MLPerf Releases Latest Inference Results and New Storage Benchmark
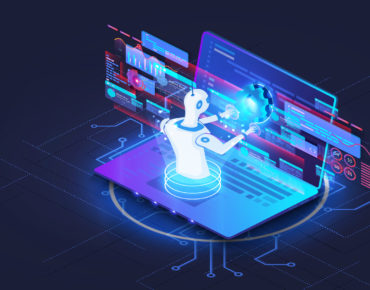
MLCommons this week issued the results of its latest MLPerf Inference (v3.1) benchmark exercise. Nvidia was again the top performing accelerator, but Intel (Xeon CPU) and Habana (Gaudi1 and 2) performed well. Google provided a peak at its new TPU (v5e) performance. MLCommons also debuted a new MLPerf Storage (v0.5) benchmark intended to measure storage performance under ML training workloads. Submitters in the first Storage run included: Argonne National Laboratory (ANL), DDN, Micron, Nutanix, and Weka.
Digging through the latest Inference results – more than 12,000 performance and 5,000 power inferencing results from 120 systems – is a challenge. There were a more modest 28 results in the storage category. From a usefulness perspective, MLCommons provides direct access to results spreadsheets that permit potential system users/buyers to drill down onto specific system configurations and benchmark tests for comparison. (Links to Inference Datacenter and Edge v3.1 results and Storage v0.5 results)
In the past, HPCwire has tended to try to cover the full exercise in a single article. The rising number of results and introduction of a new category make this less tenable. Instead, we’ll present a broad overview in this article and drill deeper into some vendor-specific results in separate articles (Nvidia and Intel/Habana). By now, you may be familiar with the MLPerf release cadence which is twice yearly for training and inference, with each released on alternate quarters. - so, inference results are released in spring and (early) fall; training results are released in winter and summer. The HPC Training benchmark is released just once yearly, close to the annual SC conference.
Broadly, inferencing and training are the foundational pieces of ML applications, with training deemed the more computational-intense of the two (i.e. think of training LLMs with trillions of parameters). Inferencing, though, is the volume workhorse, sitting behind every chatbot and similar applications.
MLPerf Inference v3.1 introduced two new benchmarks to the suite. The first is a large language model (LLM) using the GPT-J reference model to summarize CNN news articles; it garnered results from 15 different submitters, reflecting the rapid adoption of generative AI. The second change is an updated recommender, meant be more representative of industry practices, using the DLRM-DCNv2 reference model and larger datasets; it had 9 submissions. These new tests, say MLCommons, help advance AI by ensuring that industry-standard benchmarks represent the latest trends in AI adoption to help guide customers, vendors, and researchers, says MLCommons.
In a pre-briefing, David Kanter, MLCommons executive director, said, “We added our first generation recommender a couple of years ago and are now updating it. The LLM (inference) benchmark is brand new and reflects the explosion of interest in what people are calling generative AI, large language models.” An LLM had been added to the MLPerf Training benchmark in the spring (see HPCwire coverage, MLPerf Training 3.0 Showcases LLM; Nvidia Dominates, Intel/Habana Also Impress)
No ML benchmarking effort today would be complete without LLM coverage and MLCommon (parent organization for MLPerf) now has that.
“It’s important to understand large language models operate on tokens. A token is typically a piece of a word. An LLM simply takes a set of tokens as input and predicts the next token. Now, you can chain this together to actually build a predicted sentence. In practice, LLM s are used in a wide variety of applications. You can use them in search and in generating content, like essays or summaries. Summarization is what we do here,” said Kanter.
The MLPerf LLM inference benchmark is quite different from the training benchmark, he emphasized.
“One of the critical differences is the inference LLM is fundamentally performing a generative task. It's writing fairly lengthy sentences, multiple sentences, [but] it’s also actually a different and smaller model,” he said. “Many folks simply don't have the compute or the data to really support a really large model. The actual task we're performing with our inference benchmark is text summarization. So we feed in an article and then tell the language model to summarize the article.”
As is MLCommons’ practice, submitting organizations are invited to submit brief statements on their submissions. These range in quality from pure marketing to providing more granular technical descriptions of a submission's distinguishing features. Given the high number of results, a fast review of the vendor statements can be informative in conjunction with consulting the spreadsheet.
Both Inference and storage submitter statements are appended to the end of this article. As examples, here are a few snippets from a few vendor statements in MLPerf Inference v3.1 exercise:
Azure promoted its online versus on premise showing access to H100 instances. “Azure was the only submitter to publish results for virtual machines in the cloud, while matching the performance of on premises and bare metal offerings. This has been possible thanks to innovative technologies including: AI supercomputing GPUs: Equipped with eight NVIDIA H100 Tensor Core GPUs, these VMs promise significantly faster AI model performance than previous generations, empowering businesses with unmatched computational power; Next-generation computer processing unit (CPU): Understanding the criticality of CPU performance for AI training and inference, we have chosen the 4th Gen Intel Xeon Scalable processors as the foundation of these VMs, ensuring optimal processing speed."
CTuning Foundation, the non-profit ML tool developer, noted that it “[delivered the new version of the open-source MLCommons CM automation language, CK playground and modular inference library (MIL) that became the 1st and only workflow automation enabling mass submission of more than 12000 performance results in a single MLPerf inference submission round with more than 1900 power results across more than 120 different system configurations.”
Google touted its new TPU v5e. “TPU v5e systems use multiple accelerators linked together by a high-speed interconnect and can be configured with a topology ranging from 1x1 to 16x16 (256 chips), giving the user the flexibility to choose the system that best meets their needs. This wide range of topology options offered by TPU systems allows users to run and scale AI inference workloads cost-effectively, without compromising on performance."
“In this submission, Google Cloud used a TPU v5e system with a 2x2 topology (4 TPU chips) to run the 6-billion-parameter GPTJ benchmark. This benchmark demonstrates both the ease of scaling and the cost-efficiency offered by the TPU v5e systems for inference of large language models. Users can easily add more TPU v5e instances to achieve higher total queries per second (QPS), while maintaining the same performance per dollar advantage.”
HPE reported, “In the datacenter category, HPE Cray systems with eight (8) NVIDIA GPUs led our portfolio in performance, delivering more than 340,000 samples per second throughput for ResNet-50 Computer Vision, and more than 28,000 samples per second throughput for Bert 99.0 NLP. HPE also submitted for the first time the newly available HPE ProLiant DL380a Gen11 and HPE ProLiant DL320 Gen11 servers with NVIDIA H100 and L4 GPUs. The HPE ProLiant DL380a Gen11 with four (4) NVIDIA H100 GPUs is ideal for NLP and LLM inference. The HPE ProLiant DL320 Gen11 with four (4) NVIDIA L4 GPUs is a 1U server positioned for computer vision inference.”
