New ML Method Investigates Promising Solar Materials
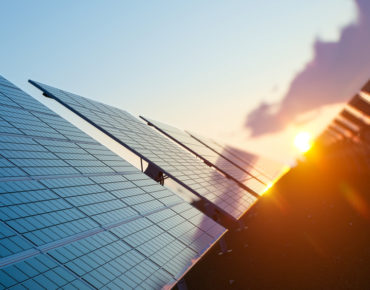
Over the past fifty years, solar power has risen from a curiosity and future prospect to a truly viable utility-scale energy source that provides nearly three percent of U.S. electricity. Growing solar’s energy share, though, necessitates continual innovations in materials science, manufacturing, grid operation and efficiency. Now, a pair of researchers at Argonne National Laboratory have designed a new machine learning technique for investigating novel solar cell materials.
Typical modern-day solar panels use silicon or cadmium telluride — but typical solar panels are also quite expensive. The Argonne researchers — Maria Chan and Arun Mannodi-Kanakkithodi — sought to investigate halide perovskites, a popular subject of study for use in solar cells due to their low costs and desirable electrical properties.
But there’s a problem.
“Unlike silicon or cadmium telluride, the possible variations of halides combined with perovskites are essentially unlimited,” Chan said in an interview with Argonne’s Joseph Harmon. “There is thus an urgent need to develop a method that can narrow the promising candidates to a manageable number. To that end, machine learning is a perfect tool.”
Using their new method, the researchers trained it with hundreds of halide perovskite variations, teaching the model to understand the relationships between the composition choice and characteristics like the stability and solar absorptivity of the perovskite. Tested on a much larger database of 18,000 compositions, the researchers were able to identify 400 of particular interest.
“Our list of candidates has compounds that have already been studied, compounds that no one has ever studied, and even compounds that were not among the original 18,000,” said Chan. “So we are very excited about that.” Those materials will now undergo further experimental study in the real world — possibly using an AI-powered robotics laboratory like Argonne’s Polybot.
“We are truly in a new era of applying AI and high performance computing to materials discovery,” Chan said. Chan also stressed the importance of developing more efficient and cheaper solar cells: “According to a recent [Department of Energy] study, by 2035, solar energy could power 40 percent of the nation’s electricity. And it could help with decarbonizing the grid and provide many new jobs.”
The researchers also see applications outside of this area. “Besides solar cells, our design methodology could apply to LEDs and infrared sensors,” Chan said.
To learn more about this research, read the reporting from Argonne’s Joseph Harmon here and read the researcher paper, published in Energy & Environmental Science, here.