Role Reversal: Google Teases Nvidia’s Blackwell as It Softens TPU Rivalry
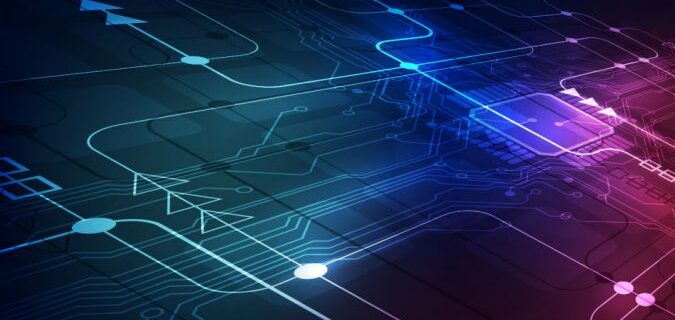
Customers now have access to Google’s homegrown hardware — its Axion CPU and latest Trillium TPU — in its Cloud service. At the same time, Google gave customers a teaser on Nvidia’s Blackwell coming to Google Cloud, which should arrive early next year.
“We’re… eagerly anticipating the advancements enabled by Nvidia’s Blackwell GB200 NVL72 GPUs, and we look forward to sharing more updates on this exciting development soon,” said Mark Lohmeyer, vice president and general manager for compute and AI infrastructure at Google Cloud, in a blog entry.
Google is preparing racks for the Blackwell platform in its cloud infrastructure.
Google was doing head-to-head comparisons of its TPUs to Nvidia’s GPUs in the past, but that tone has softened.
The company is taking steps to further integrate Nvidia’s AI hardware into Google Cloud HPC and AI consumption models via specialized hardware, such as a new network adapter that interfaces with Nvidia’s hardware.
Google wants to bring hardware and software coherency into its cloud service for customers at a system level, regardless of the technology.
It’s another role reversal in the chip industry’s “best friends forever” era, with rivals burying hatchets. AMD and Intel recently joined hands to keep x86 buzzing in the AI era, and Google is trying to move customers to its hardware for inferencing while giving Nvidia’s hardware equal footing.
Google recognizes that diversity in its cloud service is good for business and that there is an insatiable demand for GPUs.
The demand for AI hardware is overwhelming. Nvidia’s GPUs are also in short supply, and customers are already moving to Google’s TPUs.
Google’s Trillium TPU
Google’s new TPU, called Trillium, is now available for preview. It replaces the TPU v5 products and provides significant performance improvements.
The company has rebranded its TPUs to Trillium, which is basically a TPUv6. Trillium was announced just a year after TPUv5, which is surprisingly fast, considering it is about three-to-four years from TPUv4 to TPUv5.
The Trillium chip provides 4.7 times more peak compute performance over TPU v5e when measuring BF16 data type. The TPUv5e peak BF16 performance was 197 teraflops, which should put Trillium’s BF16 peak performance at 925.9 teraflops. However, as with all chips, the real-world performance never reaches theoretical estimates.
The performance boost was expected after the TPU v5e’s 197 teraflops BF16 performance actually declined from 275 teraflops on the TPUv4.
Google shared some real-world AI benchmarks. The text-to-image Stable Diffusion XL inferencing was 3.1 times faster on Trillium than on TPU v5e, while training on the Gemma2 model with 27 billion parameters was four times faster. Training on the 175-billion parameter GPT3 was about three times faster.
Trillium boasts a host of chip improvements. It has two times more HBM memory than TPU v5e, which had 16GB of HBM2 capacity. Google didn’t clarify if Trillium had HBM3 or HBM3e, which is in Nvidia’s H200 and Blackwell GPUs. HBM3e memory has more bandwidth than HBM2 memory.
Google has also doubled Trillium’s inter-chip-interface communication compared to TPU v5e, which had an ICI of 1,600Gbps.
Supercomputers packed with TPUs can be assembled by interconnecting tens of thousands of pods, each with 256 Trillium chips. Google has developed a technology called Multislice that distributes large AI workloads across thousands of TPUs over a multi-petabit-per-second datacenter network while ensuring high uptime and power efficiency.
Trillium also gets a performance boost with third-generation SparseCores, an intermediary chip closer to high-bandwidth memory, where most of the AI processing occurs.
Axion CPUs
Google’s first CPU, Axion, was intended to be paired with Trillium. Google is making these chips individually available in VMs for inferencing.
The ARM-based Axion CPUs are available in its C4A VM offerings and offer “65% better price-performance and up to 60% better energy efficiency than comparable current-generation x86-based instances” for workloads such as web-serving, analytics, and databases, Google said.
But take those benchmarks with a pinch of salt. At some point, a beefier x86 chip would be required to handle databases and ERP applications. Fresh independent Google Cloud Axion vs x86 instance benchmarks are available from Phoronix.
Connecting Nvidia and Google’s Cloud
Nvidia’s H200 GPU is finally available in Google Cloud in the A3 Ultra virtual machines. Google is directly bridging its hardware infrastructure into Nvidia’s hardware interfaces via high-speed networking.
At the core is Titanium, which is a hardware interface that allows Google Cloud to run smoothly and efficiently with workload, traffic, and security management.
Google has introduced a new Titanium ML network adapter that includes and “builds on the Nvidia ConnectX-7 hardware to further support VPCs, traffic encryption, and virtualization.”
“While AI infrastructure can benefit from all of Titanium’s core capabilities, AI workloads are unique in their accelerator-to-accelerator performance requirements,” Lohmeyer said.
The adapter creates a virtualization layer that runs a virtual private cloud environment but can take advantage of various AI hardware, including Nvidia’s environment.
It’s unclear if the Titanium ML interface would allow customers to connect or switch between Google’s Trillium and Nvidia GPUs when running unified AI workloads. Lohmeyer previously told HPCwire that it was making that concept possible in containers.
Google did not immediately respond to requests for comment on this, but HPCwire will update after talking to Google Cloud about it.
Nvidia’s hardware already provides a blueprint for GPU-optimized offload systems. Google already has a system that optimizes GPU workload management in its cloud service.
The Hypercomputer interface includes a “Calendar” consumption model that defines when a task should start and end. A “Flex Start” model has guarantees on when a task will end and deliver results.
HPC workloads
The company announced Google Hypercluster, which offers customers one-click deployments of pre-defined workloads via an API call. The Hypercompute cluster automates the network, storage, and compute management, which can otherwise be complex to manage.
The deployments include popular AI models and HPC workloads. Google has followed AWS’s footsteps with SLURM (Simple Linux Utility for Resource Management) scheduler, which allows customers to orchestrate their own storage, networking, and other components in an HPC cluster.
Google didn’t share additional details about how SLURM will integrate into the Hypercluster.
This article first appeared on sister site HPCwire.