Berkeley Lab Researchers Harness Power of AI for Plant Root Analysis
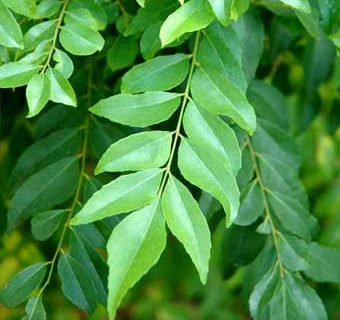
Roots play a pivotal role in the plant’s life cycle, including its water uptake, nutrient absorption, soil interaction, stability, and adaptation to changing environmental conditions. Despite significant progress in plant science, researchers have struggled to fully understand the intricate structures, growth dynamics, and responses to environmental stresses of the hidden half of living plants.
In an investigation to boost agricultural yields and develop plants resistant to climate change, Berkeley Lab researchers have introduced RhizoNet, a computational tool that harnesses the power of AI to transform plant root analysis and empower scientists to uncover new insights about root behavior under various environmental conditions.
The research was conducted by Lawrence Berkeley National Laboratory’s (Berkeley Lab’s) Applied Mathematics and Computational Research (AMCR) and Environmental Genomics and Systems Biology (EGSB) divisions.
The AI-powered tool, detailed in a paper published in Scientific Reports, is designed to automate the root analysis process to deliver unprecedented accuracy. It uses an advanced deep learning approach based on a convolutional neural network, allowing it to segment plant roots for a comprehensive biomass and growth assessment.
Traditional methods for plant root analysis, such as those that rely on flatbed scanners and manual segmentation methods, are labor-intensive and prone to human error. They also limit the ability of scientists to capture the fine details of root growth and behavior, especially for complex root systems. Now with RhizoNet, researchers have a tool to track root growth and biomass with greater precision.
“The capability of RhizoNet to standardize root segmentation and phenotyping represents a substantial advancement in the systematic and accelerated analysis of thousands of images. This innovation is instrumental in our ongoing efforts to enhance the precision in capturing root growth dynamics under diverse plant conditions,” said Daniela Ushizima, lead investigator of the AI-driven software, Berkeley Lab.
The key challenges in plant root analysis is the intricate nature of root structure and the presence of “noisy backgrounds” such as bubbles, droplets, reflections, and shadows that can complicate the root image segmentation. In some cases, the fine structures are only as wide as a pixel making it extremely challenging for even the best human annotators.
To address this issue, RhizoNet is reinforced by the latest version of EcoFAB, a unique hydroponic device that facilitates in-situ plant imaging. This device was developed by the EGSB, the DOE Joint Genome Institute (JGI), and the Climate & Ecosystem Sciences division at Berkeley Lab. EcoFAB can provide detailed imaging of root systems, eliminating the complexities of manual annotation and traditional imaging methods.
The Scientific Reports paper illustrates how the Berkeley Lab researchers used RhizoNet and EcoFAB in the analysis of root scans of Brachypodium distachyon plants under different conditions over five weeks. The high-throughput nature of EcoBOT, the new image acquisition system for EcoFABs, enabled the researchers to perform systematic experimental monitoring.
“We’ve made a lot of progress in reducing the manual work involved in plant cultivation experiments with the EcoBOT, and now RhizoNet is reducing the manual work involved in analyzing the data generated,” noted Peter Andeer, a research scientist in EGSB and a lead developer of EcoBOT, who collaborated with Ushizima on this work. “This increases our throughput and moves us toward the goal of self-driving labs.”
The accuracy and efficiency of RhizoNet are poised to drive research efforts toward more efficient and insightful plant studies. However, the new technology is not without challenges.
There are concerns about the standardization of data interpretation generated by AI algorithms. Researchers will need to ensure the reproducibility and accuracy of the AI model across different setups and plant species. In addition, they will have to address the need for continuous validation and optimization to maintain the tool’s efficacy over time.
While there are challenges in the use of RhizoNet in various settings, it still represents a paradigm shift in plant roof analysis. Researchers are now equipped with a powerful tool to explore the hidden dimensions of root biology. It could lead to solutions for improved crop productivity, climate resilience, sustainable agriculture, and other benefits.
“Our next steps involve refining RhizoNet’s capabilities to further improve the detection and branching patterns of plant roots,” said Ushizima. "We also see potential in adapting and applying these deep-learning algorithms for roots in soil as well as new materials science investigations.”
Related Items
AI Saves the Planet this Earth Day
Going Green in the AI Era: an Imperative for Performance-Intensive Applications