Intel discussed Gaudi2 accelerators, 4th Gen Intel Xeon Scalable processors and Intel Xeon CPU Max Series. “Gaudi2 performance on both GPT-J-99 and GPT-J-99.9 for server queries and offline samples are 78.58/second and 84.08/second, respectively. These outstanding inference performance results complement our June training results and show continued validation of Gaudi2 performance on large language models. Performance and model coverage will continue to advance in the coming benchmarks as Gaudi2 software is updated continually with releases every six to eight weeks.
“Intel remains the only server CPU vendor to submit MLPerf results. Our submission for 4th Gen Intel Xeon Scalable processors with Intel AMX validates that CPUs have great performance for general purpose AI workloads, as demonstrated with MLPerf models, and the new and larger DLRM v2 recommendation and GPT-J models.”
You get the general flavor. It’s necessary to dig into the spreadsheet for meaningful comparisons.
MLPerf Debuts Storage Benchmark
The new storage benchmark (v0.5) has been in the works for two years. MLCommons says, “It’s the first open-source AI/ML benchmark suite that measures the performance of storage for ML training workloads. The benchmark was created through a collaboration spanning more than a dozen leading industry and academic organizations and includes a variety of storage setups including: parallel file systems, local storage, and software defined storage. The MLPerf Storage Benchmark will be an effective tool for purchasing, configuring, and optimizing storage for machine learning applications, as well as for designing next-generation systems and technologies.”
Although it’s being introduced along with the latest inference results, storage performance in ML is typically a more sensitive system element in training. MLCommons notes, “Training neural networks is both a compute and data-intensive workload that demands high-performance storage to sustain good overall system performance and availability. For many customers developing the next generation of ML models, it is a challenge to find the right balance between storage and compute resources while making sure that both are efficiently utilized.”
MLPerf Storage is intended to help overcome this problem by accurately modeling the I/O patterns posed by ML workloads, providing the flexibility to mix and match different storage systems with different accelerator types. The new benchmark reports results in sample/s and MB/s. Of course, the choice of storage hardware, protocol/filesystem, and network all influence performance.
The MLPerf Storage benchmark suite is built on the codebase of DLIO, a benchmark designed for I/O measurement in high performance computing, adapted to meet current storage needs.
Talking about the motivation and goals for the new benchmark, Kanter said “I’d heard about pretty large hyperscalers, who deployed really large training clusters, that could not hit their peak utilization because they didn't have enough storage. That [suggested] there's fundamentally a hard problem in storage and one that's under appreciated. Most hyperscalers that are buying 1000s, or tens of 1000s of accelerators also have engineers on staff to design proper storage subsystems."
“The key accomplishment is we created a tool that represents ML training IO patterns, that doesn't require having any compute or accelerators,” said Kanter. “That's important, because if you want to size a storage subsystem for 1000 accelerators, you don't want to have to buy 1000 accelerators. Another interesting thing is it’s a dynamic tool that is coupled to compute. The metric for MLPerf storage is how many samples per second can be streamed out, for a given compute utilization; so we model a compute subsystem. If your storage falls behind too much, the compute subsystem will be idle, and we only allow 10% idle due to storage.”
If the storage system us too slow, you can’t run the benchmark, said Kanter. Obviously, these are early days for MLPerf Storage and it will take some time for the community take its full measure. There are already plans for additions. Given its newness, it’s best look through MLCommon’s documentation. (Link to MLPerf Storage Benchmark Rules)
Link to MLCommons, https://mlcommons.org/en/
VENDOR SUPPLEMENT STATEMENTS ON INFERENCING RESULTS (Unedited)
ASUSTeK
ASUStek recently benchmarked its new AI servers using the MLPerf Inference v3.1 suite, aiming to highlight its performance across varied deep learning tasks. Our results exhibit our system's competency in inferencing some of the most demanding models with remarkable efficiency.
In the modern era of AI, speed and efficiency in deploying machine learning models to production are paramount. Enter ASUS GPU Server portfolios - designed to redefine the standards of inference, as validated by our recent MLPerf Inference benchmarks. Harness the power of AI frameworks like TensorFlow, PyTorch, and more. ASUS servers are not just about raw power; they're about smart power. Optimized software-hardware integrations ensure that you get the most out of every tensor operation. Power doesn’t have to come at the cost of the planet. ASUS GPU servers not only boast top-tier performance metrics but do so with impressive energy efficiency ratings, as highlighted in the MLPerf power efficiency results. Seamlessly scale your AI workloads. With our multi-GPU configurations and optimized in hardware and software, ASUS GPU servers are built to handle increasing data demands, ensuring you’re always ahead of the curve.
System Configuration:
Hardware: ASUS flagship AI Server ESC8000A-E12 with Dual AMD Genoa CPU up to 8 NVIDIA H100 GPUs, and ESC4000A-E12 with Dual AMD Genoa CPU up to 8 L4 GPUs
The results signify the DL system's enhanced performance and capability to address contemporary deep learning challenges, making it an apt choice for researchers and industries requiring accelerated inferencing workloads.
Azure
Microsoft Azure announced the general availability of the ND H100 v5-series for Generative AI at scale. These series of virtual machines vary in sizes ranging from eight to thousands of NVIDIA H100 GPUs interconnected by NVIDIA Quantum-2 InfiniBand networking. Azure was the only submitter to publish results for virtual machines in the cloud, while matching the performance of on premises and bare metal offerings. This has been possible thanks to innovative technologies including:
- AI supercomputing GPUs: Equipped with eight NVIDIA H100 Tensor Core GPUs, these VMs promise significantly faster AI model performance than previous generations, empowering businesses with unmatched computational power.
- Next-generation computer processing unit (CPU): Understanding the criticality of CPU performance for AI training and inference, we have chosen the 4th Gen Intel Xeon Scalable processors as the foundation of these VMs, ensuring optimal processing speed.
- Low-latency networking: The inclusion of NVIDIA Quantum-2 ConnectX-7 InfiniBand with 400Gb/s per GPU with 3.2 Tb/s per VM of cross-node bandwidth ensures seamless performance across the GPUs, matching the capabilities of top-performing supercomputers globally.
- Optimized host to GPU performance: With PCIe Gen5 providing 64GB/s bandwidth per GPU, Azure achieves significant performance advantages between CPU and GPU.
- Large scale memory and memory bandwidth: DDR5 memory is at the core of these VMs, delivering greater data transfer speeds and efficiency, making them ideal for workloads with larger datasets.
- These VMs have proven their performance prowess, with up to six times more speedup in matrix multiplication operations when using the new 8-bit FP8 floating point data type compared to FP16 in previous generations. The ND H100 v5 VMs achieve up to two times more speedup in large language models like BLOOM 175B end-to-end model inference, demonstrating their potential to optimize AI applications further.
