Nvidia Announces ‘1 Exaflops’ AI Supercomputer with 256 Grace-Hopper Superchips
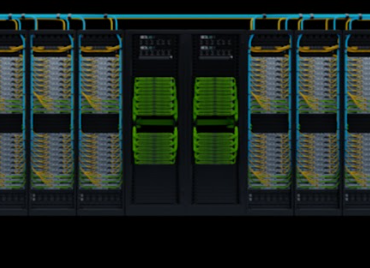
A new system from Nvidia might live up to the moniker "AI supercomputer": the DGX GH200. Announced on Monday at Computex in Taipei, the newest addition to Nvidia’s burgeoning portfolio ties together 256 Grace-Hopper Superchips, connected by 36 NVLink Switches, to provide over 1 exaflops of FP8 AI performance (or nearly 9 petaflops of FP64 performance). The system further touts 144TB of unified memory, 900 GB/s of GPU-to-GPU bandwidth and 128 TB/s bisection bandwidth. Nvidia is readying the product for end-of-year availability.
“We’re building it now,” said Nvidia CEO Jensen Huang during the livestreamed Computex keynote. “Every component is in production.”
“We [designed] DGX GH200 as a new tool for the development of the next generation generative AI models and capabilities,” said Ian Buck, VP and general manager of hyperscale and HPC at Nvidia, in a press briefing held earlier.
Hyperscalers Google, Meta and Microsoft – not surprisingly – have already queued up to kick the proverbial tires. AWS is not on that early access list.
“[These hyperscalers] will be the first to get access to the DGX GH200 to understand the new capabilities of Grace Hopper, and the multi-node NVLink that allows all those GPUs to work together as one,” said Buck.
One of the system’s top attributes is its 144TB of addressable memory, a combination of the HBM memory of the Hopper GPUs and the LPDDR5X memory of all the Grace CPUs in the network.
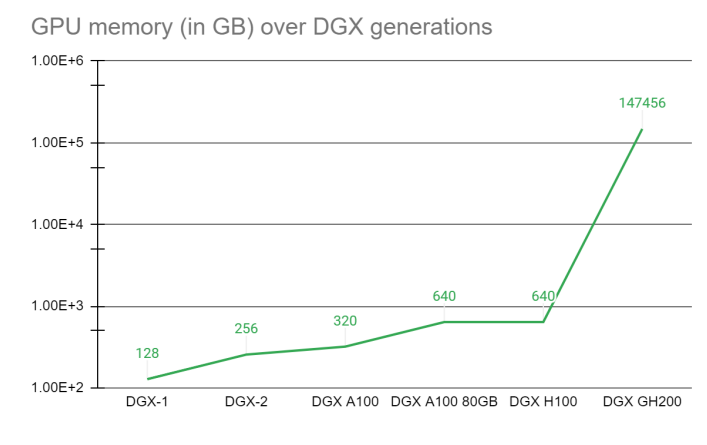
Figure shows DGX memory over generations. The DGX GH200 includes the memory of the Arm “Neoverse V2” CPUs, each with 480GB of LPDDR5X memory, and the H100 GPUs, each with 96GB HBM3 memory for this variant. Source: Nvidia.
DGX President Charlie Boyle highlighted the significance of this leap in memory (see chart at right). “For some applications, one of our [customers’] historic issues has been ‘my GPU acceleration is fantastic, but my application working space has to fit into GPU memory.’ We have customers with massive applications that they need to accelerate that are much bigger than the 640 gigabytes of memory provided by the Nvidia DGX H100 [equipped with 2 Intel CPUs and 8 H100 GPUs].
“The foundation of the DGX GH200 is being able to connect both via hardware in our NVLink Switch system, and software with all of the CUDA primitives, all of our communication libraries, so that customers can operate that system, because there’s 256 discrete computers, there’s 256 operating systems running in there, but our software works together with all of that so that you can launch a single job on the entire memory space using all the GPU capacity and either get a job you couldn’t do previously done or highly accelerate [one you could],” said Boyle.
To illustrate the potential speedups, Nvidia shared the following internal benchmarking projections, showing improvements from 2.2x (for the 1T GPT3) all the way to 6.3x (for the 40TB Distributed Join).
