AI Has a Data Problem, Appen Report Says
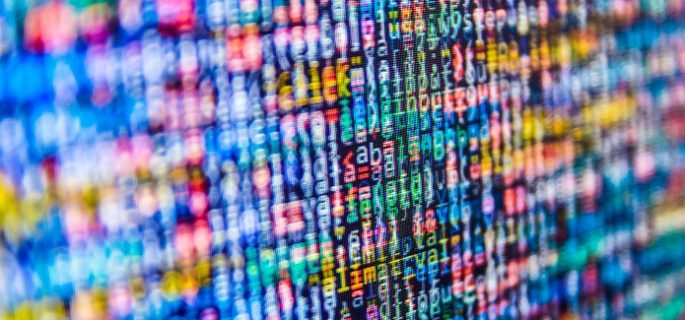
AI may be a priority at American companies, but the difficulty in managing data and obtaining high quality data to train AI models is becoming a bigger hurdle to achieving AI aspirations, according to Appen’s State of AI in 2024 report, which was released yesterday.
AI is dependent on data. Whether you’re training your own AI model, fine tuning someone else’s model, or using RAG techniques with a pre-built model, successful deployment of AI requires bringing data to the table–preferably lots of clean, high-quality data.
As a provider of data labeling and annotation solutions, Appen has a front row seat to the data sourcing challenges that organizations run into when building or deploying AI solutions. It has documented these challenges in its annual State of AI reports, which is now in its fourth year.
The data challenges of AI have reached new lows according to the company’s State of AI in 2024 report, which is based on a survey it commissioned Harris Poll to conduct of than 500 IT decision-makers at US firms earlier this year.
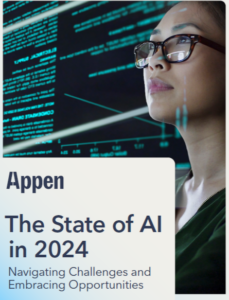
You can download the Appen State of AI in 2024 report here
For instance, the average accuracy of data reported by survey-takers has declined by 9 percentage points over the past four years, according to the report. And the lack of data availability has risen by 6 percentage since the company released the State of AI report for 2023.
The drop in quality and availability may be due to a shift away from simpler machine learning projects build on structured data towards more complex generative AI projects built on unstructured data over the past two years, says Appen Vice President of Strategy Si Chen.
“We see a lot of data now that’s unstructured. It’s not very standardized,” Chen tells BigDATAwire. “They often require lots of domain expertise and subject matter expertise to actually go and build those data sets. And I think that’s the reason that we see causing some of that decline in terms of data accuracy. It’s just because the data that people want and need nowadays is just much more complex data than it used to be.”
In its report, Appen also picked up on an emerging bottleneck when it comes to the AI data pipeline. Companies are struggling to succeed at multiple steps, whether it’s getting access to data, being able to appropriately manage the data, or having the technical resources to work with the data. Overall, Appen is tracking a 10 percentage point increase in bottlenecks related to sourcing, cleaning, and labeling data since 2023.
While it’s hard to pinpoint a single cause of that decline, Chen theorizes that one of the leading causes could be a general increase in the types of AI initiatives that organizations are embarking upon.
“A lot of it could be related to the fact that there’s just more diverse use cases that are being designed and developed,” she says, “and each specific use case that you design from an enterprise will require custom data to actually go and support that use case.”
Appen is a giant in the data annotation and labeling space, with nearly three decades of experience. While GenAI is fueling a surge in the need for high quality training data at the moment, Appen recognizes that every individual project requires its own unique data set to train on, which is the company’s specialty. The figures coming out of Appen’s State of AI report indicate that many organizations are struggling with that.
“There’s just more diverse use cases that are being designed and developed, and each specific use case that you design from an enterprise will require custom data to actually go and support that use case,” says Chen, who joined about Appen a year ago after stints working in AI for Tencent and Amazon.
“So all of that diversity means that to go and actually build those models, you need to make sure you have a really robust data pipeline to enable you to go and set that up,” she continues. “There’s a whole series of steps revolving around data for every individual use case. And so as more people are deploying more of these models, maybe they’re stumbling across the fact that all of this is not necessarily mature in their existing data pipelines.”
Organizations that developed these data pipelines and skills to develop traditional machine learning applications on structured data are finding that developing generative AI applications using unstructured data requires a different type of data pipeline and different skills, Chen says.
“I think that’s going to be a bit of a transition period,” she says. “But it’s very exciting.”
Appen’s survey concludes the adoption of GenAI use cases went up 17% from 2023 to 2024. This year, 56% of the organizations it surveyed having GenAI use cases. The most popular GenAI use case is for boosting the productivity of internal business processes, with a 53% share, while 41% say they’re using GenAI to reduce business costs.
As GenAI ramps up, the percent of successful AI deployments goes down, Appen found. For instance, in its 2021 State of AI report, Appen found an average of 55.5% of AI projects made it to deployments, a figure that dropped to 47.4% for 2024. The percentage of AI projects that have found a “meaningful” return on investment (ROI) has also dropped, from 56.7% in 2021 to 47.3% in 2024.
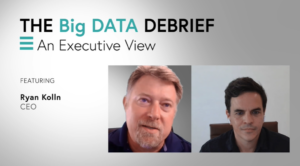
Appen CEO Ryan Kolln recently appeared on the Big Data Debrief
Those figures reflect data challenges, Chen says. “Even though there’s a lot of interest and people are working on lots of different use cases, there are still a lot of challenges in terms of getting to deployment,” she says. “And data is playing a pretty central role into whether something can be successfully deployed.”
There are three broad types of data that organizations are using for AI, according to the report. Appen found 27% of uses cases are using pre-labeled data, 30% are using synthetic data, and 41% are using custom-collected data.
The capability to employ custom-collected data that nobody has seen before provides a strong competitive advantage, Appen CEO Ryan Kolln said on a recent appearance on the Big Data Debrief.
“There’s a large amount of publicly available data out there, and that is being consumed by all the model builders,” he said, “But the real competitive advantage with generative AI is the ability to access bespoke data. What we’re seeing is it’s a very competitive approach around how to you go and find bespoke data. and we’re seeing real-world, human -collected data being important part of that data corpus.”
You can read Appen’s State of AI in 2024 here.
This article first appeared on sister site BigDATAwire.
Related
Alex Woodie has written about IT as a technology journalist for more than a decade. He brings extensive experience from the IBM midrange marketplace, including topics such as servers, ERP applications, programming, databases, security, high availability, storage, business intelligence, cloud, and mobile enablement. He resides in the San Diego area.