Five Big Questions for HPC-AI in 2025
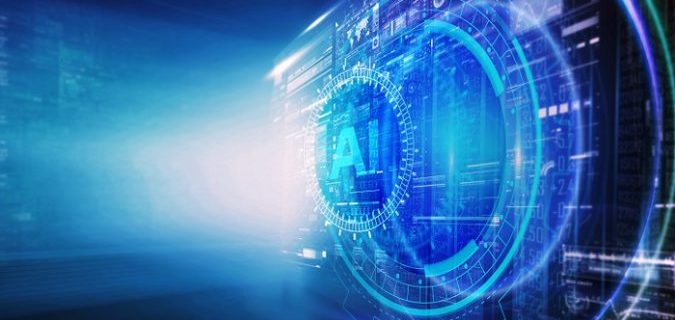
2024 ended in a bang for the HPC-AI market. SC24 had record attendance, the Top500 list had an impressive new number one with El Capitan at Lawrence Livermore National Laboratory, and the market for AI boomed, with hyperscale companies spending more than double their already lofty investments in 2023.
So why does it all feel so unstable? As we kick off 2025, the HPC-AI industry is at a tipping point. The ballooning AI market is dominating the conversation, with some people concerned it will take the air out of HPC, and others (or perhaps the same people) waiting for the AI bubble to pop. Meanwhile political change is threatening the status quo, potentially altering the market dynamics of HPC-AI.
Intersect360 Research is forming its research calendar for the year, fueled by inputs from the HPC-AI Leadership Organization (HALO). As we lay out the surveys that will help us craft a new five-year forecast, here are five big questions facing the HPC-AI market for the back half of the decade.
1. How big can the AI market get?
In our pre-SC24 webinar, Intersect360 Research made a dramatic adjustment to its 2024 HPC-AI market forecast, announcing that we expected a second straight year of triple-digit growth in hyperscale AI, with more years of high growth rates ahead. We also increased the outlook for the blended, on-premises (non-hyperscale) HPC-AI market, but this relatively modest bump was dwarfed by the mammoth gains posted by hyperscale.
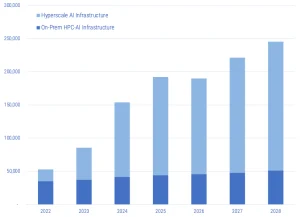
Revised HPC-AI Market Forecast ($M) Intersect360 Research, November 2024. (Source: Intersect360 Research)
AI has already dominated the conversation in data center infrastructure. At Hot Chips 2024, for example, the few presentations that didn’t focus explicitly on AI still made reference to it. Vendors are racing to embrace the seemingly boundless growth in the AI market.
The hyperscale AI market is predominantly consumer in nature, and there is precedent for it. Hyperscale hit its initial growth spurt by creating cloud data center markets out of consumer markets that did not rely on enterprise computing previously. Calendars, maps, video games, music, and videos used to exist offline, and social media is a category with no prior analogy. AI is building on all these phenomena, and it is creating new ones.
No market is truly boundless, but the hyperscale component is still groping for its ceiling. To take one example, Meta announced in its April 2024 earnings call that it was increasing its capital expenditures to $35 to $40 billion per year to accommodate its accelerated investment in AI infrastructure. Taking out a measure of capex potentially not related to AI, that still leaves about $10 per user across Meta’s approximately 3.2 billion users worldwide on its various platforms (Facebook, Instagram, WhatsApp, etc.).
In that context, it makes sense that a hyperscale company might expect it can earn an additional ten dollars of profit per user per year through the use of AI. To go higher, a company would need either more users, or more anticipated value per user. Not many companies count more than a third of the world population as users. Can a single user’s personal data be worth twenty dollars more because of AI? One hundred
Beyond economics, the most cited limiting factor in hyperscale AI data center production is power consumption. AI data centers are being constructed hundreds of megawatts, even gigawatts, at a time. Companies are pursuing innovative solutions to find power to fuel these buildouts. Most notoriously, Microsoft signed a contract with the Crane Clean Energy Center that will restart Unit 1 at Three Mile Island, the Pennsylvania site that suffered a nuclear meltdown accident in 1979. (Unit 1 is independent from Unit 2, which suffered the accident, and Unit 1 continued operations thereafter.)
