Industrial AI: A Critical Tool for Complex Energy Systems
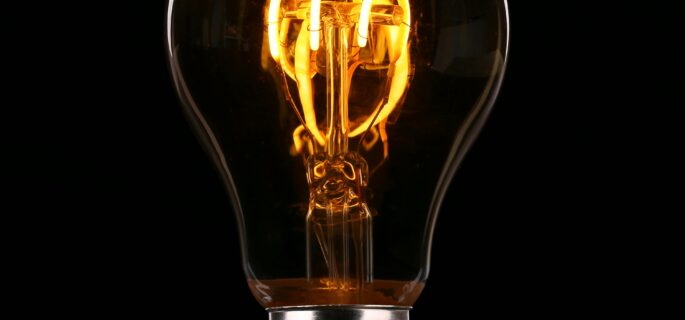
From oil and gas to power and utilities, energy companies around the globe have seen their businesses become more complex as the world works to navigate the energy transition. To better manage those changes, many have turned to a wide range of digital tools, including artificial intelligence (AI).
When it comes to the energy industries, however, not all AI is the same.
Asset-intensive companies – especially energy companies – are turning to what’s considered “industrial AI.” Industrial AI is built for the intricacies of complex energy systems and is equipped with specific guardrails designed to ensure it behaves in predictable ways. Industrial AI doesn’t take unexpected actions that might damage equipment or put workers and the community at risk.
For companies that properly apply industrial AI, the benefits are often significant.
Optimizing sustainability efforts
While there are many areas where AI can benefit the energy industries, one of the most important is helping companies embrace the energy transition.
In recent years, AI has been used to help utilities forecast renewable energy production, allowing operators to plan for when and how to switch to traditional generation sources if renewable production fluctuates as the weather changes. AI forecasting allows operators to better balance the grid and ensure operations remain stable while also bringing new renewable generation sources online.
The energy industry is also using AI to replace outdated and manual processes for emission monitoring, instead using automation to identify, and remediate, areas of excess emissions or leaks across plants. AI can also be used to help model and select new sustainability pathways, like hydrogen & carbon capture, as the energy industry accelerates progress toward net zero goals. By helping to make accurate predictions of the technical and economic feasibility of a new sustainability project, AI has proven to be a useful tool to mitigate risk and lower CAPEX & OPEX.
Tracking equipment health
Industrial AI can even help companies more precisely understand the condition of their assets and adjust operations to ensure they don’t over-stress equipment or undergo an unexpected breakdown.
Electrical grid operators use AI tools to track the condition of transformers, which typically degrade over time due to partial discharge and other issues. Overloading transformers can accelerate that process, so utilities use AI tools to monitor the status and health of each transformer. This allows operators to efficiently schedule maintenance, extending the life of equipment while also avoiding potentially costly breakdowns. If energy systems do break down, utility operators could turn to industrial AI tools to automatically optimize repair crew schedules based on different criteria.
In the example of an oil & gas company, predictive & prescriptive maintenance backed by AI uses vast amounts of data to monitor critical assets at refineries. It learns patterns of behavior and alerts the organization to impending failure, which could also release excess emissions into the atmosphere, so they can remediate the situation before it happens.
Why guardrails are critical for industrial AI
Certainly, it’s easy to find ways industrial AI can improve or optimize operations for energy companies, from reliably automating systems to identifying the most efficient way to pursue new sustainability pathways.
As they take on those challenges, however, it is critical industrial AI systems have built-in guardrails that ensure they don’t produce confusing or incorrect results that might damage assets. One of the clearest examples of why those guardrails are needed can be found in advanced process control (APC) technology. Traditional APC systems are built around static models, continuously applying the same solutions until an operator manually changes it. Industrial AI allows systems to dynamically optimize processes as conditions change, resulting in significantly improved outcomes.
Allowing AI systems to make those changes automatically, however, opens the door to significant risks if the algorithm makes the wrong decision. By building guardrails into the system – such as mandating operator intervention in some situations or restricting an algorithm’s possible responses – operators can trust that the AI won’t accidentally trigger a runaway process that could damage equipment or lead to other problems.
In other cases, those guardrails play a key role in ensuring plant operations run efficiently. Without guardrails, AI models could inadvertently violate laws of physics when optimizing poor-performing processes. Thus, the AI would propose infeasible solutions that are not optimal and potentially cause large production losses.
As they look ahead, energy companies are facing a period of unprecedented uncertainty. How the world will adapt to the energy transition, what the energy mix of the future will look like and how decarbonization efforts will go forward are all – to some degree – open questions.
Industrial AI represents a powerful tool that can help companies make sense of those questions. It will take careful management and planning, but when properly applied, AI will be key in making the energy economy of the future a reality.
Heiko Claussen is Senior Vice President at Aspen Technology, leading the AI Shared Services group. As part of the Technology organization, Heiko is responsible for Industry 4.0 strategy, AI research, and shared services to foster synergies and innovation throughout the company. Prior to Aspen Technology, he was Head of Autonomous Machines and Principal Key Expert of AI at Siemens and led initiatives to enable autonomous machine applications for factory automation. During his 15-year tenure at Siemens, Heiko worked in many areas related to AI and digitization, including remote monitoring, machine learning, robotics, pattern recognition and statistical signal processing. Heiko is a recipient of numerous technical awards and recognitions.
He has been named Inventor of the Year twice at Siemens – in 2016 for the development of a virtual sensor to monitor the flame status of gas turbines, and in 2019 for the development of a neural net accelerator for industrial control systems. He is also the author of over 100 registered inventions with 67 granted/published patents. Heiko holds a Ph.D. degree in Electrical Engineering from the University of Southampton, UK; a master’s degree in Electrical Engineering from the University of Ulster, UK; and a Dipl.-Ing degree in Electrical Engineering from the University of Applied Sciences Kempten, Germany.