Google’s DataGemma Tackles AI Hallucination
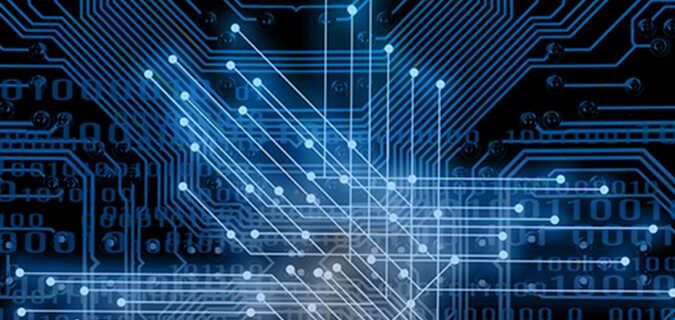
The rapid evolution of large language models (LLMs) has fueled significant advancement in AI, enabling these systems to analyze text, generate summaries, suggest ideas, and even draft code. However, despite these impressive capabilities, challenges remain, particularly with the phenomenon of "hallucination," where LLMs deliver factually incorrect or nonsensical output.
To address this issue, Google has enhanced the accuracy and reliability of its AI models with the launch of DataGemma last week. This innovative approach anchors LLMs in the extensive, real-world statistical data of Google's Data Commons. Building on the advanced research and technology of Gemini models, DataGemma improves LLM accuracy and reasoning by connecting the LLMs to Data Common’s real-world data.
Rather than requiring familiarity with the specific data schema or API of the underlying datasets, DataGemma leverages the natural language interface of Data Commons to query and retrieve information.
Serving as a foundation for factual AI, Data Commons is Google’s publicly accessible knowledge graph, featuring over 250 billion data points across hundreds of thousands of statistical variables sourced from trusted organizations.
At its core, the DataGemma series utilizes Gemma 2 27B, an open-source large language model introduced by Google in June. This model, built on the widely adopted Transformer neural network architecture, boasts 27 billion parameters. Google claims the model delivers performance capable to LLMs with twice as many parameters.
Google’s new approach relies on retrieval-augmented generation (RAG), a method that is increasingly adopted by businesses. With the introduction of DataGemma, Google seeks to bring this method into the AI mainstream.
RAG has transformed the industry by leveraging retrieval-based techniques that dynamically fetch and integrate contextually relevant external data into the generation process, thereby enhancing accuracy and minimizing hallucinations.
Google’s move to integrate RAG with Data Commons represents the first large-scale cloud-based implementation of RAG. While many enterprises have employed RAG with proprietary data, Google’s application of a public resource like Data Commons represents a significant advancement. This approach aims to improve AI reliability and functionality by utilizing high-quality and verifiable data.
According to Google, DataGemma takes a two-pronged approach to integrate data retrieval with LLM output. The first method is to use RAG to retrieve relevant contextual information and necessary facts upfront, allowing the model to deliver more comprehensive and accurate answers.
The second method is to use retrieval-interleaved generation (RIG) to fetch specific statistical data in real time to fact-check questions posed in the query prompt. The collected information is sent to Google’s more advanced, proprietary Gemini 1.5 Pro model, which generates an output.
By employing these two distinct approaches, factual accuracy and transparency are improved, reducing the occurrence of hallucinations. Additionally, it provides users with the sources for the information.
DataGemma is currently available only to researchers, but Google plans to expand access after further testing. While it shows promise, there are a few caveats to consider.
One of the key limitations of DataGemma is whether the data needed for RAG and RIG approaches is available in the Data Commons. Google researchers found that in 75% of test cases, the RIG method couldn’t retrieve any useful information from Data Commons. In some cases, Data Commons contains the needed information, but the model does not formulate the right command to find it.
Another challenge is related to accuracy. The RAG method gave incorrect answers 6-20% of the time, while the RIG method only pulled stats from Data Commons about 58% of the time.
Although these numbers may seem modest, they reflect a notable improvement over Google’s LLMs that do not access Data Commons. Google plans to improve the model by training it on more information and increasing its question-answering capacity from a few hundred to millions.
“While DataGemma represents a significant step forward, we recognize that it's still early in the development of grounded AI,” Google software engineer Jennifer Chen and Prem Ramaswami, the head of Data Commons, detailed in a blog post.
“We invite researchers, developers, and anyone passionate about responsible AI to explore DataGemma and join us in this exciting journey. We believe that by grounding LLMs in the real-world data of Data Commons, we can unlock new possibilities for AI and create a future where information is not only intelligent but also grounded in facts and evidence.”
The introduction of DataGemma indicates that Google and other AI companies are moving beyond the basic capabilities of large language models. The future of AI depends on its capacity to integrate with external data sources, including public databases or proprietary corporate information.