Hyperscale AI is therefore entwined with the notion of sustainability, and whether it is globally responsible to consume so much power. But if spending tens of billions of dollars per year hasn’t been an impediment, then finding the power hasn’t been either, and hyperscale companies have not yet found the limit to how much power they can access and consume.
Perhaps the most amazing fact about all this growth in hyperscale AI is that it hasn’t been the focus of most data center conversations. Instead, we are chasing the notion of “enterprise AI,” the promise of AI to revolutionize enterprise computing.
This revolution will undoubtedly happen. Just as personal computers, the internet, and the world wide web all revolutionized the enterprise, so will AI. The market opportunity for enterprise AI is bounded by the expected business result. For AI to be a profitable initiative, there are two paths: it can reduce costs, or it can bring in more revenue.
To date, most of the emphasis seems to be on cost optimization, such as through streamlined operations or (let’s face it) reduction of headcount. This investment is limited by a simple riddle: How much money will you spend to save one dollar? Even annualizing the payoff—dollars per year—gives a practical limit to how much it is worth spending. Furthermore, there are diminishing returns to this path. If a company can spend two million dollars to save one million dollars per year, it is unlikely it can repeat the trick, at the same level to the same benefit, with the next two million.
As to increased revenue, there are two types: primary (creating more revenue overall) and secondary (taking share from a competitor). Let’s look at an airline as an example. By implementing AI, will the airline get more people to take flights? Will passengers, on average, spend more money per flight, specifically because of the airline’s AI? (Bonus question: If so, how does this affect consumer spending in other markets? Or do people just have more money?)
More likely, we’re looking at a competitive market share argument: More customers will choose Airline A over Airline B because of Airline A’s AI investment. Making a timely pivot in this case can be important. Amazon got its start as a bookseller. If Borders or Barnes and Noble had made an earlier investment in web commerce, Amazon might never have had its chance.
But this is a zero-sum game. If both Airline A and Airline B make the same investment in AI, and their respective revenues are unchanged, they spend necessary money for no gain. (This is a classic “prisoner’s dilemma” in microeconomics game theory. In this simplified example, both airlines are better off if neither invests, but each is individually better off making the investment, regardless of what the other does.)
Ultimately, those who build hardware, models, and services for AI are banking on a major enterprise migration. If AI goes the way of the web, then in ten years, a robust AI investment will simply be considered the cost of doing business, even if profitability hasn’t soared as a result. In this way, AI becomes a dominant part of the IT budget, but probably not much different quantitatively than IT budgets as they already existed.
2. Will hyperscale completely take over enterprise computing?
In the pursuit of enterprise AI, some hardware companies may be ambivalent as to whether the systems for AI wind up on-premises or in the cloud, but for the hyperscale community, everything (including AI) as-a-Service is the vision of the future. We’ve already seen the cloudification of consumer markets. With the extreme concentration of data at hyperscale, AI could be the lever that does the same for enterprise.
Intersect360 Research has been forecasting an asymptote in cloud penetration of the HPC-AI market, at roughly one-quarter of total HPC budget. The primary limiting factor isn’t any cloud barrier, but rather simple cost; for anyone who can hit a high enough utilization mark, it’s cheaper to rent than to buy. Furthermore, data gravity and sovereignty issues are pushing more organizations to lean on-premises. As one example, representatives from GEICO presented its movement away from cloud for its full range of applications, including HPC and AI, at the OCP Global Summit in September.
But what if cloud becomes the only choice? Over three-quarters of HPC-AI infrastructure—and of all data center infrastructure overall—is now consumed by the hyperscale market. The top hyperscale companies spend tens of billions of dollars per year; each of them is a market unto itself. Component and system manufacturers understandably prioritize them when it comes to product design and availability.
Those seeking solutions for HPC-AI may find that the latest technologies simply aren’t available, as hyperscalers are capable of consuming the entire supply of a particular product. Nvidia GPUs—the definitive magical gems that enable AI—when they are available at all, are priced high, with long wait times. HPC-focused storage companies are similarly engaged in hyperscale AI deployments.
