LANL Explores Diffusion-Based AI Models for Accelerator Diagnostics
Jan. 17, 2025 -- Accelerators — machines that speed up particles such as protons — are useful in nuclear and high-energy physics as well as materials science, dynamic imaging and even isotope production for cancer therapy. A Los Alamos National Laboratory-led project presents a machine learning algorithm that harnesses artificial intelligence capabilities to help tune accelerators, making continuous adjustments that keep the beam precise and useful for scientific discovery.
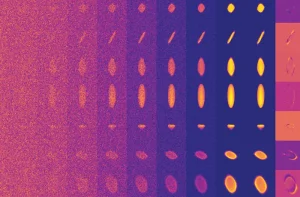
LANL: A 2D projection of a particle accelerator beam, computer-generated based on generative diffusion. The process is adaptively guided by accelerator signals that take the image from pure noise (left) to increasingly clear versions (right). (Source: LANL)
“The complexity and time variation of the machinery means that over extended usage, the characteristics of an accelerator’s particle beam change,” said Alexander Scheinker, research and development engineer at Los Alamos and the project’s lead. “Factors like vibrations and temperature changes can cause problems for accelerators, which have thousands of components, and even the best accelerator technicians can struggle to identify and address issues or return them to optimum parameters quickly. It is a high-dimensional optimization problem that must be repeated again and again as the systems drift with time. Turning these machines on after an outage or retuning between different experiments can take weeks.”
An accelerator that can be effectively tuned in real time can provide higher currents to experiments and is more likely to stay running, offering more beam time for science experiments, and is also more likely to ensure precise results. In a collaboration with Lawrence Berkeley National Laboratory, the approach developed by Scheinker couples adaptive feedback control algorithms, deep convolutional neural networks and physics-based models in one large feedback loop to make better, noninvasive predictions that enable autonomous control of compact accelerators.
Teaching Machine Learning Applications to Tune Accelerators
In addition to complex controls and diagnostics, scientists in the accelerator community use feedback algorithms to help accelerators adapt to component shifts over time. But because they are based on local accelerator feedback, such algorithms can get stuck with a local solution that is not the overall best solution. Machine learning algorithms, however, can use training data to identify relationships between data and results with a higher-level, global view.
What Scheinker and colleagues at Lawrence Berkeley have developed is a new machine learning technique that tweaks the model with real-time data from accelerator diagnostics. The team has developed an adaptive version of the advanced generative AI process known as diffusion, which includes the capability to virtually diagnose the accelerator beam. The non-invasive approach, described in Scientific Reports, means that diagnostics can occur in real time during beam operations.
Demonstrating the diffusion-based model at the European X-Ray Free-Electron Laser Facility, an X-ray pulse accelerator in Germany, the team was able to capture images of the particle beam in respect to time versus energy. Across a wide range of accelerator settings, the model’s data resulted in accurate predictions of the distributions of a diverse set of particle group profiles.
The tool can also reconstruct the multi-dimensional projections into a representation that is useful for real-time training of the AI algorithm. The conditional diffusion variational autoencoder (cDVAE) is a diffusion approach tested on the High Repetition-rate Electron Scattering (HiRES) beamline at the Berkeley lab. The cDVAE was able to extrapolate beyond the training data and between various beam setups, suggesting its potential as a general method that can be applied for accelerator diagnostics.
“The results we’ve seen from our diffusion model studies show a great deal of promise,” Scheinker said. “Tuning accelerators with these kinds of advanced machine learning techniques will bolster our ability to drive scientific discovery.”
The work represents ongoing progress from Los Alamos’ Adaptive Machine Learning team, led by Scheinker, which over several years has been developing novel adaptive AI methods for real-time autonomous control and optimization of complex dynamic systems, including particle accelerators and dynamic imaging experiments of interest to the Laboratory.
Scheinker’s team at Los Alamos is also developing such adaptive diffusion models for the Laboratory’s LANSCE accelerator.
Funding: This work is funded by the U.S. DOE Office of Science, Office of High Energy Physics Accelerator Stewardship program and the Laboratory Directed Research and Development program at Los Alamos.
Papers: “cDVAE: VAE-guided diffusion for particle accelerator beam 6D phase space projection diagnostics.” Scientific Reports. DOI: 10.1038/s41598-024-80751-1
“Conditional guided generative diffusion for particle accelerator beam diagnostics.” Scientific Reports. DOI: 10.1038/s41598-024-70302-z
Source: LANL