Graphing Biodiversity to Improve Drug Discovery
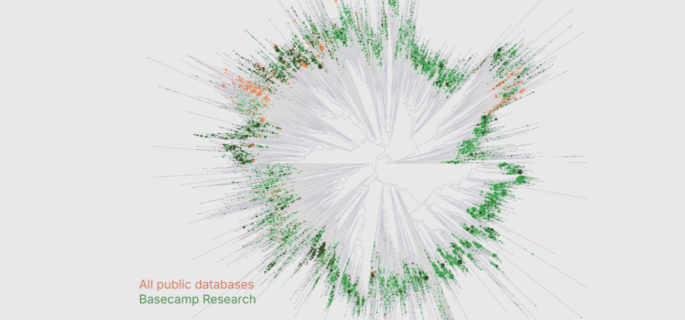
(Source: Basecamp Research)
Most pharmaceuticals are naturally occurring, either directly or indirectly. Yet when it comes to cataloging all of the proteins and enzymes that have evolved on Earth over the past 4 billion years, human knowledge barely scratches the surface. That’s why a company called Basecamp Research is bringing together graph and AI technologies to expand the scope of human knowledge and accelerate drug discovery.
Basecamp Research was founded in 2019 by Glen Gowers and Oliver Vince with the goal of accelerating data-driven breakthroughs in pharmaceutical research. The two biologists with PhDs from Oxford University were frustrated by the lack of progress in bringing field data into the lab to fuel drug discovery, so they decided to found a company to address it.
At the core of the private UK company’s endeavor is a knowledge graph that is designed to function as a digital twin of the natural world. Running on the Neo4j graph database, the BaseGraph contains 5.5 billion biological relationships and is the biggest such database in the world. The company says it has gathered 10x more data than all comparable public databases, and structured it to maximize the context, diversity, and biological signals within.
Neo4j is used by many pharmaceutical firms to do drug discovery, says Philip Rathle, the CTO at Neo4j. But what makes BaseGraph unique is that it also catalogs the environmental conditions in which they exist, such as temperature, humidity, soil chemistry, pH, mineral content of soils, etc., which is critical to achieving understanding of the enzymes, proteins, and full organisms.
“They are the only ones, to the best of my knowledge, to recognize that only a fractional percentage point, like 0.01%, of all life on Earth, has been cataloged in a way that can be used towards discovering new drugs,” Rathle says. “They’re taking the data in the ecosystem, putting it into a graph that connects it to the microbiology, and then their customers–companies doing drug development–use that information to develop better drugs, faster.”
Fielding Data
Environmental data is critical to fully understand how proteins and enzymes will behave in different environments and ultimately what value they can offer to pharmaceutical development.
For instance, if the pH in a lab setting is off by 1% relative to the natural setting, it can cause proteins to behave in an entirely different manner, Rathle says. The existence of iron, for example, can make the difference between a biological interaction happening and not happening at all.
To gather this data, Basecamp Research works with third-party scientists who go out into the field and collect this data. The data they collect comes from some of the most remote spots on the globe, places like the Amazon rainforest and the frozen deserts of Antarctica (the name of the company came from DNA sequencing fieldwork Goers and Vince did while living on an ice cap).
When Basecamp makes money off some of the data, the company has committed to returning a portion of the proceeds back to the national parks and other entities protecting the land. Ensuring the integrity of data from its field supply chain is critical, the company says, as is maintaining Earth’s wild places, where enzymes, proteins, and organisms live and evolve.
5.5 Billion Edges and Counting
BaseGraph contains three types of data, including: environmental, geological, and chemical data; microecology, metagenomics, and genomic context; and deep learning-derived functional and structural protein characteristics.
All of this data is loaded into BaseGraph, which at 5.5 billion biological relationships, is already the largest graph of biological data in the world. It’s expanding at the rate of 500 million new ones every four weeks, as new data comes in, the company says.
The decision to use a graph database came after some period of tech discovery for BaseCamp. “My first instinct was ‘stick it all in tables and JOIN it,’” said Saif Ur-Rehman, the data engineering team lead at Basecamp Research, according to a YouTube presentation published by Neo4j.
However, they quickly ran into the limits of standard database tech. “Life works as a network, not as a list,” Basecamp’s CTO Phil Lorenz said in a story on the Neo4j website.
After selecting Neo4j, which is one of the most heavily used and most well-established graph databases on the market, the Basecamp Research team set out to model their data. They used graph embeddings available through the Neo4j Graph Data Science (GDS) library to represent proteins “not just through their sequence alone, but incorporate essential contextual information that can show how these proteins will interact, behave, and ultimately perform,” Neo4j says in its write-up.
Base storing connected data in this way, Basecamp customers can query the graph and discover relationships that would otherwise stay hidden, what the company calls “microbial dark matter,” which refers to the vast space of unexplored microorganisms.
Enter AI
This is already paying dividends. According to Neo4j, researchers have discovered 30 times more Large Serine Recombinases (LSR) enzymes, which opens up the potential for creating novel therapies through gene editing.
Another success came from the chemical manufacturing industry, where a $16 billion company was able to leverage a Neo4j graph algorithm and BaseGraph to optimize a specific enzyme in just a month, recreating work that took two years previously
Basecamp Research is also using AI technology in combination with the graph database to drive even more discovery. It is training large language models (LLMs) with the known interactions established in the graph database, which allows it to generate potential candidates for drug development.
The company has published a paper on ZymCTRL, or enzyme control, a model trained on enzyme sequences that can generate active enzymes according to user needs. It has also published papers on BaseFold, a model for large complex protein structures, and Hierarchically Fine-tuned Nearest Neighbor method (HiFi-NN), a protein function model.
In the “GEN Biotechnology” journal, Vince, Gowers, and Siân McGibbon write that Basecamp Research has embarked upon a new model that enables the continued generation of data from the natural world that’s necessary for research without compromising on ethics.
“The advent of AI in biotechnology brings a watershed moment for the industry,” they write. “Limited availability of high-quality training data is already slowing the pace of innovation. The nascent big data era in biotechnology presents a natural opportunity to align commercial interests, development goals, and sustainability objectives of stakeholders in the bioeconomy. The growing demand for vast quantities of high-quality genetic data for training large models can only be met by developing sustainable partnership-based data supply chains which actively align incentives and share benefits with the providers of biodiversity.”
This article first appeared on sister site BigDATAwire.
Related
Alex Woodie has written about IT as a technology journalist for more than a decade. He brings extensive experience from the IBM midrange marketplace, including topics such as servers, ERP applications, programming, databases, security, high availability, storage, business intelligence, cloud, and mobile enablement. He resides in the San Diego area.