AI is capable of tipping the scales further in favor of cloud. If it does, it would push the on-premises market for HPC-AI technologies into decline. Traditional OEM enterprise product and solutions companies, such as HPE, Dell, Atos/Eviden, Fujitsu, Cisco, EMC, and NetApp, would compete for a smaller market. (Others, including Lenovo, Supermicro, and Penguin Solutions, have already embraced hybrid ODM-OEM business models in order to sell effectively to the high-growth hyperscale market.)
Fueled by AI, hyperscale companies have already grown well beyond levels forecast by Intersect360 Research. Historically this level of market concentration has been unstable. Five years ago, in forecasting the hyperscale market, Intersect360 Research wrote, “Such market power is not unprecedented in the world’s economic history, but it has not previously been seen at this level in the information technology era.”
Hyperscale has grown much more since then. The worldwide data center market is concentrated into a small number of buyers. If the trend continues, it will fundamentally disrupt how enterprise computing is bought and used, and the view of everything-as-a-service could become a reality, whether buyers want it or not.
3. What effect will the new U.S. administration have on HPC-AI?
National sovereignty concerns around HPC-AI capabilities have been on the rise for years. The global HALO Advisory Committee recently cited “HPC nationalism” as a key issue impeding industry progress. There are independent initiatives for HPC-AI sovereignty, based on local technologies, in the U.S., China, the EU, the UK, China, Japan, and India. The New York Times reported [subscription required] that King Jigme Khesar Namgyel Wangchuck of Bhutan recently traveled to visit Nvidia’s headquarters in California to discuss the building of AI data centers.
President Trump is already accelerating this trend toward national independence. His political platform is one of American exceptionalism, and his first days in office signaled an intention to promote American greatness. Notably, Trump amplified the announcement of the Stargate Project, “a new company which intends to invest $500 billion over the next four years building new AI infrastructure for OpenAI in the United States.” Trump called Stargate “a new American company … that will create over 100,000 American jobs almost immediately.”
The Stargate Project can hardly be called a Trump achievement, since it was evidently already in the works before Trump took office. Furthermore, the investment doesn’t come from the U.S. government. Two of the primary funders, Softbank (Japan) and MGX (UAE) are non-American companies; MGX was established only recently by the Emirati government. But Trump might get credit for creating an environment that kept the data centers and the associated jobs in the U.S.
Trump seized on the announcement and tied it to his intended policies. “It’ll ensure the future of technology. What we want to do is we want to keep it in this country. China is a competitor, and others are competitors. We want it to be in this country, and we’re making it available,” Trump noted.
As to construction and power generation for Stargate, Trump vowed to make things easy. “I’m going to help a lot through emergency declarations, because we have an emergency. We have to get this stuff built,” he said. “They have to produce a lot of electricity, and we’ll make it possible for them to get that production done very easily, at their own plants, if they want.”
Coupled with other actions, such as Trump’s immediate withdrawal from the Paris Climate Accords, Trump sends a message that he wants American investment to race ahead, with urgency, regardless external factors, such as other countries’ sentiments or concern for the environment. He promises to clear hurdles for businesses, primarily through deregulation, and he will promote American energy production. All these actions should translate to a net increase in spending on HPC-AI technologies, not only by hyperscale companies, but also in key HPC commercial vertical markets, such as oil and gas exploration, manufacturing, and financial services.
Public sector spending is more in doubt. The new Department of Government Efficiency (DOGE), an unofficial advisory operating outside the establishment, headed by Elon Musk, is specifically tasked with slashing government spending. Some supercomputing strongholds, such as the Office of Science and Technology Policy (OSTP) within the U.S. Department of Energy, have traditionally held solid bipartisan support. Other government departments, such as NASA, NSF, or NIH, could come under the microscope, or worse, the axe.
Consider the National Oceanic and Atmospheric Administration (NOAA), which operates under the U.S. Department of Commerce. Google announced last month that its GenCast ensemble AI model can provide “better forecasts of both day-to-day weather and extreme events than the top operational system, the European Centre for Medium-Range Weather Forecasts’ (ECMWF) ENS, up to 15 days in advance.” Within the next four years, would DOGE recommend downsizing (or eliminating) NOAA in favor of a private-sector AI contract?
