Aurora Atmosphere Model is 5,000x Faster Than Traditional Systems
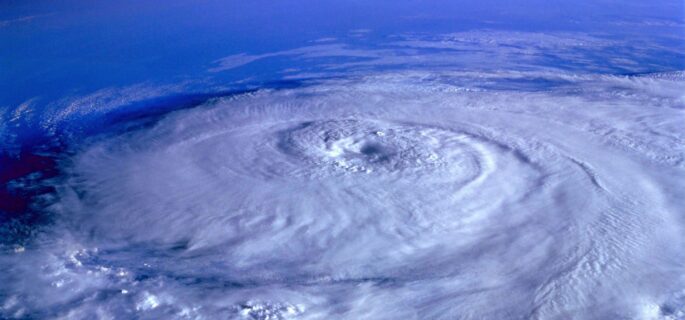
While the onset of human-driven climate change brings with it many horrors, the increase in the frequency and strength of storms poses an enormous threat to communities across the globe.
As climate change is warming oceans and pushing the sea to rise by more than six inches since 1900, storms will only become more intense. The proportion of Category 3 or above storms has doubled in the Atlantic Ocean since 1980 and these hurricanes are three times more likely than 100 years ago.
Prevention of climate change by lessening the amount of human-expelled carbon in the atmosphere is the only true solution to these problems.
However, a recent atmosphere model from Microsoft may help us better prepare for these storms. Called Aurora, this solution is the first large-scale foundation model of the Earth’s atmosphere that can work with enormous amounts of atmospheric data.
Microsoft asserts that Aurora's training on more than a million hours of meteorological and climatic data has resulted in a 5,000-fold increase in computational speed compared to numerical forecasting Integrated Forecasting Systems.
To learn more about this system, I spoke with Paris Perdikaris – the Principal Research Manager at Microsoft Research AI4Science.
“The results of Aurora indicate that just by two factors, just increasing the data set diversity and also the model size, you can get improved accuracy,” Perdikaris said. “Both in predicting typical weather events, but also more importantly, improved accuracy for extreme events.
With Aurora’s promising capabilities in mind, let’s delve deeper into how this system could forever alter our approach to storm prediction and preparedness.
Why AI?
Perdikaris began our talk by outlining the two current weather prediction models scientists can use. First, the traditional weather and climate models that we’ve used for years aim to predict how the atmosphere evolves.
They do so by formulating a set of governing equations using physical principles, such as the conservation of mass and energy. When these equations are formulated, researchers then use large supercomputers to simulate the solutions.
“To generate a ten-day weather forecast, that supercomputer runs for a few hours to give that prediction,” Perdikaris said. “And in this process, there needs to be a step called data simulation, where the outputs of those simulators are calibrated to be in closer agreement with the real observations we’re collecting. It’s a long and expensive process.”
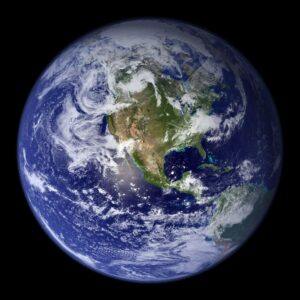
From satellites to weather balloons, scientists have collected an enormous amount of data on Earth weather patterns.
The second class of prediction models are the AI-based models that Aurora falls under. Perdikaris pointed out that the Earth is an extremely unique system in that it has been monitored around the clock by satellites, weather stations, weather balloons, and more. There is a wealth of data about our planet that is primed for AI exploration and use. Perdikaris stated that these systems can tap into this information and build purely data-driven prediction systems. They’re not directly leveraging the physics equations of atmospheric dynamics, and as such these systems are extremely fast in their predictions.
Although Perdikaris mentions that systems like Aurora are expensive during their training phase because they need to learn all about weather events over the last decade, this upfront cost pays dividends down the road.
“Once you have trained the system, now in a matter of a second you can get the same ten-day forecast that the traditional prediction tool would need a supercomputer running for a couple of hours to give you,” Perdikaris said. “This is the main advantage and the promise of AI methods, which is the enhanced efficiency and computational speed.”
However, Perdikaris also mentioned a second advantage that is a little more subtle. He pointed out that AI methods are agnostic of what data sources are used to train them. Scientists can train them on simulation data, but they can also train them using real observation data – or even a combination of the two, which is called reanalysis data. Reanalysis data reflects our most accurate understanding of the atmosphere because they combine the outputs of physics-based models and real observations.
By training these machine learning models on reanalysis data, scientists get a product at the end that can give more accurate predictions than a purely physics-based model.
How is Aurora Different?
