Cracking Biology’s Code with Evo 2, an AI Trained on 100K Species
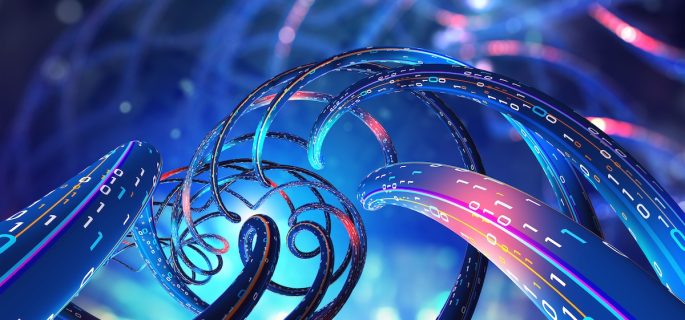
A new foundation model designed to unlock deeper insights into biological code has been released today. Developed through a collaboration led by Arc Institute and Nvidia, Evo 2 is trained on the DNA of more than 100,000 species, covering a vast range of life forms across different domains of biology.
Developers of Evo 2 say it can identify patterns in gene sequences across disparate organisms that experimental researchers would need years to uncover. It can also accurately identify disease-causing mutations in human genes and design new genomes that are as long as the genomes of simple bacteria.
Evo 2 was created by scientists from Nvidia and Arc Institute, a nonprofit biomedical research organization based in Palo Alto that works with collaborators across Stanford University, UC Berkeley, and UC San Francisco. Details about Evo 2 will be posted as a preprint today, accompanied by a user-friendly interface called Evo Designer. The Evo 2 code is publicly accessible from Arc Institute's GitHub and is also integrated into Nvidia’s BioNeMo framework as part of the collaboration.
Beyond just building the model, the Evo 2 team is prioritizing transparency and interpretability. In collaboration with AI research lab Goodfire, Arc Institute developed a visualizer to reveal how the model identifies key biological patterns in genomic sequences. To further support open science, the researchers are also releasing Evo 2’s training data, code, and model weights, making it the largest fully open source AI model of its kind, its creators claim.
Evo 2’s predecessor was Evo 1, a model trained entirely on single-cell genomes. Evo 2 builds on this previous model, having been trained on over 9.3 trillion nucleotides—the building blocks that make up DNA or RNA—from over 128,000 whole genomes as well as metagenomic data, its developers say. In addition to an expanded collection of bacterial, archaeal, and phage genomes, Evo 2 includes information from humans, plants, and other single-celled and multi-cellular species in the eukaryotic domain of life.
“Our development of Evo 1 and Evo 2 represents a key moment in the emerging field of generative biology, as the models have enabled machines to read, write, and think in the language of nucleotides,” says Patrick Hsu, Arc Institute Co-Founder, Arc Core Investigator, an Assistant Professor of Bioengineering and Deb Faculty Fellow at UC Berkeley, and a co-senior author on the Evo 2 preprint.
Hsu says Evo 2 has a generalist understanding of the tree of life that is effective at tasks like predicting disease-causing mutations and designing potential code for artificial life.
Evo 2 detects and uses the encoded biological information present in patterns throughout DNA and RNA. “Just as the world has left its imprint on the language of the Internet used to train large language models, evolution has left its imprint on biological sequences,” says the preprint’s other co-senior author Brian Hie, an Assistant Professor of Chemical Engineering at Stanford University, the Dieter Schwarz Foundation Stanford Data Science Faculty Fellow, and Arc Institute Innovation Investigator in Residence. “These patterns, refined over millions of years, contain signals about how molecules work and interact.”
Powering Evo 2’s massive training effort required serious computational muscle, and Nvidia played a key role in making it happen. The model was trained over several months on the NVIDIA DGX Cloud AI platform via AWS, leveraging more than 2,000 NVIDIA H100 GPUs with support from Nvidia researchers and engineers."
Achieving Evo 2’s ability to process long genetic sequences, consisting of up to 1 million nucleotides at once, also required rethinking AI architecture. Greg Brockman, co-founder and president of OpenAI, spent part of a sabbatical tackling this challenge, helping develop a new system called StripedHyena 2 that dramatically expanded the model’s capacity, allowing it to be trained with 30 times more data than Evo 1 and reason over 8 times as many nucleotides at a time.
Evo 2 is already demonstrating its potential in biological research. The model has shown over 90% accuracy in identifying which mutations in the BRCA1 gene (the gene associated with breast cancer) are benign or potentially pathogenic—an ability that could streamline genetic research, reducing the need for costly and time-consuming experiments.
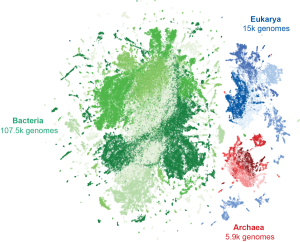
Evo 2 is trained on over 9.3 trillion tokens–in this case, nucleotides–from over 128 thousand genomes across the three domains of life (visualized here as points clustered by similarity), making it similar in scale to the most powerful generative AI large language models. (Source: Arc Institute)
Aside from genetic analysis, Evo 2 could also be a powerful tool for designing new biological therapies. Researchers could engineer gene therapies that activate only in specific cell types, for example, reducing side effects and improving precision.
“If you have a gene therapy that you want to turn on only in neurons to avoid side effects, or only in liver cells, you could design a genetic element that is only accessible in those specific cells,” explains co-author Hani Goodarzi, Arc Core Investigator and computational biologist at UCSF. “This precise control could help develop more targeted treatments with fewer side effects.”
The research team sees Evo 2 as a foundation for even more specialized AI models in biology. “In a loose way, you can think of the model almost like an operating system kernel—you can have all of these different applications that are built on top of it,” says David Burke, Arc Institute CTO and co-author on the preprint. As the model is refined and applied in new ways, its full potential is still unfolding.
Recognizing the ethical considerations of large-scale biological AI, the researchers took precautions by excluding pathogens that infect humans and other complex organisms from Evo 2’s training data. Stanford’s Tina Hernandez-Boussard and her lab contributed to ensuring responsible development and deployment of the model.
“Evo 2 has fundamentally advanced our understanding of biological systems,” says Anthony Costa, director of digital biology at NVIDIA. “By overcoming previous limitations in the scale of biological foundation models with a unique architecture and the largest integrated dataset of its kind, Evo 2 generalizes across more known biology than any other model to date—and by releasing these capabilities broadly, the Arc Institute has given scientists around the world a new partner in solving humanity’s most pressing health and disease challenges.”