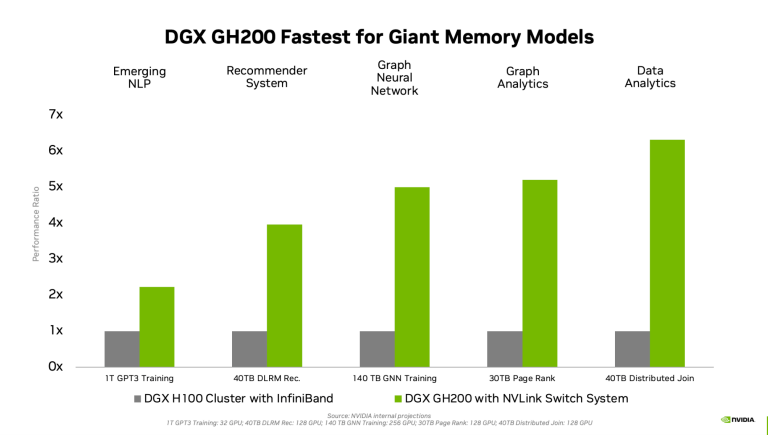
Performance comparisons going from a DGX H100 cluster with InfiniBand to a DGX GH200 fully NVLinked system. For each workload, the number of GPUs is the same for grey and green columns. Source: Nvidia.
Boyle compared the introduction of the fully NVLink-connected DGX GH200 to the arrival of NVLink in 2016. “For NVLink, which debuted with Pascal, we said every system beyond this is going to have this technology in it, and future Nvidia’s systems, future DGX systems, will also have this external NVLink capability.”
Nvidia is presenting the DGX GH200 as a 256-GPU system, which is the fully-configured version. But customers can buy in at 32 nodes, 64 nodes or 128 nodes, and can upgrade at any point along the way. “If somebody started with a 32-, they can buy another 32-; all the switching is already there,” said Boyle. “You plug in a few cables, you’ve got 64- and up and up.”
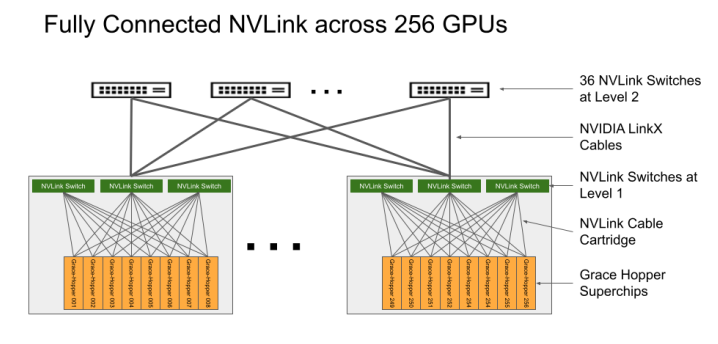
The fully configured (256-GPU) Nvidia DGX GH200 has 96 L1 NVLink Switches and 36 L2 NVLink Switches. Source: Nvidia.
This DGX GH200 is Nvidia’s first multi-rack DGX system. Each rack houses 16 Grace Hopper GH200 nodes and 256 nodes fill up 16 racks, as shown in the full article-header image, here. Bigger systems are also possible using InfiniBand to connect multiple DGX GH200s.
In fact, Nvidia is building one such mega-system – its own DGX GH200-based AI supercomputer, named Helios (the name was predicted by HPCwire) – to advance research and development and power the training of very large AI models. The system connects four DGX GH200 systems – for a total of 1,024 Grace Hopper Superchips – using Nvidia’s Quantum-2 InfiniBand networking. Nvidia is planning to bring the system online by the end of the year. Helios will provide about 4 exaflops of AI performance (FP8), and while it’s not the intended use case, would deliver ~34.8 theoretical peak petaflops of traditional FP64 performance. That would put it in the running for a spot in the top decile of the Top500 list if Nvidia opts to submit to the bi-annual list.
No HGX version of the new DGX has been announced yet, but it sounds like one is in the works. Similar to the HGX H100 design that is the foundation of Nvidia’s DGX H100 and which Nvidia makes available to hyperscalers and other system partners to customize to their specifications, Nvidia indicated that the DGX GH200 would be likewise available in an “HGX” form but the company is not making a specific announcement at this time.