The ND H100 v5 is now available in the East United States and South Central United States Azure regions. Enterprises can register their interest in access to the new VMs or review technical details on the ND H100 v5 VM series at Microsoft Learn.
CTuning
As a founding member of MLCommons, cTuning.org is committed to democratizing MLPerf benchmarks and making them accessible to everyone to deliver the most efficient AI solutions while reducing all development, benchmarking and optimization costs.
We are proud to deliver the new version of the open-source MLCommons CM automation language, CK playground and modular inference library (MIL) that became the 1st and only workflow automation enabling mass submission of more than 12000 performance results in a single MLPerf inference submission round with more than 1900 power results across more than 120 different system configurations from different vendors (different implementations, all reference models and support for DeepSparse Zoo, Hugging Face Hub and BERT pruners from the NeurIPS paper, main frameworks and diverse software/hardware stacks) in both open and closed divisions!
This remarkable achievement became possible thanks to open and transparent development of this technology as an official MLCommons project with public Discord discussions, important feedback from Neural Magic, TTA, One Stop Systems, Nutanix, Collabora, Deelvin, AMD and NVIDIA, and contributions from students, researchers and even school children from all over the world via our public MLPerf challenges. Special thanks to cKnowledge for sponsoring our developments and submissions, to One Stop Systems for showcasing the 1st MLPerf results on Rigel Edge Supercomputer, and to TTA for sharing their platforms with us to add CM automation for DLRMv2 available to everyone.
Since it’s impossible to describe all the compelling performance and power-efficient results achieved by our collaborators in a short press-release, we will make them available with various derived metrics (power efficiency, cost, etc) and reproducibility reports at the MLCommons CK playground (x.cKnowledge.org), github.com/mlcommons/ck_mlperf_results and github.com/mlcommons/ck/blob/master/docs/news-mlperf-v3.1.md shortly after official release.
We continue enhancing the MLCommons CM/CK technology to help everyone
automatically co-design the most efficient end-to-end AI solutions
based on their requirements and constraints. We welcome all submitters to join our public MLCommons Task Force on Automation and Reproducibility if you want to automate your future MLPerf submissions at scale.
Connect Tech Inc
As a new member of MLCommons, Connect Tech ran performance and accuracy benchmarks in the Inference: Edge category in its recent MLPerf submission. Using Connect Tech’s feature-rich Hadron carrier board with the NVIDIA Jetson Orin NX, a high-performance, energy-efficient platform, showcased remarkable levels of performance across various AI workloads.
Connect Tech additionally supports NVIDIA Jetson Orin NX with Photon and Boson carrier boards, and system devices like Polaris and Rudi-NX. By deploying on Connect Tech’s production-ready hardware, customers can take immediate advantage of Jetson Orin NX for performance improvements and enhanced user experience with robotics and other edge AI applications.
Connect Tech's involvement in MLCommons signifies more than just technical achievement. It reflects the company's commitment to pushing the envelope of what's possible in the world of AI at the edge. The seamless integration of Connect Tech's hardware with NVIDIA's cutting-edge technology presents engineers and scientists with the tools to drive AI and machine learning innovations across diverse industries, including robotics, industrial automation, and healthcare.
Connect Tech is a hardware design and manufacturing company, specializing in rugged, small form factor solutions. As an Elite NVIDIA Jetson ecosystem partner, Connect Tech designs carrier boards, enclosures, and embedded systems for each Jetson generation. With a rich history of innovation, Connect Tech integrates edge AI solutions within various industries, empowering engineers and scientists to harness the potential of machine learning.
Connect Tech remains at the forefront as the world delves deeper into AI and machine learning. Navigating the complex landscape of embedded AI computing is made easier by using NVIDIA and Connect Tech’s innovative products.
Dell
Enterprise IT is bracing for the most transformative technology trend in decades: generative AI. Dell Technologies is ready to meet this demand with the world’s broadest Generative AI solutions portfolio from desktop to edge to data center to cloud, all in one place.
For the MLPerf inferencing v3.1 benchmark testing, Dell submitted 230 results, including the new GPT-J and DLRMv2 benchmark results, across 20 system configurations. Dell Technologies works with customers and collaborators, including NVIDIA, Intel, and Qualcomm, to optimize performance and efficiency, boosting inferencing workloads, including generative AI.
The Dell PowerEdge XE accelerated server family continues to deliver tremendous performance gains across several benchmarks. Here are some of the latest highlights:
- The PowerEdge XE9680 with 8 NVIDIA H100 SXM GPUs continues to deliver Dell’s best performance results, up to ~16% better performance than the previous MLPerf 3.0 benchmark results in Image classification, Speech-to-text, Language processing, and Recommendation.
- Stellar results for the complete PowerEdge XE server family, including the Direct Liquid Cooled PowerEdge XE9640 that packs either 4 NVIDIA H100 SXM GPUs or 4Intel Data Center GPU Max OAM GPUs in an ultra-dense 2RU profile.
- New NVIDIA L40 GPU results in the PowerEdge R760xa, Dell’s most versatile accelerated server with the best price-to-GPU performance ratio.
- New Intel Xeon CPU-based machine learning and GPT-J results for the PowerEdge R760.
- The rugged, edge-optimized PowerEdge XR5610 yielded impressive power efficiency relative to performance results with the NVIDIA L4 GPU.
- Updated Qualcomm results featuring the compact and energy-efficient PowerEdge XR4520c compute sled with Qualcomm Cloud AI 100 Standard accelerator cards.
Generate higher quality, faster time-to-value predictions and outputs while accelerating decision-making with powerful solutions from Dell Technologies. Take a test drive in one of our worldwide Customer Solution Centers. Collaborate with our Innovation Lab and tap into one of our Centers of Excellence.
Fujitsu
Fujitsu offers a fantastic blend of systems, solutions, and expertise to guarantee maximum productivity, efficiency, and flexibility delivering confidence and reliability. Since 2020, we have been actively participating in and submitting to inference and training rounds for both data center and edge divisions.
In this round, Fujitsu demonstrated the performance of PRIMERGY CDI with four A100-PCIe-80GB GPUs installed in an external PCIe BOX and measured the benchmark program only for the data center closed division. Fujitsu Server PRIMERGY CDI is expertly engineered to deploy the necessary resources according to each customer's unique workload, releasing them when no longer needed. CDI stands for Composable Disaggregated Infrastructure, a next-generation technology that supports the diversification of data processing. This results in an efficient operation that maximizes resource utilization, while providing user-friendly services that eliminate the drawbacks of traditional physical servers.
