Microsoft-Backed Fastino Launches with $7M to Power GPU-Less AI Models
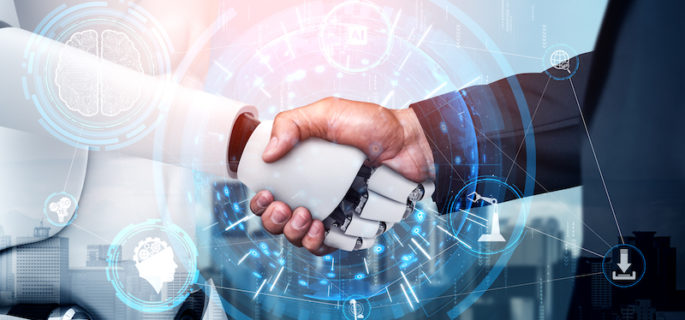
Fastino, a new foundation model provider, emerged from stealth today with a $7 million pre-seed funding round. The company says it offers a family of task-optimized language models that are more accurate, faster, and safer than traditional LLMs.
Fastino claims to have a differentiated approach to generative AI. Fastino CEO and co-founder Ash Lewis explained his company’s concept in a recent interview with AIwire.
“We started building a new model architecture, and it turns out that it's very performant on specific tasks,” said Lewis. “If we can cut out everything that a model is trying to do apart from the task that we're trying to make it do, you may lose breadth, but you're able to do that one task better than a large language model could do, and you're able to do it on a CPU.”
Lewis claims the biggest difference between his models and traditional LLMs is that the larger models can do any task to a reasonable standard, whereas his task-optimized models can only do one task but to an exceptionally high standard. Fastino’s foundation models are called “task-optimized” due to the company’s goal of making its models more narrowly focused for specific enterprise tasks, claiming they enable much higher accuracy than the larger, more universal LLMs.
Fastino says its key features include task-optimized models for critical enterprise use cases like the structuring of textual data, RAG systems, text summarization, and task planning. The company also touts the ability for CPU-level inferencing, claiming its novel architecture operates up to 1000x faster than traditional LLMs and is designed to enable flexible deployment on CPUs or NPUs to minimize reliance on expensive GPUs.
The company also claims its task-optimized models are safer and can enable new, distributed AI systems that are less vulnerable to adversarial attacks, hallucinations, and privacy risks.
Lewis says Fastino's model architecture is not a traditional transformer architecture, but a blend.
“We borrowed from the traditional transformer, but we've created a new architecture on top of it. I started working on this myself, but now we’ve got a team that is way smarter than me from Stanford, Google, Microsoft, and Apple,” he told AIwire. Though details are a bit scant at the moment, Lewis explains his team has made breakthroughs in AI model architecture research that they plan to publish in the coming year.
Lewis previously launched DevGPT, a community for developers using AI for autonomous coding that boasted clients like Spotify, Doordash, and IBM. This experience led him to this moment: “Last year, I was running DevGPT and we were spending about a million dollars a year on OpenAI, which wasn't incredibly economically viable. And that became a frustration point, which led to us taking this problem quite seriously.”
The cost of training traditional LLMs with thousands of GPUs can be staggering, like Sam Altman's estimate that training GPT-4 cost over $100 million. “After moving on from DevGPT, I think we approached the problem not from an academic standpoint, but from an engineering standpoint of what can be redone in these existing sequential models in order to make them more efficient,” Lewis said.
Lewis then built the very first model, one that could only tell children’s stories and nothing else. He showed it to co-founder George Hurn-Maloney, a Silicon Valley investor who is now Fastino's co-founder and COO. Hurn-Maloney then previewed it to Microsoft, gaining their interest as well as other investors, prompting Lewis to move to Palo Alto from England and the rest is history, he says.
His experience with DevGPT taught Lewis that current generative AI business models are unsustainable for many companies. Lewis is focused on building a new generation of large language model companies, saying it is not just the technology that needs an overhaul. “There's also a lot of business model innovation that needs to happen,” he said, noting that he wants to give enterprises more control over the costs of training and deploying LLMs with possibilities such as a flat annual fee.
Today’s $7 million pre-seed funding round was led by Insight Partners and M12, Microsoft’s Venture Fund, with participation from NEA, Valor, Github CEO Thomas Dohmke, and others. The company is currently hiring for several full-time positions including research scientist, machine learning engineer, and software engineer.
It will be interesting to see if Lewis and other hopefuls can break through the growing wall of AI expenses that the biggest players like OpenAI and Nvidia have built.
Lewis is optimistic, saying his models can beat GPT-4o on certain industry benchmarks for the tasks they’ve been trained on, and the models can be trained for much less money: “There's a reason why we didn't have to raise hundreds of millions of dollars, like a lot of foundational model companies out there. So far, we haven't spent $1 on GPU. Training these models is a lot more efficient.”