Harnessing Physics for Remote Sensing Precision: PNNL’s AI Breakthrough
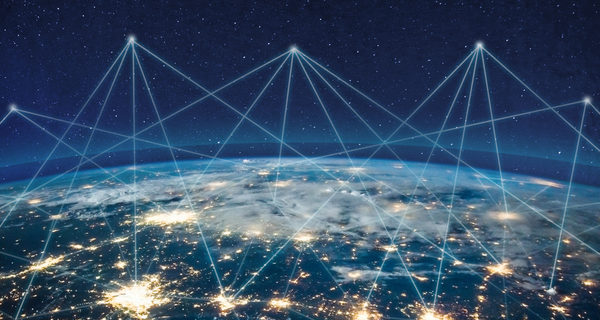
Image credit: Shutterstock
Scientists observe Earth and other planetary bodies via remote sensing satellites that detect and record reflected energy. However, as sunlight travels in space it interacts with various atmospheric components, such as water vapor, ozone, turbulence, and temperature changes. These interactions can distort the spectral data received by the satellites.
Earth observation scientists understand this phenomenon and have developed several correction methods to mitigate the influence of atmospheric interactions. However, traditional correction methods, such as dark object subtraction or empirical line calibration, have limitations and may not fully capture the complexity of atmospheric interactions.
James Koch, a data scientist at Pacific Northwest National Laboratory (PNNL), has developed a new method to address the problem using physics-informed machine learning. This method uses AI to enhance the remote sensing capabilities of the satellites.
The development of the new method was supported by the National Security Directorate’s Laboratory Directed Research and Development portfolio and is set to enhance PNNL’s remote explorations capabilities. Last week, Koch presented a paper detailing this innovative method at the International Geoscience and Remote Sensing Symposium in Athens, Greece
While scientists are equipped with tools and knowledge on how to apply atmospheric corrections to the data received by the sensors, they rely on having an atmospheric transmission profile. This profile is a detailed characterization of how the light interacts with the atmosphere at different wavelengths as it passes through various altitudes.
The complexity and variability of the atmosphere make it challenging to obtain this profile. However, Koch’s AI technique can generate this profile without needing extensive prior knowledge, and this is where it has the potential to be a game changer.
Most atmosphere correction applications have off-the-shelf tools that use generic atmospheric profiles. While these may be sufficient for urgent applications, such as disaster response, they do not deliver the precision required for target detection and other applications where high accuracy is paramount.
“The key benefit here is we can get good accuracy with a limited amount of data while not having to rely on a lot of prior knowledge in the sense of where the sensor was, or where the sun was,” said Koch. “We’re learning those things on the fly.”
According to Koch, he used a data-informed approach to the problem combining what subject matter experts do into a machine learning pipeline. This allowed him to automate the sophisticated techniques used by atmosphere science experts.
For training the ML model, Koch used data from the overhead imagery of Cook City, MT. The dataset included several spectral signatures, including vehicles. The ML model was trained to take pixels from any spectral scene to infer an atmosphere transmission profile and automatically perform the required atmospheric corrections.
Koch refers to the constraints of the differential equations as the “secret sauce” of his model as it allows the predictions to be guided by underlying fundamental laws and principles of physics.
A unique capability of Koch’s model is that it is bidirectional, which means it can be used for atmospheric correction and also for material appearance inference to predict how different atmospheric conditions might affect the observed characteristics of materials or objects.
The rapid advances in AI capabilities have been transformative in enhancing scientific research and discovery. From proving the existence of a rare phase of matter to the discovery of new drugs, AI has revolutionized the speed and accuracy of scientific breakthroughs.
The method developed by Koch represents a significant advancement in the field of atmospheric correction. It offers greater flexibility in adapting to various atmospheric conditions while reducing the cost and resources required for obtaining accurate remote sensing data.
It also expands the utility and impact of remote sensing technologies by making them more accessible and efficient in various applications, from environmental monitoring to disaster management and beyond.
Related Items
AI-fueled Geospatial Analysis Might Change the World. And Save It
International Team of Researchers Advance Groundwater Resilience Through AI and Data Science
Scientists Use GenAI to Uncover New Insights in Physics