As demonstrated by the impressive results of this round, the PRIMERGY CDI confirms that even with GPUs mounted in an external PCIe BOX, it delivers outstanding performance and remarkable scalability for PCIe components.
Our purpose is to make the world more sustainable by building trust in society through innovation. With a rich heritage of driving innovation and expertise, we are dedicated to contributing to the growth of society and our valued customers. Therefore, we will continue to meet the demands of our customers and strive to provide attractive server systems through the activities of MLCommons.
Giga Computing
Giga Computing Technology, a subsidiary wholly owned by GIGABYTE, is the enterprise unit that split off from GIGABYTE that designs, manufactures, and sells servers, server motherboards, immersion solutions, and workstations. As the GIGABYTE brand is widely recognized, Giga Computing will continue to use and promote it, and that includes at expos where we will join as GIGABYTE. Although the company name has changed, our customers can still expect the same quality and services as before. Giga Computing strives to do better and that includes greater push for efficiency and cooling with immersion and DLC technology. As well as providing public AI benchmarks.
As one of the founding members of MLCommons, GIGABYTE has continued to support the community’s efforts in benchmarking server solutions for various AI training & inference workloads. In the latest round of MLPerf Inference v3.1, Giga Computing submitted a powerful GIGABYTE system for platforms: Intel Xeon & NVIDIA H100 SXM5, and the results speak for themselves while showing great efficiency as measured in performance/watt. We did find that our system achieved excellent performance in some tests such as rnnt-Server and bert99-offline. We would have liked to have more benchmarks, but due to resource limitations we are not able; however, we found that our partners NVIDIA, Qualcomm, and Krai chose our GIGABYTE servers to do their own testing.
Google Cloud recently launched an expansion to its AI infrastructure portfolio - Cloud TPU v5e - and is proud to announce its performance results in this round of MLPerf Inference (data center category). TPU v5e systems use multiple accelerators linked together by a high-speed interconnect and can be configured with a topology ranging from 1x1 to 16x16 (256 chips), giving the user the flexibility to choose the system that best meets their needs. This wide range of topology options offered by TPU systems allows users to run and scale AI inference workloads cost-effectively, without compromising on performance.
In this submission, Google Cloud used a TPU v5e system with a 2x2 topology (4 TPU chips) to run the 6-billion-parameter GPTJ benchmark. This benchmark demonstrates both the ease of scaling and the cost-efficiency offered by the TPU v5e systems for inference of large language models. Users can easily add more TPU v5e instances to achieve higher total queries per second (QPS), while maintaining the same performance per dollar advantage.
We are looking forward to seeing what Google Cloud customers achieve with the new TPU v5e systems.
HPE
HPE successfully submitted results in partnership with Intel, NVIDIA, Qualcomm, and Krai. HPE demonstrated a range of high-performing inference systems for both the datacenter and edge in Computer Vision, natural language processing (NLP), and large language models (LLM).
In the datacenter category, HPE Cray systems with eight (8) NVIDIA GPUs led our portfolio in performance, delivering more than 340,000 samples per second throughput for ResNet-50 Computer Vision, and more than 28,000 samples per second throughput for Bert 99.0 NLP.
HPE also submitted for the first time the newly available HPE ProLiant DL380a Gen11 and HPE ProLiant DL320 Gen11 servers with NVIDIA H100 and L4 GPUs. The HPE ProLiant DL380a Gen11 with four (4) NVIDIA H100 GPUs is ideal for NLP and LLM inference. The HPE ProLiant DL320 Gen11 with four (4) NVIDIA L4 GPUs is a 1U server positioned for computer vision inference. The HPE ProLiant DL380a Gen11 showed strong inference performance using 4th Gen. Intel Xeon Scalable Processors in CPU-only inference scenarios. The HPE ProLiant DL385 Gen10 Plus v2 with eight (8) Qualcomm Cloud AI 100 Standard accelerators remained well balanced for over-network inference compared to offline datacenter performance. Qualcomm Cloud AI 100 Standard is ideal for both computer vision and NLP inference.
In the Edge category, HPE Edgeline e920d powered by four (4) Qualcomm Cloud AI 100 Standard accelerators remains one of the lowest latency systems in the Edge category for SingleStream and MultiStream inference scenarios. The HPE Edgeline e920d also achieved strong performance improvements in throughput and energy efficiency.
Many thanks to Krai’s collaboration in achieving high-performance and energy efficiency for Qualcomm Cloud AI 100 accelerators.
IEI
IEI Industry Co., LTD is a leading provider of data center infrastructure, cloud computing, and AI solutions, ranking among the world’s top 3 server manufacturers. Through engineering and innovation, IEI delivers cutting-edge computing hardware design and extensive product offerings to address important technology arenas like open computing, cloud data center, AI, and deep learning.
In MLCommons Inference v3.1, IEI submitted the NF5468M6 system.
NF5468M6 is a highly versatile 4U AI server supporting between 4 and 16 NVIDIA single and double-width GPUs, making it ideal for a wide range of AI applications including AI cloud, IVA, video processing and much more. NF5468M6 offers ultra-high storage capacity and the unique function of switching topologies between Balance, Common and Cascade in one click, which helps to flexibly adapt to various needs for AI application performance optimization.
Intel
Intel is pleased to report MLPerf Inference v3.1 performance results for our Gaudi2 accelerators, 4th Gen Intel Xeon Scalable processors and Intel Xeon CPU Max Series. These results reinforce Intel’s commitment to delivering the full spectrum of products to address wide-ranging customer AI requirements.
Gaudi2 performance on both GPT-J-99 and GPT-J-99.9 for server queries and offline samples are 78.58/second and 84.08/second, respectively. These outstanding inference performance results complement our June training results and show continued validation of Gaudi2 performance on large language models. Performance and model coverage will continue to advance in the coming benchmarks as Gaudi2 software is updated continually with releases every six to eight weeks.
Intel remains the only server CPU vendor to submit MLPerf results. Our submission for 4th Gen Intel Xeon Scalable processors with Intel AMX validates that CPUs have great performance for general purpose AI workloads, as demonstrated with MLPerf models, and the new and larger DLRM v2 recommendation and GPT-J models.
The results confirm that 4th Gen Intel Xeon Scalable processor with optimized data pre-processing, modeling and deployment tools and optimizations, is an ideal solution to build and deploy general purpose AI workloads with the most popular open source AI frameworks and libraries.
For the GPT-J 100-word summarization task of a news article of approximately 1,000 to 1,500 words, 4th Gen Intel Xeon processors summarized two paragraphs per second in offline mode and one paragraph per second in real-time server mode.
This is the first time we’ve submitted MLPerf results for our Intel Xeon CPU Max Series, which provides up to 64GB of high-bandwidth memory. For GPT-J, it was the only CPU able to achieve 99.9% accuracy, which is critical for usages for which the highest accuracy is of paramount importance.
