OpenAI Develops New AGI Benchmark to Assess Potential Risks of Advanced AI
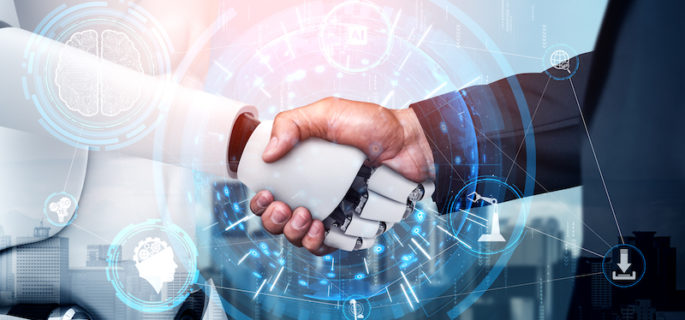
As artificial intelligence systems grow more advanced, there is increasing concern over models capable of modifying their own code and enhancing their abilities without human oversight. Such AI agents, if left unchecked, could progress at a rate that surpasses human understanding, potentially leading to unpredictable or even catastrophic outcomes.
Researchers from OpenAI have developed MLE-bench to assess how effectively AI models can perform tasks in “autonomous machine learning (ML) engineering.” This new artificial general intelligence (AGI) benchmark consists of a series of tests designed to measure whether AI agents can modify their own code and improve their capabilities without human instruction. AGI represents an AI system with intelligence surpassing human capabilities
The MLE-bench consists of 75 Kaggle tests, also referred to as Kaggle datasets, which serve as rigorous challenges to evaluate the ML engineering capabilities of AI models. Each sample of the Kaggle dataset was manually sourced from Kaggle to reflect a core set of day-to-day skills that ML engineers use in advanced research and development environments.
The Kaggle tests represent real-world challenges, such as the Stanford OpenVaccine initiative, which focuses on developing an mRNA vaccine for COVID-19, and the Vesuvius Challenge, aimed at deciphering ancient scrolls from a library in Herculaneum, a town next to Pompeii.
The researchers tested MLE-bench with OpenAI’s most powerful AI model, the o1. The results indicate that the AI model achieved at least a Kaggle bronze medal level on 16.9% of all of the 75 tests in MLE-bench. This percentage increased as the model was given more opportunities to take on the challenges.
Achieving a bronze medal means ranking among the top 40% of human participants on the Kaggle leaderboard. OpenAI's o1 model earned a total of seven gold medals on MLE-bench, which is two more than what is required for a human to be considered a "Kaggle Grandmaster." The researchers noted in their paper that only two humans have ever achieved medals in the 75 different Kaggle competitions.
The OpenAI researchers emphasize that AI agents could have numerous positive impacts, especially in accelerating scientific research and discovery, however, if the AI agents are not controlled, it could lead to unmitigated disaster.
"The capacity of agents to perform high-quality research could mark a transformative step in the economy. However, agents capable of performing open-ended ML research tasks, at the level of improving their own training code, could improve the capabilities of frontier models significantly faster than human researchers," the researchers explained in their paper published on arXiv.
"If innovations are produced faster than our ability to understand their impacts, we risk developing models capable of catastrophic harm or misuse without parallel developments in securing, aligning, and controlling such models.”
Highlighting the limitations of MLE-bench, the researchers pointed out the risk of contamination. As Kaggle competitions are publicly available, it's possible the models may have been trained on such data, including competition details and solutions. Additionally, the AGI benchmark is also resource-intensive. A single full run of the experiment by OpenAI scientists required 1800 GPU hours of compute, making it impractical for several applications.
The researchers further explained that a model capable of successfully tackling a substantial portion of MLE-bench is likely equipped to handle numerous open-ended ML tasks. To support research into the agentic capabilities of language models and improve transparency around acceleration risks in research labs, they have chosen to open-source MLE-bench.
This move to open source will allow other researchers to test other AI models against MLE-bench. The OpenAI researchers hope their work on MLE-bench will help advance the understanding of how AI agents can autonomously perform ML engineering tasks, which is crucial for the safe deployment of more powerful models in the future.