ORNL Unveils New AI Method for Quick Cancer Data Analysis, Enhancing National Reporting Efficiency
March 8, 2024 -- Artificial Intelligence researchers have taken a major step forward in using AI in the fight against cancer.
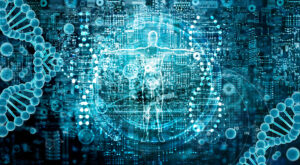
ORNL researchers are using attention mechanisms to maximize AI’s capabilities in the scanning of medical texts, which can drastically reduce the time needed for cancer incidence reporting. Credit: Shutterstock.
In partnership with the National Cancer Institute, or NCI, researchers from the Department of Energy’s Oak Ridge National Laboratory’s Modeling Outcomes for Surveillance using Scalable Artificial Intelligence, or MOSSAIC, are building on their groundbreaking work to circumvent the information log jam for nationwide cancer reporting.
With the use of advanced AI models, ORNL researchers have established a method of extracting valuable information from cancer pathology reports to streamline uploading data into the NCI database. This data extraction is critical for cancer researchers to better understand how communities are impacted by the disease and, by extension, for experts to develop and implement prevention strategies.
The research, published in the Journal of Biomedical and Health Informatics, details the team’s evaluation of various attention mechanisms, which train AI models through machine learning to focus only on the information needed. Attention mechanisms are commonly used in models performing the automated encoding of clinical documents, such as hospital discharge summary notes, with medical codes that are provided by the MIMIC-III database. The MOSSAIC team then uses the findings to improve the models performing the information extraction of crucial cancer data elements from electronic cancer pathology reports.
“We are developing models that read the raw cancer pathology reports to find useful information,” said Christoph Metzner, University of Tennessee doctoral student and member of the development team. “And in these reports, we find the information such as site, subsite, histology or the relative behavior of a cancer cell from a person.”
It can take as long as two years to extract and upload critical cancer data elements from pathology reports into population-level databases. With AI, that process could speed up to real time.
To improve the model’s efficiency, the diverse team used attention mechanisms to identify key words in the clinical documents and extract the needed information.
“To understand an attention mechanism, you have to imagine how your eye works,” Metzner said. “When you view your surroundings, your eye focuses on something and blurs out everything else. That’s how an attention mechanism works. They are successful in identifying a sequence of important, relevant words in cancer pathology reports for a specific cancer class.”
By comparing different attention mechanism strategies, the team was able to evaluate varying architectures such as convolutional neural networks, recurrent neural networks and transformers, in relation to the model’s performance, which helped to identify the optimal framework for locating useful information in the normally vast hospital discharge summary notes.
The study’s results on attention mechanisms, which focus on global word-level relationships, have enabled Metzner to explore ideas to increase the receptive field of attention mechanism to phrase-level, as opposed to word-level, context information. The experiments revealed that initializing label-wise attention with pretrained information improved the classification performance of all models.
By quantifying the outputs of different types of text-encoder architectures with differing attention mechanisms, the team hopes their research will assist other machine learning researchers in their quest to advance medicine via the automated analysis of medical texts.
These experiments were performed on the MIMIC III dataset using the Oak Ridge Leadership Computing Facility Summit supercomputer and the Compute and Data Environment for Science at ORNL. The project was sponsored by ASCR BrAVE and the NCI-DOE MOSSAIC project, and led by Heidi Hanson, group leader of Biostatistics and Biomedical Informatics at ORNL. OLCF is a DOE Office of Science user facility.
Source: Mark Alewine, ORNL