Anthropic Pushes for Third-Party AI Model Evaluations
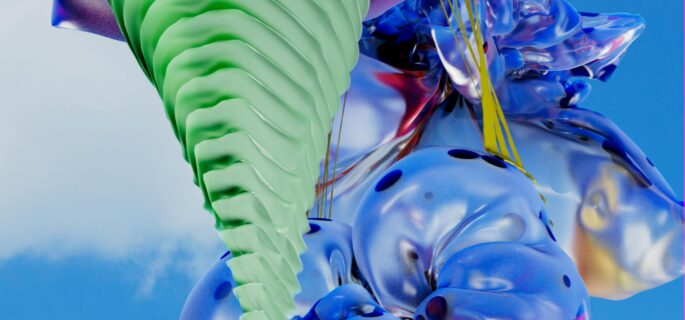
Although AI tools are rapidly advancing and becoming a part of just about every sector, the AI community is still looking for a standardized means to assess the capabilities and potential risks that these tools offer. Although tools like Google-Proof Q&A exist to provide a foundation for assessing AI capabilities, current evaluations are generally too simplistic or have solutions readily available online.
Thus, Anthropic has recently announced a new initiative for developing third-party model evaluations to test AI capabilities and risks. An in-depth blog post from the company outlined the specific types of evaluations Anthropic is prioritizing, and readers are asked to send in a proposal for new evaluation methods.
Anthropic outlined three ikey areas of evaluation development that they will be focusing on:
- AI Safety Level assessments: Evaluations are meant to measure AI ASafety Levels (ASLs) to include focuses on cybersecurity; chemical, biological, radiological, and nuclear (CBRN) risks, model autonomy, national security risks, social manipulation, misalignment risks, and more.
- Advanced capability and safety metrics: Measurements of advanced model capabilities like harmfulness and refusals, advanced science, improved multilingual evaluations, and societal impacts.
- Infrastructure, tools, and methods for developing evaluations: Anthropic wants to streamline the evaluation process to be more efficient and effective by focusing on templates/No-code evaluation development platforms, evaluations for model grading, uplift and uplift trials.
In the hopes of spurring creative discussion, Anthropic also provided a list of characteristics that the company believes should be inherent in a valuable evaluation tool. While this list covers a wide variety of topics, there were some specific points of interest.
To begin, evaluations should be sufficiently difficult to measure the capabilities for levels ASL-3 or ASL-4 in Anthropic’s Responsible Scaling Policy. In a similar vein, the evaluation should not include training data.
“Too often, evaluations end up measuring model memorization because the data is in its training set,” the blog post stated. “Where possible and useful, make sure the model hasn’t seen the evaluation. This helps indicate that the evaluation is capturing behavior that generalizes beyond the training data.”
Additionally, Anthropic pointed out that a meaningful evaluation tool will comprise a variety of formats. Many evaluation tools focus specifically on multiple choice, and Anthropic states that other formats such as task-based evaluations, model-graded evaluations, or even human trials would help in truly evaluating an AI model’s capabilities.
Finally, and perhaps most interestingly, Anthropic states that realistic, safety-relevant thread modeling will be vital to a useful evaluation. Experts should ideally be able to conclude that a major incident could be caused by a model with a high score in a safety evaluation. When models perform well, experts have typically come to the conclusion that this is not reason for concern, even when the models perform well on that particular version of the evaluation. This does not allow for a proper evaluation.
At the moment, Anthropic is asking for proposals from those who wish to submit evaluation methods. The Anthropic team will review submissions on a rolling basis and follow up with certain proposals to discuss the next steps.