With our ongoing software updates, we expect continued advances in performance and productivity, and reporting new training metrics with the November training cycle.
For more details, please see MLCommons.org.
Notices & Disclaimers
Performance varies by use, configuration and other factors. Learn more at www.Intel.com/PerformanceIndex .
Performance results are based on testing as of dates shown in configurations and may not reflect all publicly available updates. See
backup for configuration details. No product or component can be absolutely secure. Your costs and results may vary.
Intel technologies may require enabled hardware, software or service activation.
© Intel Corporation. Intel, the Intel logo, and other Intel marks are trademarks of Intel Corporation or its subsidiaries. Other names and brands may be claimed as the property of others.
Krai
Established in 2020 in Cambridge, UK, KRAI comprises a dynamic group of exceptional engineers committed to transforming the practice of AI development and deployment on cutting-edge hardware. Acknowledging the growing significance of AI, and the limitations of computer systems, KRAI was founded with the mission to help propel the adoption of AI in a responsible way. We take pride in our collaborations with industry leaders such as Qualcomm, HPE, Dell, Lenovo, and more.
We are thrilled to power many v3.1 submissions with our KRAI X automation technology. In v3.0, we previewed KRAI X through a single-digit number of submissions. In v3.1, we have fully transitioned from using our acclaimed automation technology based on Collective Knowledge v1 to using KRAI X, having enabled a triple-digit number of submissions with compelling outcomes.
Notably, we dedicated considerable effort to optimizing energy efficiency. For example, on a server equipped with 16 Qualcomm Cloud AI accelerators, we achieved 237.0 QPS/W for ResNet50 and 9.2 QPS/W for BERT-99, compared with 210.7 QPS/W and 7.7 QPS/W, respectively, in the previous round (up to 20%).
Finally, we unveiled support for several new targets to our open-source KRAI Inference Library Technology (KILT): TensorRT for GPUs; ONNX Runtime for CPUs and GPUs; and Snapdragon Neural Processing Engine (SNPE) for Qualcomm's CPUs, GPUs, DSPs and NPUs.
Our team remains steadfast in enhancing KRAI technologies to deliver fully optimized end-to-end AI solutions tailored to specific constraints and performance objectives. Our technologies empower system designers to accelerate the design and deployment of AI by eliminating laborious manual processes. Our mission is to assist companies in the development, benchmarking, optimization and deployment at scale of their AI solutions.
Moffett
Moffett AI is a leader in sparse AI computing, dedicated to providing AI computing platforms and services, with a mission to continue evolving the frontiers of AI performance using sparse computing.
Following the outstanding performance in the MLPerf Inference v2.1 and v 3.0 benchmarks, Moffett AI has once again submitted impressive results for the S30 Accelerator. These results were achieved on GPT J-99 model in offline mode in the Data Center Open Division.
In MLPerf Inference v3.1, MLPerf's first introduction of large model inference, Moffett AI was the only one in the Data Center Open Division to submit results of large model GPT J inference.
The S30 Accelerator is powered by Moffett's Antoum processor - the world's First Al computing Accelerators with 32x sparsity. Moffett's patented deep dual sparsity algorithm and the co-designed Antoum chip and software platform architecture drastically increase the computing performance of the accelerators, increasing throughput, reducing latency and power, maintaining accuracy, while also significantly reducing the Total Cost of Ownership (TCO).
Moffett Al's submissions showcase the remarkable benefits of Moffett's Antoum processor, especially the advantages of sparse computing for large model inference with software and hardware co-design:
- Enabling extraordinarily low TCO: Moffett AI accelerators perform computing workloads with less infrastructure, simplified deployment, and reduced Total Cost of Ownership (TCO) to achieve overall cost reduction and efficiency.
- Excellent performance on large model inference: The S30 accelerators in 8-card mode achieve impressively high performance (170.59 Sample/s) on GPT J-99.
- Scalable highly performant across data center ecosystem: The S30 accelerator performed exceptionally well in single-card, 4-card, 8-card mode across different servers.
Moffett's deep sparse industry leading performance is optimal for generative AI workloads such as GPTJ. These AI models are massively increasing in size and the demand for computing performance is skyrocketing, as well as the need to reduce power, latency and TCO.
Neural Magic
While pursuing research at MIT, Nir Shavit and Alexander Matveev encountered the limiting barriers of GPUs and other prevailing hardware options in the realm of deep learning. This frustration drove them to develop software that unfetters AI innovation from GPUs, which ultimately led to the founding of Neural Magic in 2018. Now, enterprises and communities alike, can use Neural Magic software and algorithms to get performant and accurate AI deployments on commodity CPUs.
Neural Magic’s DeepSparse is a sparsity-aware inference runtime that delivers power-efficient AI performance on commodity CPUs, from the cloud to the edge. Our open-source compression framework, SparseML, unifies state-of-the-art sparsification algorithms for easy use and application across ML use cases like computer vision, natural language processing, and generative AI.
In partnership with the cTuning Foundation, Neural Magic leveraged the open-source CK technology to automate and reproduce results across all 74 benchmarks in MLPerf Inference v3.1. We're excited to share our DeepSparse CPU benchmarks, which showcase the performance of an array of sparsified BERT question answering models. These models are readily available for deployment from Neural Magic’s SparseZoo. They have been tested across a diverse range of platforms - like Intel and AMD, across GCP and AWS. Our benchmarks span both x86 and ARM architectures, to ensure comprehensive coverage between edge devices and robust cloud infrastructures.
As we continue to refine our algorithms to finesse performance and accuracy, particularly for large model compression, we’ve appreciated the early enthusiasm shown towards our nascent research, including GPTQ and SparseGPT. We’re excited to see our research included in initiatives that drive a new era of generative AI pursuits by partners. As we continue to push boundaries with model optimization, we are motivated by our work with customers, to redefine what's possible in the space of deep learning, all without the burden of specialized hardware, operational complexities, or daunting costs.
NVIDIA
In MLPerf Inference 3.1, we are thrilled to have made our first submission using the NVIDIA GH200 Grace Hopper Superchip, which combines NVIDIA Grace, our first data center CPU, with the NVIDIA Hopper GPU to create a processor for the era of generative AI and accelerated computing. It ran every workload in the datacenter category, including the new GPT-J and DLRMv2 tests, and extended the leading performance of the H100 Tensor Core GPU across the board.
We were also pleased to make our first available submission using the L4 Tensor Core GPU powered by the NVIDIA Ada Lovelace architecture. With a single-slot, low-profile design and low thermal design power, it can bring the performance and versatility of the NVIDIA platform in AI, video, and graphics to any server.