On that point, Buck said, “all of these hyperscalers have their own system designs and datacenter designs and further optimize and take what we build in DGX as a blueprint in general, to build their own servers and optimize further for their datacenter infrastructure,” adding, “the components and building blocks and pieces inside of DGX will be made available to these hyperscalers so that they can take it and expand on the design for their custom datacenters and server designs. That product is known as HGX.”
That said, Nvidia is announcing the modular-focused MGX server specification. MGX is “an open, flexible and forward-compatible system reference architecture for accelerated computing,” said Buck.
(Informally, the ‘D’ in DGX stands for datacenter, the ‘H’ for hyperscale, ‘C’ is for cloud, there’s an Omniverse-targeted OVX, and now ‘M’ for modular, but this usage is not officially sanctioned by Nvidia’s PR department).
The modular architecture design was created to standardize server designs – pertaining to the mechanical, thermal and power aspects of servers – which may be outfitted with GPUs, CPUs and DPUs from Nvidia and others (both x86 and Arm). “By standardizing, the different components become replaceable, interchangeable and future-proofed to forward Nvidia products and others. With the new MGX reference architecture, we see the possibility to create a new design in as little as two months at a fraction of the cost,” said Buck, who had cited that the design process as it exists currently can take as long as 18 months.
MGX will support the following form factors:
- Chassis: 1U, 2U, 4U (air or liquid cooled)
- GPUs: Full Nvidia GPU portfolio including the latest H100, L40, L4
- CPUs: Nvidia Grace CPU Superchip, GH200 Grace Hopper Superchip, x86 CPUs
- Networking: Nvidia BlueField-3 DPU, ConnectX-7 network adapters
If you’re wondering how MGX is different from HGX, Nvidia provided this explanation: “MGX differs from Nvidia HGX in that it offers flexible, multi-generational compatibility with Nvidia products to ensure that system builders can reuse existing designs and easily adopt next-generation products without expensive redesigns. In contrast, HGX is based on an NVLink-connected multi-GPU baseboard tailored to scale to create the ultimate in AI and HPC systems.”
In other words, HGX is “just a baseboard,” while MGX is a full reference architecture.
MGX is compatible with the Open Compute Project and Electronic Industries Alliance server racks and is supported by Nvidia’s full software stack, including Nvidia AI Enterprise.
ASRock Rack, ASUS, GIGABYTE, Pegatron, QCT and Supermicro have begun to implement MGX into their product design process. Two such products were announced today with planned availability in August: QCT’s S74G-2U system is based on the Nvidia GH200 Grace Hopper Superchip, and Supermicro’s ARS-221GL-NR system employs the Nvidia Grace CPU Superchip.
Another launch partner, Softbank, is relying on MGX to craft custom servers that will be deployed in its hyperscale datacenters across Japan. The design that Softbank created using the blueprints will help them dynamically allocate GPU resources in multi-use settings, for example to support both generative AI and 5G workloads.
Nvidia also declared that the GH200 Grace Hopper Superchip is in full production. In total, Nvidia says it now has more than 400 system configurations based on its latest CPU and GPU architectures – including Nvidia Grace, Nvidia Hopper and Nvidia Ada Lovelace – targeting the demand for generative AI. The systems all align with Nvidia’s software stack, including Nvidia AI Enterprise, Omnivere and the RTX platform.
No external system wins have yet been announced for the DGX GH200, but several Grace Hopper Superchip systems have been announced previously, using the same GH200 Superchips. Alps, the new Swiss supercomputing infrastructure at CSCS, is slated to debut the hybrid Arm-GPU architecture, while the U.S. prepares for the arrival of its first Grace Hopper system, “Venado,” at Los Alamos National Laboratory. Grace Hopper Superchips will also power the new Shaheen III supercomputer at KAUST. All three supercomputers are being built by HPE and are exected to be fully operational and available to researchers next year.
Nvidia declined to answer a question about the power draw of the DGX GH200 system, and likewise didn’t provide pricing info, but noted that all DGX products are sold through partners who help set the final customer prices. Regarding TDP, if you take the DGX H100 as a proxy, and multiple the max power consumption of 10.2kW by 32 (to get to 256 GPUs), that comes out to 326.4kW. We’ll update when the actual power spec becomes available.
Related
With over a decade’s experience covering the HPC space, Tiffany Trader is one of the preeminent voices reporting on advanced scale computing today.