Sam Altman Debunks GPT-5 Rumors, Shifts Focus to Improving Existing Models
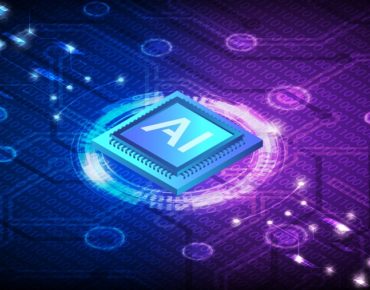
Those anticipating an eventual GPT-5 release may have a long time to wait. The current trend of developing ever-larger AI models like GPT-4 may soon come to an end, according to OpenAI CEO Sam Altman.
“I think we’re at the end of the era where it’s going to be these, like, giant, giant models. We’ll make them better in other ways,” he said on a Zoom call at an MIT event earlier this month.
Scaling up these GPT language models with increasingly larger training datasets has led to an impressive array of AI language capabilities, but Altman believes that continuing to grow the models will not necessarily equate to further advancements. Some have taken this statement to mean that GPT-4 may be the final significant breakthrough to result from OpenAI’s current approach.
During the event, Altman was asked about the recent letter asking to pause AI research for six months, signed by 1,200 professionals in the AI space, that alleged the company is already training GPT-5, the presumed successor to GPT-4.
“An earlier version of the letter claimed we were training GPT-5. We are not and we won’t be for some time, so in that sense, it was sort of silly, but we are doing other things on top of GPT-4 that I think have all sorts of safety issues that are important to address and were totally left out of the letter.”
Altman did not elaborate on what those other projects could be but said it will be important to focus on increasing the capabilities of the technology as it stands. While it is known that OpenAI’s previous model, GPT-3.5, was trained on 175 billion parameters, the company did not release the parameter count for GPT-4, citing concerns over sensitive proprietary information. Altman says increasing parameters should not be the goal: “I think it’s important that what we keep the focus on is rapidly increasing capability. If there’s some reason that parameter count should decrease over time, or we should have multiple models working together, each of which are smaller, we would do that. What we want to deliver to the world is the most capable and useful and safe models,” he said.
Since its release in November, the world has been enamored with ChatGPT, the chatbot enabled by OpenAI’s large language models. Tech giants like Google and Microsoft have scrambled to either incorporate ChatGPT into their own products or speed up the development of similar technology. Several startups are competing to build their own LLMs and chatbots, such as Anthropic, a company seeking to raise $5 billion for the next generation of its Claude AI assistant.
It would make sense to focus on making LLMs better in their current form, as there are valid concerns with their accuracy, bias, and safety. GPT-4’s accompanying technical paper acknowledges this: “Despite its capabilities, GPT-4 has similar limitations to earlier GPT models: it is not fully reliable (e.g., can suffer from “hallucinations”), has a limited context window, and does not learn. Care should be taken when using the outputs of GPT-4, particularly in contexts where reliability is important,” the paper states.
The GPT-4 paper also cautions again overreliance on the model’s output, something that could increase as the model’s size and power grows: “Overreliance is a failure mode that likely increases with model capability and reach. As mistakes become harder for the average human user to detect and general trust in the model grows, users are less likely to challenge or verify the model’s responses,” it says.
Overall, Altman’s shift in focus to improving LLMs over continuing to scale them mirrors the sentiment that other AI researchers have raised concerning model size in the past. Google infamously fired members of its Ethical Artificial Intelligence Team for their work on a research paper that asked, “How big is too big?” when it comes to LLMs. The paper looks at how these models are “stochastic parrots” in how they cannot assign meaning or understanding to the statistics-driven text outputs they create and examined the social and environmental risks involved with their development. The paper mentions that the larger these models grow, the harder it will be to mitigate these issues.
This article originally appeared on sister site Datanami.