And on our Jetson platforms for edge AI and robotics, powered by the NVIDIA Orin system-on-chip (SoC), we were thrilled to deliver up to 85% more performance compared to our prior submissions thanks to software advances and use of the second-generation Programmable Vision Accelerator (PVA) built into Orin.
The NVIDIA AI platform delivers innovation across the full stack, accelerates the entire AI workflow end-to-end – from data preparation to model training to deployed inference from cloud to edge – and achieves great performance across a broad range of AI models. It’s also available from every major cloud and server maker, and offers the quickest path to production AI and enterprise-grade support with NVIDIA AI Enterprise.
We are thrilled to see 13 NVIDIA partners submit great inference results, with both on-prem and cloud solutions spanning the breadth of our data center GPU portfolio.
We also wish to commend the ongoing work MLCommons is doing to bring benchmarking best practices to computing, enabling peer-reviewed apples-to-apples comparisons of AI and HPC platforms to better understand and compare product performance across diverse workloads.
Nutanix
The Nutanix Cloud Platform solution is a hybrid multicloud platform that provides a software stack to enable the full lifecycle of AI/ML applications, regardless of where the hardware is deployed. The consistent operating model helps enable ease of management whether data is being collected, transformed, or fed into models for inferencing at the edge, or models are being fine-tuned at the core data center or in the public cloud.
Nutanix is pleased to announce the first set of published results with the MLPerf benchmark. The benchmark was executed in a lab environment on a Nutanix NX-3155-G8 node with two NVIDIA A100 Tensor Core GPU 80GB. It was fully virtualized on the AHV hypervisor.
Oracle
Oracle Cloud Infrastructure (OCI) offers AI Infrastructure, AI Services, ML Services, and AI in our Fusion Applications. Our AI infrastructure portfolio includes bare metal instances and virtual machines powered by NVIDIA H100 (coming soon), NVIDIA A100, and NVIDIA A10 GPUs.
The inference benchmark results for the high-end BM.GPU.H100.8 and BM.GPU.A100-v2.8 instances demonstrate that OCI provides high performance that is comparable to other deployments on both on-premises and cloud infrastructure. These instances provide eight NVIDIA GPUs per node. In addition to inferencing, for training workloads each node can be clustered using a high performance RDMA network to tens of thousands of GPUs.
The results for GPU.A10.4 indicate superior performance for smaller AI model inferencing workloads. The evaluated bare metal instance featured four NVIDIA A10 GPUs. OCI also offers virtual machines with one or two NVIDIA A10 GPUs. All three OCI instance types based on the NVIDIA A10 GPU are priced lower than their OCI counterparts with NVIDIA A100 or H100 GPUs.
Qualcomm Technologies, Inc.
The Qualcomm Cloud AI inference accelerators leverage the Company’s heritage in advanced signal processing and power efficiency to deliver high throughput, low power AI inference processing in the cloud and at the Edge. The Cloud AI products support all AI Inferencing workloads, spanning ML frameworks and network models, including large networks with multi-accelerator aggregation.
Qualcomm MLPerf v3.1 inference results demonstrate optimization of all the ML Benchmarks across the board. All our submissions show incremental upside in performance, power efficiency and lower latencies for NLP and computer-vision networks. Qualcomm partner Lenovo has added a new datacenter server platform ThinkSystem SR665v1 with five Qualcomm Cloud AI 100 PCIe Pro AI accelerators. Qualcomm and HPE have added RetinaNet network benchmarks to its Network division submissions in addition to BERT. Our partner Dell has made submission with Cloud AI 100 Standard Accelerator with PowerEdge XR4520c Server. Qualcomm AI software partner Krai.ai has made several Cloud AI accelerator-based platform submissions in Edge Category and Open Division.
Qualcomm MLPerf v3.1 inference benchmark results have surpassed its own previous records of peak offline performance, power efficiency and lower latencies in several categories. The 2U datacenter server platform with 16x Qualcomm Cloud AI 100 PCIe Pro (75W TDP) accelerators has further improved its power efficiency by another 15-20% across NLP and CV networks. The Gloria Highend, Edge appliance platform has peaked its performance and power efficiency for all three neural network categories we have submitted to. The RetinaNet performance across all platforms has been optimized by additional ~12%
Qualcomm continues to innovate and optimize AI solutions across all submissions. The Network division applicable for datacenter has been extended to RetinaNet Network in addition to BERT. All Network division submission results achieved nearly the same results as Closed division.
All submissions are powered by the KRAI X and KILT technologies.
Qualcomm is a trademark or registered trademark of Qualcomm Incorporated.
Qualcomm Cloud AI is product of Qualcomm Technologies, Inc. and/or its subsidiaries
Quanta Cloud Technology
Quanta Cloud Technology (QCT) is a global datacenter solution provider that enables diverse HPC and AI workloads, was named amongst MLPerf inference list in the latest MLPerf results released by MLCommons.
QCT participated in the latest round of MLPerf Inference v3.1 and submitted results to the data center closed division for four different system configurations.
One of the configurations showcased QCT's cutting-edge platform, QCT submitted its state-of-the-art platform QuantaGrid D54U-3U in preview with four NVIDIA H100 PCIe GPU. QuantaGrid D54U-3U is an acceleration server designed for AI/HPC. Supporting two 4th Gen Intel Xeon Scalable processors with up to 350W and 32x DIMM slots, this 3U system features support for four dual width accelerator cards or up to eight single width accelerator cards to provide a comprehensive and flexible architecture that can be optimized for various AI/HPC applications.
Additionally, QCT presented results for the QuantaGrid-D54Q-2U System, equipped with four NVIDIA L4 Tensor Core GPUs. Thanks to innovative hardware design, meticulous system tuning, and software optimization, QCT achieved outstanding performance in MLPerf Inference v3.1.
Going forward, QCT remains committed to delivering comprehensive hardware systems, solutions, and services to both academic and industrial users. The company will continue to share its MLPerf results with the MLCommons community, contributing to the advancement of MLPerf inference and training benchmarks.
SiMa
SiMa.ai prides itself on being at the forefront of edge AI technology, consistently pushing the boundaries of performance and energy efficiency. We are extremely excited to share our results in this latest MLPerf benchmark, where we achieved a 20% improvement in our scores since April 2023.
In the edge AI sector, where both performance and energy efficiency are paramount, the standout metric is frames per second per watt. This metric is a critical indicator of how many frames our system can process per watt of electricity consumed and vital to edge AI workloads. SiMa.ai’s custom-made ML Accelerator is the linchpin of our success in achieving unparalleled power efficiency without compromising performance.