What happens in the U.S. will naturally have effects abroad. The European Commission had already been concerned with establishing HPC-AI strategies that do not rely on American or Chinese technologies. Anders Jensen, the executive director of the EuroHPC Joint Undertaking, said in an interview with Intersect360 Research senior analyst Steve Conway, “Sovereignty remains a key guiding principle in our procurements, as our newly acquired systems will increasingly rely on European technologies.” With the threat of U.S. tariffs and export restrictions rising, these efforts can only escalate.
China had already been working toward HPC-AI technology independence, and Chinese organizations have stopped submitting system benchmarks to the semiannual Top500 list. The coming years will likely see a U.S.-China “AI race” akin to the U.S.-USSR space race of the previous century. Other countries with smaller but still notable HPC-AI presences, such as Australia, Canada, Japan, Saudi Arabia, South Korea, or the UK, will be challenged to devise strategies to keep pace.
Returning to the U.S., it is worth considering what “American leadership” means in this context. While the EU focuses on public sector funding and China has a unique model of state-controlled capitalism, American companies are neither owned nor controlled by the U.S. government. The world’s largest hyperscale organizations are headquartered in the U.S., but they are global companies that rely on foreign customers. Similarly, key technology providers, such as Nvidia, Intel, and AMD, are American companies that also sell their products abroad. Limiting the distribution of those products hurts the companies in question.
In a blog, Ned Finkle, VP of Government Affairs at Nvidia, blasted the Biden administration’s “AI Diffusion” rule, passed in President Biden’s final days in office, as “unprecedented and misguided,” calling it a “regulatory morass” that “threatens to squander America’s hard-won technological advantage.” Considering these views, the Trump administration has a tricky needle to thread—promoting the use of world-leading American technologies for HPC-AI, such as Nvidia GPUs, while keeping leadership over other countries, notably China, which the U.S. government considers to be competition.
4. Can anyone challenge Nvidia?
The opinion of Nvidia’s executive leadership matters, because when it comes to AI, Nvidia controls the critical technology, the GPU. GPUs were once confined to graphics, until Nvidia executed a masterful, decade-long campaign to establish the CUDA programming model that would bring GPUs to HPC. When it turned out that the GPU was a perfect fit for the neural network computing that would power machine learning, Nvidia was truly off to the races.
Nvidia now thoroughly dominates the AI market for GPUs and their associated software. Additionally, Nvidia has pioneered a proprietary interconnect, NVlink, for networking GPUs and their memory into larger, high-speed systems. Through its 2020 acquisition of Mellanox, Nvidia assumed control of InfiniBand, the leading high-speed system-level interconnect for HPC-AI. And Nvidia has vertically integrated into complete system architecture, with its Grace Hopper and Grace Blackwell “superchip” nodes and DGX SuperPOD infrastructure.
Most importantly, Nvidia is seeking to eliminate its reliance on outside technology through the release of its own CPU, Grace. Nvidia Grace is an ARM architecture CPU that complements Nvidia GPUs in Grace Hopper and Grace Blackwell deployments. While Nvidia has a commanding lead in GPUs, it is coming from behind in CPUs, which remain at the heart of the server.
The two most natural competitors to Nvidia, therefore, are the leading U.S.-based CPU vendors, Intel and AMD. Intel’s big advantage is in the CPU. Intel’s Xeon CPUs are still the leading choice for enterprise servers, and decades’ worth of legacy software is optimized for it. In mixed-workload environments serving both traditional scientific and engineering HPC codes and emerging AI workloads, the compatibility and performance of those CPUs is important.
This entrenched advantage offered Intel an inside lane for defending against the incursion of the GPU. To Intel’s credit, the company foresaw the threat and attempted to head it off. In the early days of CUDA, Intel announced its own computational GPU, codenamed Larrabee. The project was canceled less than two years after it was conceived, having never come to market.
Since that time, Intel has tried and failed at one accelerator project after another, including the Many Integrated Core (MIC) architecture, which became Intel Xeon Phi, a commercial flop as both an accelerator and as an integrated CPU. Intel’s latest GPU accelerator, codenamed Ponte Vecchio, suffered a series of delays and fell short of performance expectations in the Aurora supercomputer at Argonne National Laboratory.
