New ML Search Method Expands Horizon in Hunt for New Polymer Electrolytes
Jan. 18, 2023 -- Using computing resources at the National Energy Research Scientific Computing Center (NERSC), researchers at the Massachusetts Institute of Technology (MIT), in partnership with the Toyota Research Institute, have pioneered a new method for using machine learning to screen for new materials, yielding the largest dataset of polymer electrolytes ever seen in the field and signaling progress for the search for new materials generally. Their results were published in Nature Communications last year.
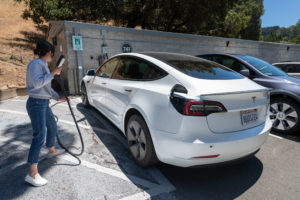
Most electric cars are powered by lithium-ion batteries, which rely on polymer electrolytes to conduct lithium ions and produce energy. Improved polymer electrolytes could improve battery performance in electric cars.
Lithium-ion batteries are one of the most-used types of rechargeable batteries in the world and power most current smartphones and electric cars. These batteries rely on electrolytes to conduct lithium ions from the anode to the cathode of a cell, producing energy. One class of electrolyte is the polymer electrolyte; however, the most commonly used class of polymer electrolytes has been in use since the 1980s, and scientists are on the hunt for new molecules that might be safer and more conductive.
“Today we use liquid electrolytes, but they can explode easily because they’re a flammable liquid,” said researcher Tian Xie, the primary author of the paper and a member of the Grossman group at MIT. “So if we could find a polymer electrolyte to replace the liquid, it would be much safer. But the best polymer we have so far still doesn’t conduct lithium ions fast enough. So the goal of this work is to use large-scale computational screening to find a new polymer that can beat the best material so far.”
Scientists increasingly use automated search to screen different molecules for useful properties based on molecular structure. However, the amorphous nature of these polymers makes them difficult and computationally expensive to screen. Because they don’t form clear, easily categorizable crystals, and because the simulations must run for a relatively long time to allow for the traveling of the lithium ion, the process can be prone to both random and systematic errors.
“It’s not only that the polymers have complex structures; it also needs to simulate for a really long time because the lithium ion transfers in this matrix and it takes a really long time,” said Xie. “So you’re dealing with a very big system of 5,000 to 10,000 atoms, and you also need to simulate 50 nanoseconds to get a converged polymer. So this is just such an expensive task; it’s almost impossible to simulate at a larger scale.”
This relatively accurate, less expensive way of screening combines two types of simulations. The first is just five nanoseconds, which is computationally inexpensive to run but also tends to overestimate the conductivity of the sample and contain a lot of errors. The second is a longer, 50-nanosecond simulation, which is long enough to allow the convergence of the lithium ion but is ten times more expensive to run. Xie and the team built a machine learning system that uses the first set of quick-and-dirty data to screen a large search space, then follows up with about 100 of the longer simulations to correct the errors from the first set. Using this method, which has been tested against real polymers to prove its accuracy, the team screened a space of about 6,000 polymers and can extrapolate their work to a total of 40,000 polymers. The research team believes it’s the largest molecular dynamics simulation for polymers ever performed at NERSC.
“All this relies on NERSC computing,” said Xie. His team ran their simulations on the Cori system at NERSC, using LAMMPS software scaled up from an existing molecular dynamics protocol, using the Fireworks workflow management system. “In terms of infrastructure, I feel that NERSC is one of the best-managed supercomputing centers in the U.S.—it’s easy to set up workflow systems there.”
The polymer electrolytes currently in use come from a class of molecules known as polyethylene oxides (PEOs) and were put into use in the 1980s. By computationally screening a significantly larger space than past studies, the team found a class of PEO-like polymers to have the highest conductivity, confirming that PEO remains the best option for now. However, Xie noted some limitations on the search space: all of the polymers screened in this study were synthesizable polymers at the same temperature and concentration, and did not search polymers containing a benzene ring. Searching a broader space of polymers at different concentrations might yield new polymer electrolytes that are overlooked in this study.
In addition to this step forward for machine-learning search methods, the materials discovery community as a whole will benefit from the data itself. The team from MIT, in collaboration with the Toyota Research Institute, has recently put the finishing touches on a cloud-based database where other researchers can use their 5.7-terabyte dataset and contribute findings and analysis functions of their own.
Raw data from materials-discovery research comes in large chunks that are difficult to download and share among scientists. This new platform will allow scientists to view and analyze the data without downloading it, as well as upload their own findings, a major step toward greater collaboration in the field.
Now that the search space for polymer electrolytes has been blown open from 20 to 40,000, Xie says the next step is to scale the technique up for other uses across materials science. “We hope that this platform is something that can be more broadly used beyond polymer electrolytes, because the concept of data-sharing is very important for the materials discovery community,” he said. “Having a platform like this is huge. We have the biggest dataset out there, which means there’s a lot of interesting machine learning to do.”
About NERSC and Berkeley Lab
The National Energy Research Scientific Computing Center (NERSC) is a U.S. Department of Energy Office of Science User Facility that serves as the primary high-performance computing center for scientific research sponsored by the Office of Science. Located at Lawrence Berkeley National Laboratory, the NERSC Center serves more than 7,000 scientists at national laboratories and universities researching a wide range of problems in combustion, climate modeling, fusion energy, materials science, physics, chemistry, computational biology, and other disciplines. Berkeley Lab is a DOE national laboratory located in Berkeley, California. It conducts unclassified scientific research and is managed by the University of California for the U.S. Department of Energy. »Learn more about computing sciences at Berkeley Lab.
Source: Elizabeth Ball, NERSC