Our 20% improvement since the April 2023 submission is one of the most thrilling aspects of SiMa’s latest MLPerf results. Substantial enhancements in our compiler technology and memory management systems drove this increase. These foundational improvements optimize code execution and resource allocation, boosting the overall performance of our hardware. What makes this even more compelling is the translation of these improvements beyond the benchmarks to real-world use cases. We’re able to enhance the performance of all models running on our hardware and offer our customers more value and versatility across a wide range of applications.
SiMa.ai’s consistent participation and performance in MLPerf is part of a broader growth strategy where we are transitioning from the 16nm process to more advanced technologies in future generations. This move is not merely a technical upgrade; it's a strategic evolution to ensure we continue to lead in performance, efficiency, and innovation. As we look to the future, our focus remains clear: to continue pushing the boundaries of what's possible in edge AI, particularly in performance and ease of use.
Supermicro
Supermicro has a long history of designing a wide range of products for various AI use cases. In MLPerf Inference v3.1, Supermicro has submitted four systems in the closed division, datacenter category.
Supermicro’s mission is to provide application-optimized systems for a broad range of workloads. For example, Supermicro designs and manufactures four types of systems for the NVIDIA HGX H100 8GPU and 4GPU platforms, with 16 lanes of connectivity so that nothing stands in the way of the flow of data to the accelerators, which is ideal for AI workloads that are very I/O intensive and that need a balance of CPU and GPU performance.
Supermicro offers a range of CPUs and quantities of GPU in various form factors for customers who may have differing compute and environmental requirements. Furthermore, Supermicro provides upgraded power supplies to give customers choices on using cost-effective power supplies or genuine N+N redundancy to maximize the TCO. Supermicro also offers liquid cooling options for NVIDIA HGX systems to help deployments use higher TDP CPUs and GPUs without thermal throttling.
For those in pursuit of PCIe Gen5 platforms, Supermicro presents an array of compelling alternatives. For the MLPerf v3.1 Inference, Supermicro submitted H100 results for the GPU SuperServer SYS-521GE-TNRT, a compact high-performance server with a 5U rackmount form factor. The system is currently shipping worldwide.
Supermicro’s GPU A+ Server, the AS-8125GS-TNHR (AMD CPU), and SuperServer, the SYS-821GE-TNHR (Intel CPU), both have 8 H100 SXM5 GPUs and GPU-GPU interconnect with NVLink and NVSwitch. Furthermore, the dual root configuration features directly attached 8 GPUs to achieve the lowest latency possible and improve performance, which is hugely beneficial for demanding scenarios our customers face with machine learning (ML) and HPC workloads. The models achieved outstanding results and are an excellent choice for AI development.
Supermicro's commitment extends beyond these specific models, spanning a vast assortment of GPU-based servers tailored for any conceivable environment. This steadfast commitment is evidenced by the impressive performance exhibited across a gamut of MLPerf tests. Supermicro pledges to continue raising the bar for exceptional performance, serving users' distinct needs across various workstations and servers.
TTA
Telecommunications Technology Association (TTA) is a non-profit organization that was established in 1988. Its primary mission revolves around the standardization of information and communication technology (ICT), alongside the testing and certification of various ICT products, services, and data.
TTA also supports global marketing and market share expansion for Korean server/storage hardware vendors. TTA recognizes the value of publishing performance results through platforms like MLCommons, and on this occasion, we test the server KR580S1 manufactured by KTNF Co, Ltd.
Since its founding in 2001 under the motto of Korea Technology aNd Future, KTNF has emerged as a server industry leader with the best technology and expertise
KTNF is a trusted company that not only provides server circuit and system technology for services optimized for cloud and edge computing environments, but one that also supports your business in response to the rapidly changing fourth industrial revolution environment based on expertise and excellent service.
The KR580S1 server used in this MLCommons inference v3.1 is a good system for AI, Cloud service workload in Datacenter.
This server is an Intel Xeon-SP based server that can install up to two double slot GPUs. It support DDR4 DIMM memory and High performance NVMe SSDs. As a hot tolerance server, it is monitoring the GPU card to achieve maximum performance.
TTA carried out the tests using two different GPUs: the NVIDIA A100 (40G) for Edge and the NVIDIA Tesla T4 for Edge and Datacenter. With Tesla T4, the results showed a relatively decent performance score for algorithms like resnet50, and bert-99, affirming that the server’s suitability for deployment in both the Edge Server Market and the Datacenter Server Market.
Thanks to CTuning for helping to automate our submissions using the MLCommons CM automation language and CK playground.
xFusion
xFusion Digital Technology Co., Ltd. is committed to becoming the world's leading provider of computing power infrastructure and services. We adhere to the core values of "customer-centric, striver-oriented, long-term hard work, and win-win cooperation", as we continue to create value for customers and partners, and accelerate the digital transformation of the industry.
In this performance competition of MLPerf Inference v3.1, we used a new generation of GPU server product, FusionServer G5500 V7 to conduct performance tests on all benchmarks under various GPU configurations and achieved excellent results.
FusionServer G5500 V7 (G5500 V7) is a new-generation 4U 2-socket GPU server. It supports a maximum of 10 x double-width GPU cards. We use Intel Xeon Platinum 6458Q CPU x2 and 8 to 10 A30 or L40 GPU configurations to test all evaluation items. It has made excellent achievements for bert, dlrm-v2 and gptj models. 62 test results can achieve the best performance under the same GPU hardware configuration.
FusionServer G5500 V7 features high performance, flexible architecture, high reliability, easy deployment, and simplified management. It accelerates applications such as AI training, AI inference, high-performance computing (HPC), image and video analysis, and database, and supports enterprise and public cloud deployment.
VENDOR SUPPLEMENT STATEMENTS ON STORAGE RESULTS (Unedited)
ANL
We evaluated the MLPerf Storage Benchmark on the Polaris supercomputer at the Argonne Leadership Computing Facility (ALCF), a US Department of Energy Office of Science User Facility. We assessed the performance of the storage system on Polaris for two distinct MLPerf Storage AI workloads: UNet3D and Bert.
Polaris is a HPE Nvidia, 44 petaflops system, which consists of 560 NVIDIA DGX A100 nodes interconnected with HPE Slingshot. Each node is equipped with 2 NVMe drives each of 1.60 TB. Eagle is a Lustre parallel file system residing on an HPE ClusterStor E1000 platform equipped with 100 Petabytes of usable capacity across 8480 disk drives. It has 160 Object Storage Targets and 40 Meta Data Targets with an aggregate transfer rate of 650 GB/s.