Intel has now scrapped both Ponte Vecchio and a previously planned follow-on codenamed Rialto Bridge, so those looking for an Intel GPU are left waiting for a product called Falcon Shores and its successor, Jaguar Shores, though the future of all things Intel is cloudy in the wake of CEO Pat Gelsinger’s sudden retirement. Intel does currently offer a non-GPU AI accelerator, Intel Gaudi, that has not made significant inroads against Nvidia’s dominance.
Intel has made retreats from other tentative forays outside of its core CPU business. Intel developed Omni-Path Architecture to compete with InfiniBand as a high-end system interconnect for HPC. After only modest success, Intel backed away; Omni-Path was picked up off Intel’s trash pile by Cornelis Networks, which now carries it forward. Intel, AMD, Cornelis Networks, and others are now part of the Ultra Ethernet Consortium, which seeks to enable high-performance Ethernet-based solutions capable of competing with Nvidia InfiniBand.
Conversely, AMD has had significant successes with both its AMD EPYC CPUs and AMD Instinct GPUs. Among the three primary vendors, AMD was the first to market with both CPU and GPU connected in an integrated system. AMD continues to gain share in HPC-AI, drafting off of its two most notable wins, the El Capitan supercomputer at Lawrence Livermore National Laboratory and the Frontier Supercomputer at Oak Ridge National Laboratory, both led by HPE.
AMD’s weakness is the software ecosystem. In a 2023 Intersect360 Research survey, HPC-AI users rated AMD ahead of both Nvidia and Intel for the price/performance of GPUs. But Nvidia crushed Intel and especially AMD in perceptions of software ecosystem. (See chart.) Nvidia also led all user ratings in “technology” and “future prospects.”
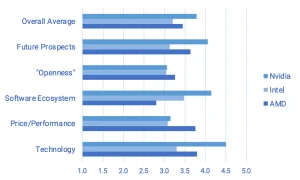
HPC-AI User Ratings of Leading GPU Vendors
User perceptions on five-point scale: 1 = “terrible”; 5 = “excellent”.
(Source: Intersect360 Research HPC-AI Technology Survey, 2023)
Naturally, buyers aren’t limited to only Nvidia, Intel, and AMD as choices. Companies such as Cerebras, Groq, and SambaNova have all had notable wins with their accelerators for AI systems. But none of these is large enough to be a competitive market threat to Nvidia’s dominance. What could be a factor is if one of these companies or their cohorts were acquired by a hyperscale company.
Nvidia is so far ahead in the AI game, the bigger threat to Nvidia (and possibly the only real threat) is a complete paradigm shift. Hyperscale companies have been Nvidia’s largest customers. These companies are fully aware of their reliance on Nvidia GPUs, which are in competitive demand globally, and therefore expensive and often in short supply. Amazon, Google, and Microsoft are all designing their own CPUs or GPUs internally, either offering them in their cloud services to others or for their own exclusive use.
At the same time, Nvidia has invested in enabling a new breed of GPU-focused clouds. CoreWeave, Denvr DataWorks, Lambda Labs, and Nebius are only a small few examples of cloud services that offer GPUs. Some of these are newcomers; others are converted bitcoin miners that now see greener pastures in AI.
This puts Nvidia in competition with its own customers on two fronts. First, Nvidia is designing complete HPC-AI systems, in competition with server OEM companies like HPE, Dell, Lenovo, Supermicro, and Atos/Eviden, which carry Nvidia GPUs to market in their own configurations. Second, Nvidia is funding or otherwise enabling GPU clouds, in competition with its own hyperscale cloud customers, who themselves are designing processing elements, potentially reducing their future reliance on Nvidia.
If AI continues to grow, and hyperscale continues to dominate, and constraints are removed from the U.S. marketplace, then we are potentially left with a new competitive paradigm. By the end of the decade, the question may not be whether Intel or AMD can catch up to Nvidia, but rather, how does Nvidia compete with Google, Microsoft, and Amazon.
