practices need to be adopted early on in the production phase because it's too difficult to change later on.
Data management features, data quality and data integration are the most sought-after features of an MLOps platform. Additionally, an MLOps platform must provide a dynamic adjustment upon infrastructure so that it's not managed manually but is automated like cruise control and put in the backend.
Data fuels and changes the model, and it needs to be updated. Before the concept of MLOps came along, there used to be many frustrated data scientists spending too much time on the data management aspects of their job. Though MLOps is predominantly focused on post-deployment, the need for these features is expanding.
Don’t forget to implement model governance
When models make decisions, they make them on a large scale and there are many things that may or may not go wrong. This is why governance across the organization is key, particularly to the executives, when these models are making decisions. Asking questions like, “Does this model pose any risk to the organization?” and “Are the proper guidelines being followed by the various teams?”
Governance provides transparency across the organization on how AI models are using and ensures that executives have peace of mind that yes, these models are making money and making decisions within acceptable risk parameters. And if risk is beyond a particular point, they need to have the power to investigate these issues and understand why they are occurring.
Business results from AI
There’s still some lingering belief out there that AI/ML is overhyped or that it can’t produce any meaningful return-on-investment. Another pervasive myth is that AI is too expensive and is accessible only to the industries’ biggest players. But advances in these technologies are rapidly exposing these myths for what they are. With the right practices in place, it’s possible for many enterprises to get AI models up and running and delivering business value quickly. Don’t let your project become part of the percentage of companies whose models fail by following the practices outlined above.
Victor Thu is president of Datatron. Throughout his career, Victor has specialized in product marketing, go-to-market and product management in C-level and director positions for companies such as Petuum, VMware and Citrix.