We ran the storage benchmark with datasets hosted on both the Eagle Lustre file system and node-local NVMe SSDs to emulate the behavior of how production users tend to use the system for AI workloads. Notably, our findings revealed a remarkable linear increase in I/O throughput for both UNet3D and Bert, as we scale to 2048 accelerators. The efficient I/O handling on Polaris allows data transfer to overlap with the computation, resulting in an impressively high accelerator utilization rate, close to 100%. For UNet3D, which is an I/O intensive workload, we observed a peak throughput of 200 GB/s when utilizing the Eagle parallel file system. When leveraging the node-local NVMe SSDs, the I/O throughput achieves 800 GB/s. In the case of Bert, which is not as I/O intensive as UNet3D, we observed the same ideal scaling pattern in I/O throughput. These outcomes clearly demonstrated that the storage system deployed at the ALCF supports efficient I/O operations for the state-of-the-art AI applications.
DDN
Organizations need a reliable and scalable storage platform to achieve their AI goals. DDN enables end-to-end Accelerated Computing with a simple but powerful appliance proven in the largest and most demanding AI deployments worldwide.
For the inaugural MLPerf storage benchmark, DDN is pleased to submit two configurations using the AI400X2 appliance in the CLOSED division to evaluate storage performance in typical small-scale machine learning deployments. The benchmark results measure the number of GPUs saturated by a single AI400X2 appliance during prescribed machine-learning tasks, the first using a single GPU compute system and the second with a cluster of GPU compute systems.
- In the single compute node benchmark, one DDN AI400X2 NVMe appliance running DDN’s EXAScaler 6.2 parallel filesystem served 40 accelerators at a throughput of 16.2 GB/s.
- In the multi-node benchmark, one DDN AI400X2 NVMe appliance served 160 accelerators across ten GPU compute nodes at a throughput of 61.6 GB/s.
- We note that this second benchmark submission was limited by the performance of the compute clients, rather than the single AI400X2 system, demonstrating the superior efficiency of the 2U appliance.
The AI400X2 appliances scale out linearly, adding performance or capacity to meet the requirements of the most ambitious AI projects.
We are excited to support the ongoing work of MLCommons to establish benchmarking best practices for AI/ML systems and allow the AI/ML community to make informed decisions based on standardized comparative measures. We look forward to future versions of the MLPerf Storage benchmark with additional workload models.
To learn more about DDN’s AI400X2, configurations, capabilities, and use cases, visit https://ddn.com/a3i
Micron
The Micron 9400 is designed to manage the most demanding data center workloads, particularly in artificial intelligence (AI) training, machine learning (ML) and high-performance computing (HPC) applications. The drive delivers an industry-leading 30.72 terabytes (TB) of storage capacity and 77% improved input/output operations per second (IOPS). The Micron 9400 is one of the world’s fastest PCIe Gen4 data center U.3 drive shipping and delivers consistently low latency at all capacity points.
Micron is proud to announce the first MLPerf storage benchmark results on the Micron 9400 NVMe SSD.
A single 7.68TB 9400 Pro is capable of supporting 17 accelerators with a throughput of 6.1 GB/s.
The Micron 9400’s capacity and performance enable larger datasets and accelerated epoch time leading to more efficient utilization of graphics processing units (GPUs).
While many SSDs are designed for pure read or write use cases, the Micron 9400 was designed with real-world applications in mind.
The Micron 9400 SSD is available in a U.3 form factor that is backwards-compatible with U.2 sockets and comes in capacities ranging from 6.4TB to 30.72TB. These options provide data center operators the flexibility to deploy the most energy efficient storage while matching their workloads with the right blend of performance, capacity and endurance.7 This versatile SSD is built to manage critical workloads whether in on-premises server farms or in a multi-tenant shared cloud infrastructure, and can be flexibly deployed in hyperscale, cloud, data center, OEM and system integrator designs.
Nutanix
The Nutanix Cloud Platform solution is a hybrid multicloud platform that provides a software stack to enable the full lifecycle of AI/ML applications. The consistent operating model helps enable ease of management whether data is being collected or fed into models for inferencing at the edge, or models are being fine-tuned at the core data center or in the public cloud.
Nutanix Files Storage provides distributed, scale-out file storage on the Nutanix Cloud Platform, with NFS and SMB support. With integrated cybersecurity and ransomware protection, Nutanix Files Storage provides high performance and low latency, along with native snapshots and disaster recovery.
Nutanix Objects Storage provides distributed, scale-out S3-compatible object storage on the Nutanix Cloud Platform. With integrated cyber resilience, encryption, and replication, Nutanix provides high-performance Objects Storage for cloud-native, analytics, AI/ML, and archive applications.
Nutanix is pleased to announce the first set of published results with the MLPerf storage benchmark.
Here are some highlights:
- We achieved 65 accelerators and a throughput of 25 GB/s with Unet3d ML training workload using five Nutanix NX-8170-G8 nodes with Nutanix Files Storage, using the standard NFS protocol
- We achieved 32 accelerators and a throughput of 13 GB/s with Unet3d ML training workload using four Nutanix NX-8150-G8 nodes with Nutanix Objects Storage, using the standard S3 protocol
- Delivered in our standard software-defined solution running Files Storage Version 4.3 on the Nutanix Cloud Platform running AOS Version 6.7 and AHV 9 hypervisor, and Objects Storage Version 4.0 on the Nutanix Cloud Platform running AOS Version 6.6.2.6 and AHV 9 hypervisor.
The benchmark was executed in a lab environment using conventional dual port 100Gb/s data center networking infrastructure. The setup could be expanded to up to 16 nodes to service more accelerators.
Weka
WEKA is a data platform software provider for AI and other performance-intensive workloads.
The WEKA Data Platform is purpose-built for modern data stacks in the cloud and AI era. It transforms stagnant data silos into dynamic data pipelines that power GPUs efficiently and fuel AI, ML, and HPC workloads seamlessly and sustainably. Its advanced, cloud-native architecture is optimized to solve complex data challenges at scale, delivering 10-100x performance improvements, whether running on-premises, in the cloud, at the edge and in hybrid and multicloud environments. The WEKA platform’s capability to support all types of IOs, reads/writes, small/large in a low latency fashion with massive metadata performance is the foundation for these performance accelerations, as well as its multiprotocol support, which eliminates multiple copying of the data throughout the AI pipelines. Additionally, the WEKA platform requires zero tuning and is auto-tuned for any IO pattern, allowing a mix of multiple AI IO patterns on the same datasets in the same filesystems, and scales linearly with the number of compute and storage instances for additional capacity and performance.
WEKA’s initial effort with this benchmark on a single host generated an industry-leading 7.3 GB/s serving 20 accelerators with the UNET3D model while being able to serve 24 accelerators for the IO-intensive BERT model with a throughput of 2.8MB/s). This single-client performance test was limited by the core and networking capabilities of the available client gear - a client with more cores could have supported more accelerators.
WEKA is committed to supporting the MLCommons Storage benchmark as it develops and looks forward to providing more extensive scale-out submissions in the future.