Through this lens, the competitive space is wide open. For Project Stargate, which purports to have $500 billion to spend in the next four years, OpenAI allied with Oracle and Microsoft, with Nvidia as a primary technology partner. Last year, X.ai, led by the aforementioned DOGE czar Elon Musk, moved into the top tier of hyperscale AI spending with the implementation of the Colossus AI supercomputer. Musk could make things even more interesting if he were to add to his stable of technologies by acquiring a company with a specialty AI inference processor.
5. What about good old HPC?
As the competitive dynamics continue to shift, the old-guard HPC crowd has understandably looked to the host of ways AI can be integrated with HPC, including notions such as AI-augmented HPC. Beyond straightforward tasks like code migration, AI can be deployed for HPC pre-processing (e.g., target reduction), post-processing (e.g., image recognition), optimization (e.g., dynamic mesh refinement), or even integration (e.g., computational steering). As AI thrives, we embrace a rosy view of a merged HPC-AI market.
This is a dream from which HPC needs a wake-up call. While AI does present these benefits to HPC, and more, it has also created a crisis.
At SC24, we rightfully celebrated El Capitan, our third exascale supercomputer, the most powerful in the world. And yet, we all knew we were kidding ourselves. Glenn Lockwood, formerly a high-performance storage expert at NERSC, now an AI architect for Microsoft Azure, confirmed in his traditional post-SC blog that Microsoft is “building out AI infrastructure at a pace of 5x Eagles (70,000 GPUs!) per month,” referring to the Microsoft Eagle supercomputer, currently number four on the Top500 list, behind the three DOE exascale systems. Microsoft, or another hyperscale company, could clearly post a larger score if desired.
We are accustomed to thinking of these national lab supercomputers as world leaders that set the course of development for the broader HPC and enterprise computing markets. This is no longer true. A $500 million, 30-megawatt supercomputer isn’t world-leading any more. It’s not even an especially big order. A DOE supercomputer may still be critical for science, but going forward, the course of the enterprise data center industry will be set by AI, not traditional supercomputing.
If that doesn’t sound important, it is. For as much as the HPC crowd has discussed the convergence of HPC and AI, we’re now heading in the opposite direction, as the technologies and configurations that serve AI drift farther away from what scientific computing needs.
This is most evident in the discussion of precision. While HPC has relied on 64-bit, double-precision floating point calculations, we have seen AI—particularly for inferencing—gravitate down through 32-bit single-precision, mixed-precision, and 16-bit half-precision, now into various combinations of “bfloats” and either floating point or integer at 8-bit, 6-bit, or even 4-bit precision. With some regularity, companies now promote how many “AI flops” their processors or systems are capable of, with no definition in sight of what an “AI flop” represents. (This is as silly as having a competition to see who can eat the most cookies, with no boundaries or standards as to how small an individual cookie might be.)
Some of this discussion of precision can be of benefit to HPC. There are cases in which very expensive, high-precision calculations can be thrown at models that aren’t very precise to begin with. But in a 2024 Intersect360 Research survey of HPC-AI software, users clearly identified FP64 as being the most important for their applications in the future. (See chart.)
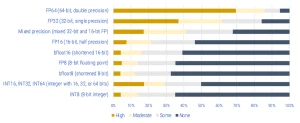
Future Importance of Precision Levels for HPC-AI Applications
Weighted averages across 11 application domains
(Source: Intersect360 Research HPC-AI Software Survey, 2024)
If processor vendors are driven by AI, we could see FP64 slowly (or quickly) disappear from product roadmaps, or at the very least, get less attention than the AI-driven lower-precision formats. Application domains with more reliance on high-precision calculations, such as chemistry, physics, and weather simulation, will have the biggest hurdles to overcome.
The balance of CPUs and GPUs is also different between traditional HPC and newer AI applications. Despite all Nvidia’s work with CUDA and software, most HPC applications do not run well on more than two GPUs per node, and many applications are still best in CPU-only environments. Conversely, AI is often best run with high densities of GPUs, with eight or more per node. Furthermore, these AI nodes might be well-served to have CPUs with relatively low power consumption and high memory bandwidth—strengths of ARM architecture, embodied in Nvidia Grace CPUs.