This all explains the advantages of AI-based atmospheric models over traditional physics-based models, but it does not address exactly why Aurora is superior to other AI methods. To do so, lets compare Aurora to the GraphCast AI weather forecast model from Google DeepMind.
Aurora
- 1.3 billion parameters
- Uses a flexible 3D Swin Transformer with 3D Perceiver-based encoders and decoders
- Can operate at 0.1° spatial resolution (roughly 11km squared at the equator.)
- Trained on over a million hours of diverse weather and climate simulations
GraphCast
- 36.7 million parameters
- Uses a graph neural network architecture
- Can operate at .25° spatial resolution (roughly 28km squared at the equator.)
- Trained on ERA5 reanalysis data
There are some obvious differences at first glance here. Aurora has a vastly larger parameter size than GraphCast, and uses the 3D Swin transformer adaptation from the 2d Shifted Window Transformer concept. Also, Aurora’s resolution size is improved over GraphCast’s.
On top of these specs, Microsoft’s Aurora has shown a massively superior performance over GraphCast. Aurora outperforms GraphCast on 94% of targets and shows a 40% improvement over GraphCast in the upper atmosphere. What’s more, Aurora demonstrates 10-15% improvements at short and long lead times.
One of the main differences that sets Aurora up for success is how it is trained. Microsoft stated that Aurora was trained on over a million hours of diverse weather and climate simulations. On the other hand, Perdikaris said that GraphCast relies solely on the ERA5 global climate reanalysis dataset.
“GraphCast and all these other AI systems were primarily trained on that single data set, and they were designed to tackle a single prediction task, which is ten-day weather forecasting,” Perdikaris said. “Now with Aurora, we try to sort of investigate the hypothesis that I mentioned earlier of what happens if we go beyond using a single data set to train those models.”
Perdikaris continues: “With Aurora, we asked the question ‘ what happens if we go beyond this ERA5 data set’, which is also a scale of a couple of terabytes or dozens of terabytes. What happens if we start using reanalysis data like ERA5, but also forecast data analysis data and increase the diversity of the sources of the data we're training on to go all the way up to a few hundred terabytes or maybe even up to a petabyte of training data. The results of Aurora indicate that just by those two factors – just increasing the data set diversity and volume, and also the model size, you can get improved accuracy both in predicting typical weather events, but also improved accuracy for extreme events.”
Perdikaris said that many of the design principles behind Aurora were precisely made to be able to accommodate different data sources, different variables, and different resolutions. Therefore, the team needed a very flexible model architecture to process all these different data streams.
This diverse set of data is one of the reasons Aurora is about 5,000 times faster than the Integrated Forecasting System while GraphCast is only 1,000 times faster.
The combination of Aurora’s advanced architecture, higher resolution, and diverse training data underscores its superior performance in atmospheric modeling.
Simulating Air Pollution
Beyond its impressive weather-prediction capabilities, Aurora also has great potential in forecasting air pollution levels. The model uses data from the Copernicus Atmosphere Monitoring Service (CAMS) to make these air pollution predictions, which are notoriously hard for computational methods to predict.
This difficulty comes from the fact that scientists have to simulate additional physics to produce an accurate air pollution prediction. The scientist wants to predict meteorological variables like wind velocity, temperature, and pressure. But on top of that, the scientists have to simulate atmospheric chemistry. This includes a new set of variables that describes concentrations of different chemicals – all of which interact with each other.
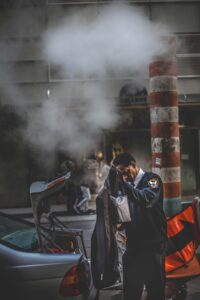
Modeling of air pollution will play a vital role in protecting humanity from the various chemicals we pump into the air
“Going back, I mentioned that a ten-day weather forecast takes a few hours on a supercomputer,” Perdikaris said. “If you want to add on top of that atmospheric chemistry that enables you to predict air pollution, then those simulations are ten times more expensive than plain weather simulations.”
This difficulty for traditional methods is a boon to the new Aurora model. Aurora can produce accurate five-day global air pollution forecasts at .4° of spatial resolution. What’s more, the model outperforms state-of-the-art atmospheric chemistry simulations on 74% of all targets for air pollution forecasting. Aurora accurately predicts a broad range of atmospheric variables, such as the concentrations of greenhouse gases and nitrogen dioxide.
While the climate crisis will involve hard work from many members of society, the ability to predict and track both weather patterns and air pollution will only increase in importance as the years pass. Aurora shows the valuable role AI will play in the fight against climate change, and its current capabilities make it seem to be a powerful tool.