The blended HPC-AI market is now full of server nodes with four GPUs per node, the most common configuration currently installed. This might work well in some scenarios, but in others, it can be the compromise that both sides hate equally: too many GPUs to be used effectively by HPC applications; not enough for AI workloads. For its newest supercomputer, MareNostrum 5, Barcelona Supercomputing Center (BSC) chose to specialize its nodes in various partitions, some with more GPUs per node, some with less. Composability technologies might also help in the future, allowing one node to use the GPUs from another. GigaIO and Liqid are two HPC-oriented companies pursuing system-level composability, but adoption thus far is limited.
High-performance storage is getting hijacked as well. Companies we associate with HPC data management, such as DDN, VAST Data, VDURA (formerly Panasas), and Weka, are now growing at astounding rates, thanks to the applicability of their solutions to AI. Fortunately for HPC, at this point, it has not led to a major change in how high-performance storage is architected.
Ultimately, if the solutions that drive enterprise computing are changing, then HPC might have to change with it. If this sounds extreme, take comfort. It has happened before.
HPC has been at the wag end of a larger enterprise computing market for decades. Market forces drove the migration from vector processors to scalar, from Unix to Linux, and from RISC to x86. These last two came at the same time, thanks to the biggest transition, from symmetric multi-processing (SMP) to clusters.
Clusters arrived in force in the late 1990s via the Beowulf project, which promoted the idea that large, high-performance systems could be constructed from industry-standard, x86-Linux servers. These commodity systems were being lifted into prominence by a trend that had about as much hype and promise then as AI has today: the dawn of the world wide web.
Many a dyed-in-the-wool HPC nerd wrung his hands at clustering, claiming it wasn’t “real” HPC. It was capacity, not capability, people said. (The IDC HPC analyst team even built “Capacity HPC” and “Capability HPC” into its market methodology; this nomenclature persisted for years.) People complained that clustering wouldn’t suit bandwidth-constrained applications, that it would lead to low system utilization, and that it wouldn’t be worth the porting efforts. These are very similar to the arguments with respect to GPUs and lower precisions today.
Clusters won out, of course, though the transition took a decade, give or take. Clusters were industry-standard and cost-efficient. And once applications were taken through the (often times painful) process of porting to MPI, they could be migrated easily between different vendors’ hardware. Like it or not, low-precision GPUs could easily become the present-day analog. It becomes the task of the HPC engineer not to design enterprise technologies, but rather to utilize the technologies at hand.
Some segments of HPC will face a bigger threat, or opportunity, depending on your perspective. If AI really does become as good at predicting outcomes as traditional simulations, there will be areas in which the AI approach really does displace deterministic computing.
Take a classic HPC case like finite element analysis, used for crash simulation. Virtual crash simulations are faster and cheaper than physical tests. A car company can test more scenarios in less time, guiding development to optimal solutions. What if AI learned do it as well, or better? Would we still run the deterministic application? After all, the virtual model was never a perfect representation of the physical car.
There are (hopefully) limits to how far this displacement can go. HPC is (or should be) a long-term market because we do not reach the end of science, and as long as there is science to be done, and problems to be solved, there is a role for HPC in solving it. AI is still a black box that does not show its work. Science is a peer-reviewed process that relies on creative thinking. And at some point, scientists need to do the math. But across the full range of HPC applications, it is worth considering where we must rely on precise calculations and where a very good guess is good enough.
There are still promising HPC-focused technologies on the horizon. NextSilicon, for example, is bucking the trend by focusing on 64-bit computing for HPC applications. Driven by the need for non-American CPUs, both the EU and China are investing in the development of high-performance solutions based on RISC-V architecture. And perhaps most exciting, there have been major recent developments in quantum computing from multiple vendors across the industry.
In many ways, 2025 is shaping up to be an inflection point that will set the course for HPC-AI, not only for the rest of this decade, but for the next decade beyond that. At Intersect360 Research, our research calendar for the year will be tailored to get clarity on these critical industry dynamics. HPC-AI users globally can help steer the conversation by joining HALO. We’re listening. We’ve got some big questions to answer.
This article first appeared on sister site HPCwire.